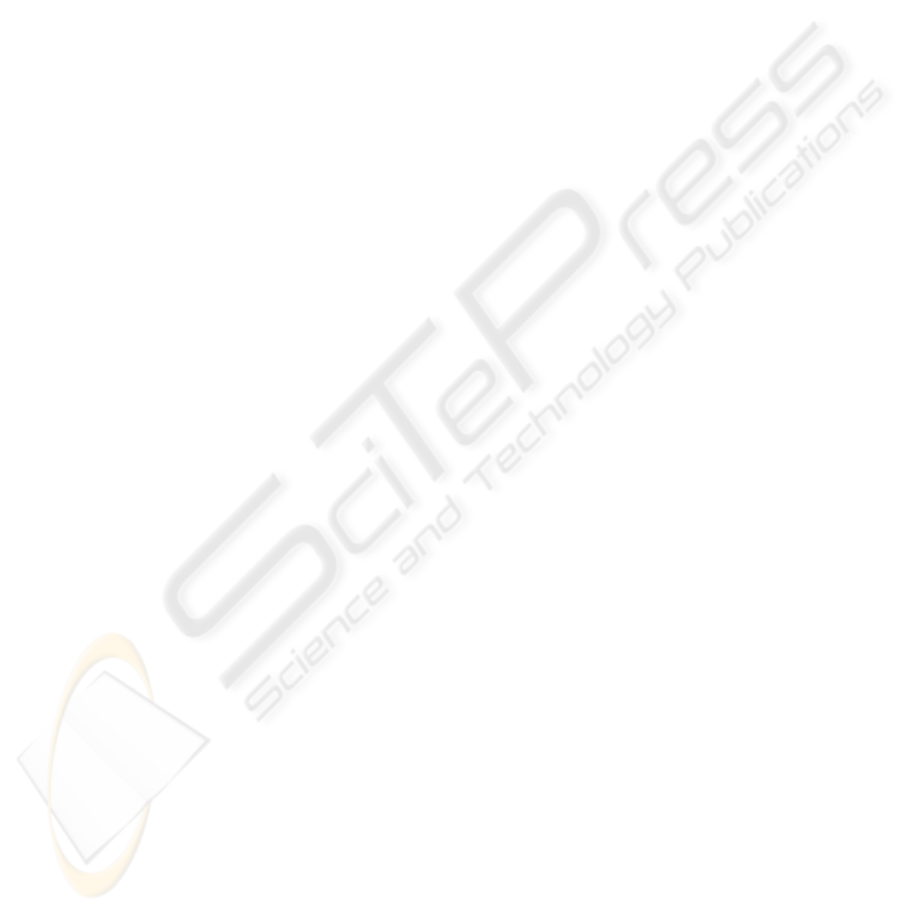
able to make the correct classification thanks to the
merging of information arising from both bands. In
the case of the Drinnan et al. algorithm, the patient
must present altered activity in the only band the al-
gorithm considers –LF.
The fact that neither the Drinnan et al. algorithm
nor ours classified any of the control patients as hav-
ing OSA suggests that both algorithms have a rather
high specificity. This specificity may have its root in
the fact that healthy patients do not usually show al-
tered HRV activity in any of the two bands, although
some OSA patients do not present the typical altered
activity, despite their disorder.
The scientific literature states that patients suffer-
ing from OSA have decreased activity in the LF band
and increased activity in the HF band. However, the
best classification bands found by our scripts for the
Apnea-ECG Database recordings differ from the tra-
ditional LF and HF bands. Our high band –between
0.06 and 0.25 Hz– incorporates a fragment of the LF
band (between 0.06 and 0.014), and our low band –
between 0.026 and 0.06 Hz– includes only the lower
part of the LF band and the VLF band (Very Low Fre-
quency, between 0.002 and 0.004 Hz).
However, the algorithm is based on patients suf-
fering from OSA having less power in the high band
and more power in the low band, because it calculates
the ratio between the low band and the high band, and
expects that OSA patients have a higher value for this
ratio than healthy patients. Furthermore, in our at-
tempt to find bands as close as possible to the LF and
HF bands, the classification results are significantly
worse than those obtained with the optimal bands –
6 recordings misclassified in the training set, and 8
in the testing test. Therefore, our results suggest that
OSA patients have increased activity only in the lower
part of the LF band and in the VLF band, and have de-
creased activity not only in part of the HF band, but
also in the 0.06 and 0.014 Hz range of the LF band.
5 CONCLUSIONS
This paper presents a simple but effective algorithm
capable of classifying patients as OSA or non-OSA
on the basis of a single ECG lead. The algorithm cal-
culates the power spectrum of the time series obtained
by filtering and resampling at 4 Hz the RR intervals
extracted from the ECG lead. Then the power of the
bands between 0.026 and 0.06 Hz and between 0.06
and 0.25 Hz is calculated, and then the ratio between
the power of the low band and the high band is ob-
tained. If this ratio is greater than a certain threshold
–0.43– the patient is classified as OSA. The bound-
aries of the bands and the threshold were obtained
by means of a semi-automatic training stage where
the Apnea-ECG Database training data set was used.
The algorithm was validated on the test data set of
the same database, incorrectly classifying only 1 of
30 control and OSA recordings.
Our future work aims to obtain a screening test
for OSA patients that can be performed at low cost
and in the patient’s home. This can avoid travels to
the hospital, long waiting lists, and other inconve-
niences for the patient. To this end, the test will be
based on MEDIM, a PDA platform with the capabil-
ity of recording ECGs (Presedo et al., 2009). The
algorithm we have developed has a low demand of
computational resources, which makes it suitable to
be implemented in such a device.
ACKNOWLEDGEMENTS
This work was supported by the Spanish MEC and the
European FEDER under the grant TIN2006-15460-
C04-02.
REFERENCES
Drinnan, M., Allen, J., Langley, P., and Murray, A. (2000).
Detection of sleep apnoea from frequency analysis
of heart ratevariability. In Computers in Cardiology
2000, pages 259–262.
K
¨
oves, P. (1999). Obstructive Sleep Apnea Syndrome.
Springer Verlag.
Narkiewicz, K., Montano, N., Cogliati, C., van de Borne,
P., Dyken, M., and Somers, V. (1998). Altered cardio-
vascular variability in obstructive sleep apnea. Circu-
lation, 98:1071–1077.
Penzel, T., McNames, J., de Chazal, R., Raymond, B., Mur-
ray, A., and Moody, G. (2002). Systematic compari-
son of different algorithms for apnea detection based
on electrocardiogram recordings. Med. Biol. Eng.
Comput, 40:402–407.
Penzel, T., Moody, G., Mark, R., Goldberger, A., and Peter,
J. (2000). The apnea-ecg database. In Computers in
Cardiology 2000, volume 27, pages 255–258.
Presedo, J., Tarasc
´
o, M., Teijeiro, T., F
´
elix, P., Castro, D.
(2009). A PDA-based modular and multipurpose sys-
tem for intelligent ubiquitous monitoring. In WISP
2009, pages 223–228.
Vila, J., Palacios, F., Rodr
´
ıguez-Presedo, J., Fernandez-
Delgado, M., F
´
elix, P., and Barro, S. (1997). Time-
frequency analisys of herat-rate variability. IEEE Eng.
in medicine and biology magazine, 16:119125.
BIOSIGNALS 2010 - International Conference on Bio-inspired Systems and Signal Processing
362