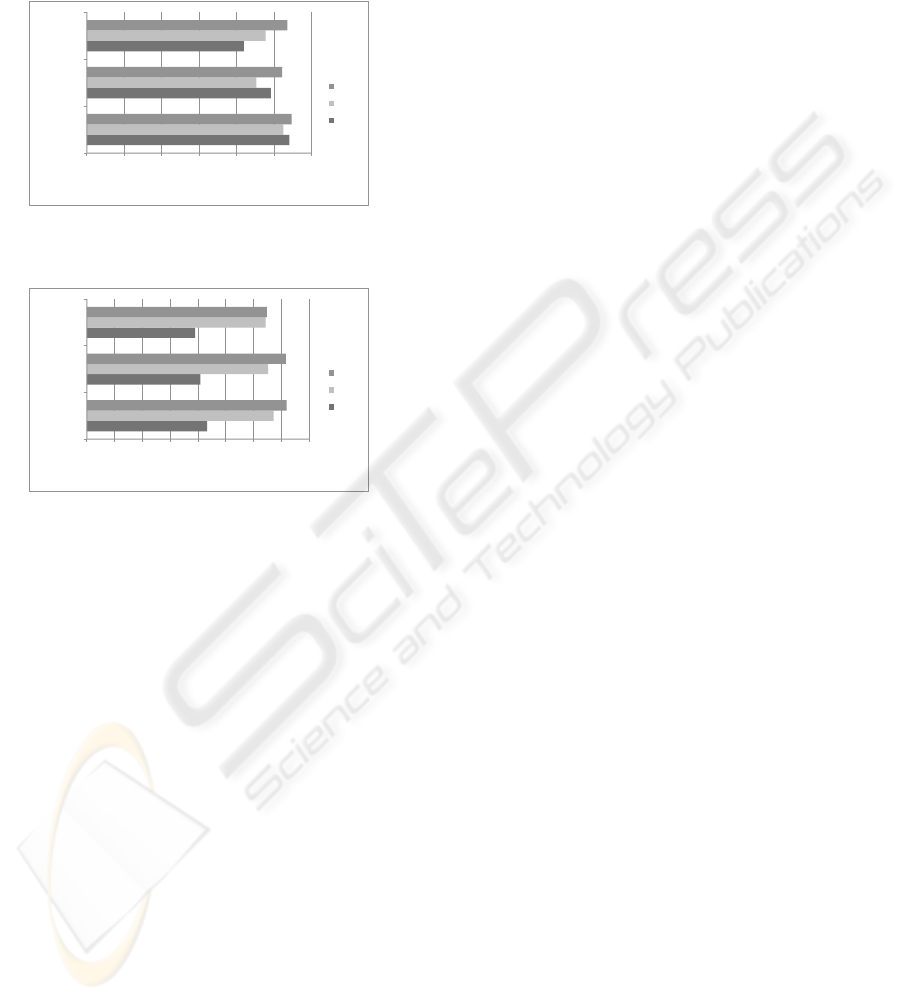
noise, 65.18% for 15 dB of SNR, 64.32% for 5 dB of
SNR) and ICA (43.23% for no noise, 40.77% for 15
dB of SNR, 38.94% for 5 dB of SNR). The results of
f
p
and SC in the second scenario are depicted in Fig
7 and Fig 8, respectively.
Figure 7: Mean f
p
of the AA extracted by ICA, WBS and
CMBS from real ECG recordings at three levels of SNR.
0% 10% 20% 30% 40% 50% 60% 70% 80%
Figure 8: Mean SC of the AA extracted by ICA, WBS and
CMBS from real ECG recordings at three levels of SNR.
6 CONCLUSIONS
Our analysis showed up that CMBS improves the ex-
traction performance of WBS and ICA in both sce-
narios so that a high accuracy of the estimated AA
for synthetic and real AF ECG episodes is accom-
plished, what is proved by the high values of R
t
. In
addition, the high values of R
s
and SC and the low
error of f
p
estimation prove that the original spectral
parameters of the AA are preserved in the AA esti-
mated by CMBS from both synthetic and real signals.
This fact enables CMBS as a suitable previous step to
the analysis of AA signals in the spectral domain.
ACKNOWLEDGEMENTS
This work was supported by the projects TEC2007–
64884 from the Spanish Ministry of Science and
Innovation and PAID–05–08 from the Universidad
Polit
´
ecnica de Valencia.
REFERENCES
Addison, P. (2001). The Illustrated Wavelet Transform
Handbook.1st edition. Taylor & Francis.
Amari, S., Cichocki, A., and Yang, H. (1996). A new learn-
ing algorithm for blind source separation. Advances in
Neural Information Processing Systems, 8:757–763.
Asano, F., Ikeda, S., Ogawa, M., Asoh, H., and Kitawaki, N.
(2001). A combined approach of array processing and
independent component analysis for blind separation
of acoustic signals. In Proceedings of the IEEE Con-
ference on Acoustics, Speech and Signal Processing,
Salt Lake City, USA.
Bollmann, A., Husser, D., Mainardi, L., Lombardi, F., Lan-
gley, P., Murray, A., Rieta, J. J., Millet, J., Olsson,
S. B., Stridh, M., and L, S. (2006). Analysis of sur-
face electrocardiograms in atrial fibrillation: Tech-
niques, research and clinical applications. Europace,
8(11):911–926.
Bollmann, A., Sonne, K., Esperer, H. D., Toepffer, I., Lang-
berg, J. J., and Klein, H. U. (1999). Non-invasive as-
sessment of fibrillatory activity in patients with parox-
ysmal and persistent atrial fibrillation using the Holter
ECG. Cardiovascular Research, 44:60–66.
Castells, F., Rieta, J. J., Millet, J., and Zarzoso, V.
(2005). Spatiotemporal blind source separation ap-
proach to atrial activity estimation in atrial tach-
yarrhythmias. IEEE Transactions on Biomedical En-
gineering, 52(2):258–267.
Hyv
¨
arinen, A., Karhunen, J., and Oja, E. (2001). Indepen-
dent Component Analysis. John Wiley & Sons, New
York.
Langley, P., , J. J., Stridh, M., Millet, J., Srnmo, L., and
Murray, A. (2006). Comparison of atrial signal ex-
traction algorithms in 12-lead ECGs with atrial fibril-
lation. IEEE Transactions on Biomedical Engineer-
ing, 53(2):343–346.
Rieta, J. J., Castells, F., S
´
anchez, C., and Zarzoso, V. (2004).
Atrial activity extraction for atrial fibrillation analysis
using blind source separation. IEEE Transactions on
Biomedical Engineering, 51(7):1176–1186.
Slocum, J., Sahakian, A., and Swiryn, S. (1992). Diagno-
sis of atrial fibrillation from surface electrocardiogram
based on computer-detected atrial activity. Journal of
Electrocardiology, 25(1):1–8.
Snchez, C., Rieta, J., Castells, F., Alcaraz, R., and Millet,
J. (2004). Wavelet blind separation: A new method-
ology for the analysis of atrial fibrillation from holter
recordings. In Proc.Int.Conf on Computers in Cardi-
ology, volume 31, pages 417–420.
Vay, C., Rieta, J. J., Snchez, C., and Moratal, D. (2007).
Convolutive blind source separation algorithms ap-
plied to the electrocardiogram of atrial fibrillation:
Study of performance. IEEE Transactions on Biomed-
ical Engineering, 54(8):1530–1533.
BIOSIGNALS 2010 - International Conference on Bio-inspired Systems and Signal Processing
376