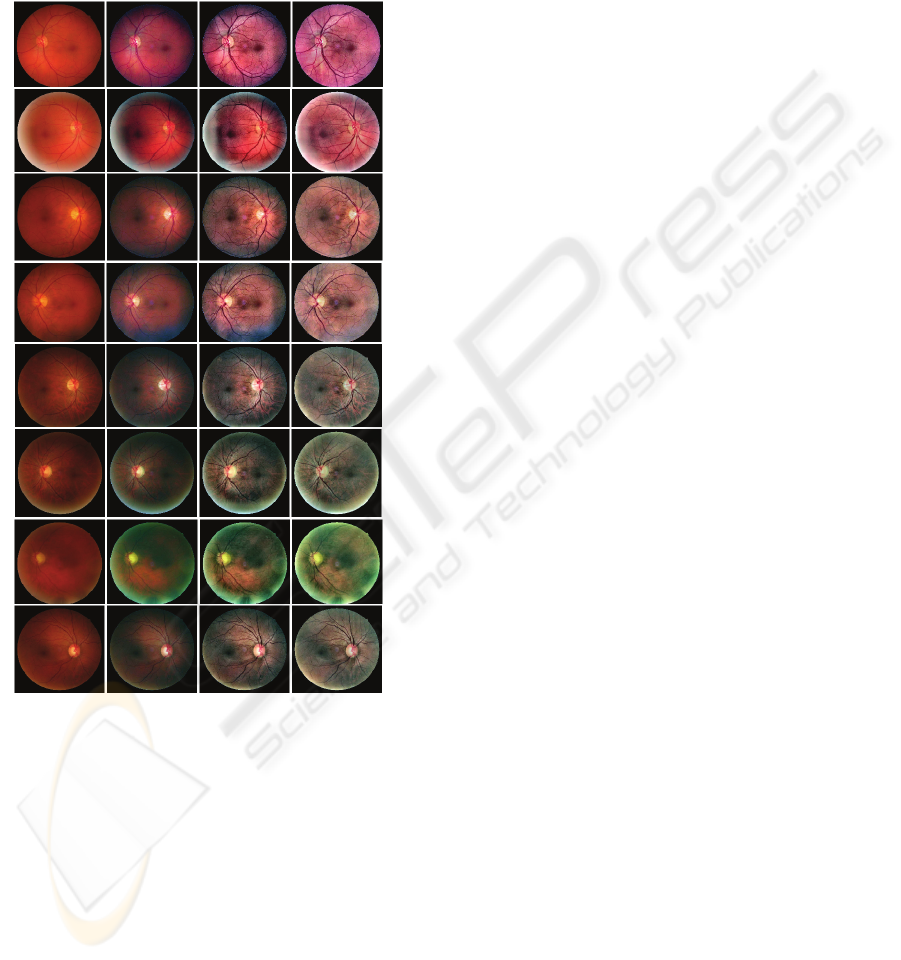
and they normally require the user intervention. The
work presented in this paper is part of a research
project developed in collaboration with the Hospital
Universitario de Canarias. The main objective of the
project is the design of an automated software
system for the delimitation of the ONH and for the
differentiation of superimposed structures (arteries,
vessels, and so on).
Figure 4: Experimental results. From left to right: original
image, image after step 1, image after step 2, image after
step 3.
Due to the difficulties presented by the
retinographies it is essential to carry out a
preprocessing stage, in order to improve the image
before developing segmentation of the different
regions of interest. This enhancement process has
been the core of this paper.
The proposed preprocessing method comprises
three steps: 1) scaling of the RGB histogram
(improvement of the image information), 2)
luminance equalization in YIQ color space (contrast
enhancement without loss of color information), and
3) illumination correction. As shown in the
experimental results, the preprocessing method
develops an important enhancement of the main
structures contained in the image. This will
considerably allow designing efficient segmentation
algorithms.
Our future research work will be oriented to the
design of automated and efficient segmentation
methods to be applied on the preprocessed images
obtained in this work.
ACKNOWLEDGEMENTS
This research work has been partially financiated by
the project ULLAPD-08/01 of the Agencia Canaria
de Investigación, Innovación y Sociedad de la
Información.
REFERENCES
Chaudhuri, S., Chatterjee, S., Katz, N., Nelson, M.,
Goldbaum, M., 1989. Detection of blood vessels in
retinal images using two-dimensionalmatched filters.
IEEE Trans. Med. Imag; vol. 8(3), pp.263-269.
Cox, J., Wood I., 1991. Computer-assisted optic nerve
head assessment. Ophthal. Physiol. Opt.; 11, pp.27-35.
Echevarria, P., Miller, T., O'Meara, J., 2004. Blood Vessel
Segmentation in Retinal Images. Project P14: Blood
Vessel Segmentation in Retinal Images.
http://robots.stanford.edu/cs223b04/project14.html.
Fang, B., Hsu, W., Lee, M.L., 2003. On the Detection of
Retinal Vessels in Fundus Images. Singapore-MIT
Alliance National University of Singapore. Computer
Science (CS).
Himaga, M., Usher, D., Boyce, J.F., 2002. Retinal Blood
Vessel Extraction by using Multi-resolution Matched
Filtering and Directional Region Growing
Segmentation. IAPR Workshop on Machine Vision
Applications.
Iqbal, M.I., Aibinu, A.M., Gubbal, N.S., Khan, A., 2006.
Automatic Diagnosis of Diabetic Retinopathy using
Fundus Images” University essay from Blekinge
Tekniska Högskola/Sektionen för Teknik (TEK).
Tesis.
Teng, T., Lefley, M., Claremont, D., 2002. Progress
towards automated diabetic ocular screening: a review
of image analysis and intelligent systems for diabetic
retinopathy. Med. Biol. Eng. Comput.; 40(1:2-13.
Young, I.Y., Gerbrands, J.J., Van Vliet, L.J., 1998. Image
Processing Fundamentals, in The Digital Signal
Processing Handbook, V. K. Madisetti and D. B.
Williams, Eds. Boca Raton, Florida: CRC Press in
cooperation with IEEE Press.
BIOSIGNALS 2010 - International Conference on Bio-inspired Systems and Signal Processing
384