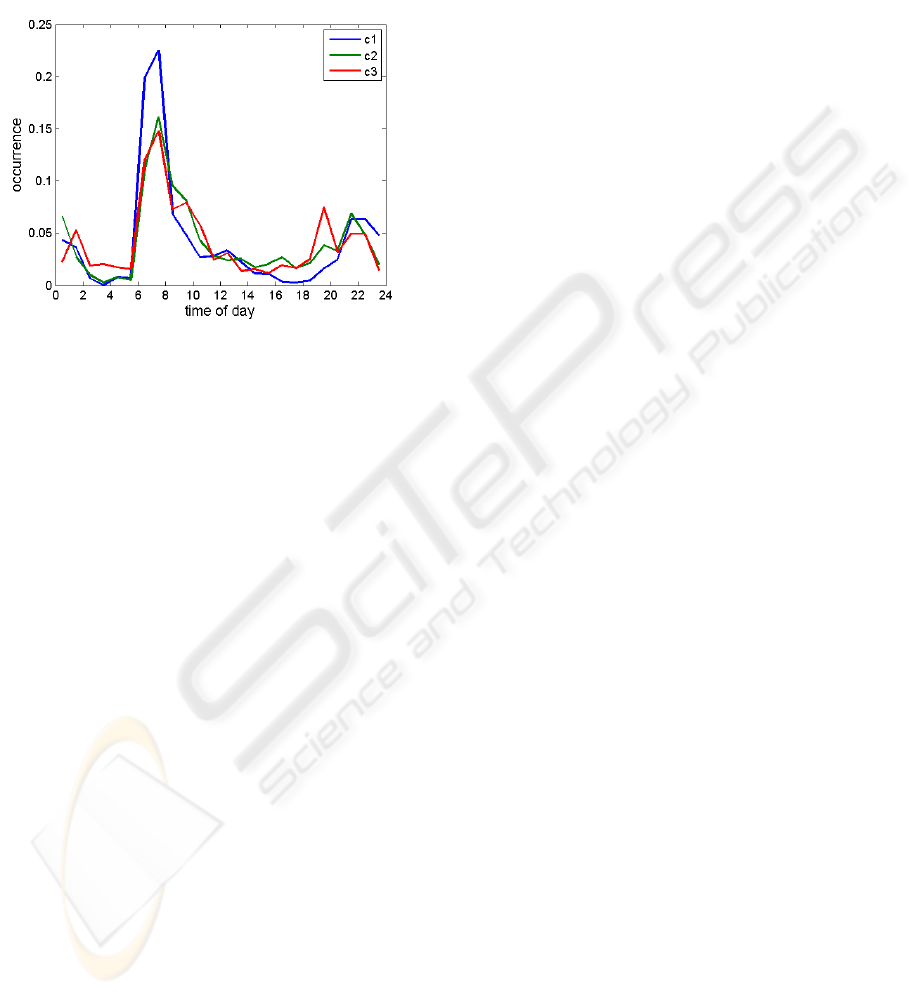
brushing is usually accompanied by other activities in
the bathroom that are likely to trigger a false logging
event. There is a pronounced maximum between 7
and 8pm, which might be caused by a regular rou-
tine (e.g. washing after work), but this can only be
guessed due to the lack of further information.
Figure 5: The normalised distribution of logged events over
the day for the three groups C1, C2, and C3.
4 CONCLUSIONS
The present paper discusses the characterisation of
toothbrushing activity, where the data consists of ac-
celeration traces logged by a sensor that is integrated
in the brush. Because the data has been collected
under free-living conditions for a large population, it
was not possible to obtain an exhaustive training-set
of all possible super-threshold activities that trigger
the logging. Thus, a structural analysis of appropri-
ate data features is performed, which reveals a parti-
tion into three clusters. The comparison with brush-
ing activity traces from laboratory experiments allows
assigning one cluster to toothbrushing activity, while
the remainder corresponds to various false activation
events like electronic noise or handling of the brush
in the context of other activities.
An inherent property of real-life activity data is
the enormous variability, both within and across sub-
jects. Here, this is reflected by an overlap between the
clusters found in the data, that leads to a 13% classi-
fication uncertainty for toothbrushing events. A pos-
sible remedy for this problem is to collect more vari-
ables (e.g., rotation rate) at higher sampling frequen-
cies, which, however, is restricted by memory and en-
ergy consumption issues in the logger design. An im-
portant point is that the logger should not influence
the normal behaviour of the subjects while being in
the field for several days.
It would be interesting to gather more information
about the possible sources of false activation and their
acceleration patterns. This will require elaborate lab-
oratory experiments that take into account learnings
from field experiments.
REFERENCES
Bao, L. and Intille, S. (2004). Activity Recognition from
User-Annotated Acceleration Data, Lecture Notes in
Computer Science, volume 3001. Springer, Berlin.
Claessen, J. P., Bates, S., Sherlock, K., Seeparsand, F., and
Wright, R. (2008a). Designing interventions to im-
prove tooth brushing. International Dental Journal,
58.
Claessen, J. P., Seeparsand, F., and Wright, R. (2008b).
Brushing up on behaviour measurement: Validation
study of new technology. PEF-IADR, London.
Preece, S. J., Goulermas, J. Y., Kenney, L. P. J., and
Howard, D. (2009). A comparison of feature extrac-
tion methods for the classification of dynamic activ-
ities from acceleration data. IEEE Transactions on
Biomedical Engineering, 56:871.
Press, W. H., Teukolsky, S. A., Vetterling, W. T., and Flan-
nery, B. P. (2007). Numerical Recipies 3rd Edition:
The Art of Scientific Computing. Cambridge Univer-
sity Press, 3rd edition.
Van Someren, E. J. W., Lazerona, R. H. C., Vonk, B. F. M.,
Mirmirana, M., and Swaab, D. F. (1996). Gravita-
tional artefact in frequency spectra of movement ac-
celeration: implications for actigraphy in young and
elderly subjects. J Neurosci Methods, 65(1):55–62.
Vega-Gonzalez, A., Bain, B. J., Dall, P. M., and Granat,
M. H. (2007). Continuous monitoring of upper-limb
activity in a free-living environment: a validation
study. Medical and Biological Engineering and Com-
puting, 45(10):947–956.
Welk, G. W., McClain, J. J., Eisenmann, J. C., and Wickel,
E. E. (2007). Field validation of the mti actigraph and
bodymedia armband monitor using the ideea monitor.
Obesity, 15(4):918–928.
Zhu, L., Petersen, P. E., and Wang, H. Y. (2005). Oral health
knowledge, attitudes and behaviour of adults in china.
Int Dent J, 55:231–241.
BIOSIGNALS 2010 - International Conference on Bio-inspired Systems and Signal Processing
380