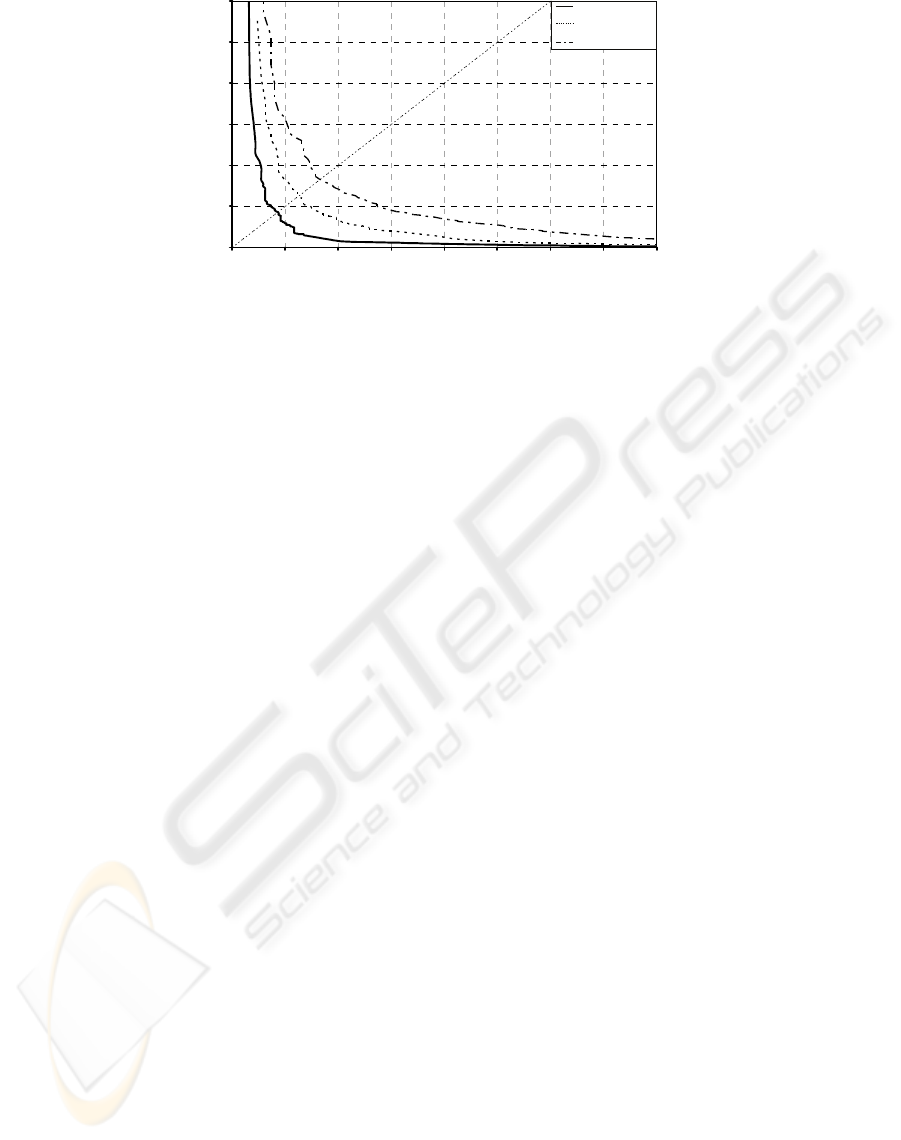
0
5
10
15
20
25
30
0 5 10 15 20 25 30 35 40
FRR
FAR
Combination Feature
Dependent Text Feature
Independet Text Feature
Figure 5: ROC curve of the verification experiment.
Currently, our better performances have been
combining independent and dependent text features.
The system performs very well on both tasks of
accept and reject. An Equal Error Rate (EER) of
about 3.90% is achieved.
In the future work, we plan to address at
improving the performance independent text feature.
We also plan to expand our database and test our
system with other databases such as the IAM
database.
ACKNOWLEDGMENTS
This present work has been supported by private
funds from Spanish Company, Telefónica. This
research project is called “Cátedra Teléfonica-
ULPGC 2009”; and by funds from Research Action
from Excellent Networks on Biomedicine and
Environment belong to ULPGC.
REFERENCES
Bishop, C.B., 1995. Neural Networks for Pattern
Recognition, Oxford University Press.
Juang, B.H., Rabiner, L.R., 1992. Spectral representations
for speech recognition by neural networks-a tutorial.
In Proceedings of the Workshop Neural Networks for
Signal Processing, pp. 214 – 222.
Romero, C.F., Travieso, C. M., Alonso, J. B., Ferrer, M.
A., 2007. Using Off-line Handwritten Text for Writer
Identification. In WSEAS Transactions on Signal
Processing. Issue 1, Vol. 3, pp. 56-61.
Cha, S. H., Srihari, S., 2000. Multiple feature integration
for writer verification. In Proceedings Seventh
Inernational. Workshop on Frontiers in Handwriting
Recognition, pages 333-342.
Leedham, G., Chachra, S., 2003. Writer Identification
using Innovate Binarised Features of Handwritten
Numerals. In Proceeding of the 7th International
Conference on Document Analysis and Recognition.
Vol. 1, pp. 413-416.
Schlapbach, A., Bunke, H., 2004. Using HMM Based
Recognizers for Writer Identification and Verification.
In Proceeding of the 9th Int’l Workshop on Frontiers
in Handwriting Recognition
Bensefia, A., Paquet, T., Heutte, L., 2005. Handwritten
Document Analysis for Automatic Writer
Recognition. Electronic Letter on Computer Vision
and Image Analysis, pp. 72-86.
Bucalu, M., Shomaker, L., 2007. Text-Independent Writer
Identification and Verification Using Textual and
Allographic Features. In IEEE Transactions on
Pattern Analysis and Machine Intelligence, Vol. 29,
No. 4.
Otsu, N., 1979. A threshold selection method from
graylevel histograms. In IEEE Transaction on
Systems, Man and Cybernetics, Vol. 9, Is. 1, pp 62-66.
Jaekyu Ha, R. M., Haralick, I. T., 1995. Document page
decomposition by the bounding-box project, In
Proceedings International Conference on Document
Analysis and Recognition, Vol. 02, No. 2, p. 1119.
Romero, C.F., Travieso, C. M., Alonso, J. B., Ferrer, M.
A., 2009. Hybrid Parameterization System for Writer
Identification. In Second International Conference on
Bio-inspired Systems and Signal Processing, pp. 449-
454.
Hertel, C., Bunke, H., 2003. A Set of Novel Features for
Writer Identification. In Proceedings of the Audio and
Video Based Biometric Person Authentication. pp.
679-687.
13. Srihari, S., Cha, S.H., Arora, H., Lee, S., 2001.
Individuality of Handwriting: A Validity Study. In
Proceedings of International Conference on Document
Analysis and Recognition pp 106-109.
14. Morita, M.E. Facon, J. Bortolozzi, F. Garnes,
S.J.A. Sabourin, R., 1999. Mathematical Morphology
and Weighted Least Squares to Correcthandwriting
Baseline Skew. In Proccedings of the 5th
International Conference on Document Analysis and
Recognition, pp. 430-433.
BIOSIGNALS 2010 - International Conference on Bio-inspired Systems and Signal Processing
358