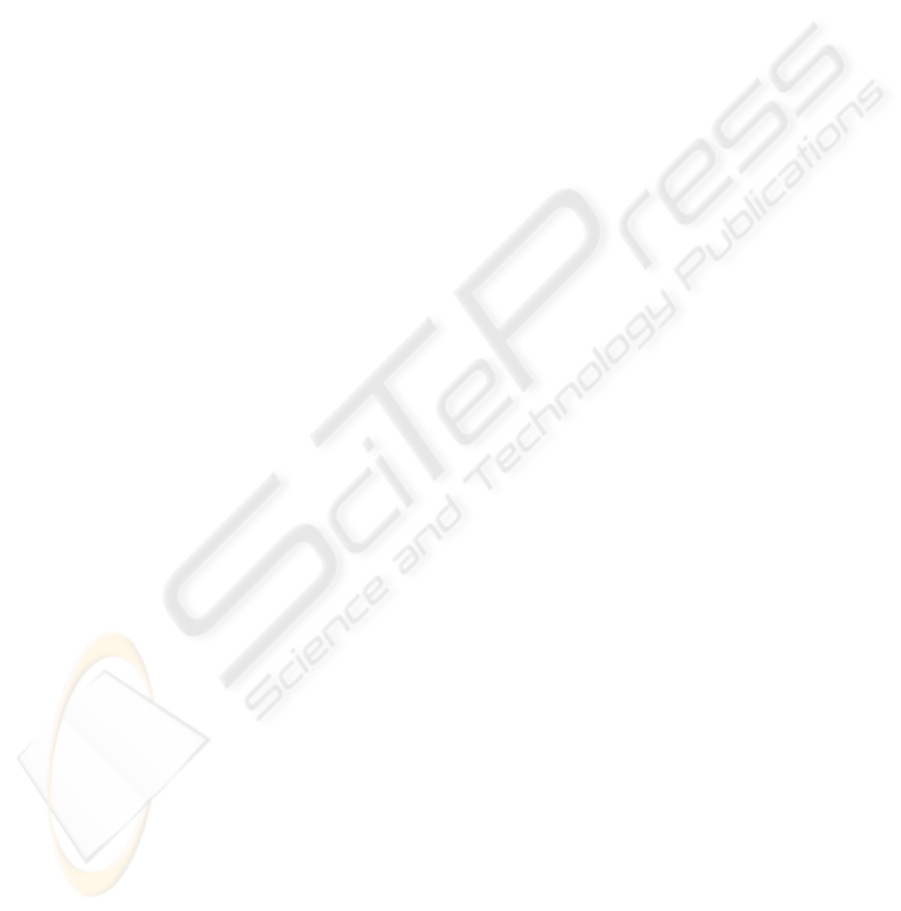
improve their computation times, and probably to in-
crease results quality due to the denoising effect of
STPCA.
5 CONCLUSIONS
This paper presents the adaptation of the Space
Time Principal Component Analysis (STPCA), a bi-
dimensional innovative reduction method, to images
data sets. Unlike to the other STPCA applications
proposed in the literacy that essentially exploit sum-
marizing abilities of the method, our approach fo-
cuses more on its dimension reduction capacities pro-
vided by the STPCA descriptor.
Numerical experiments on the Mnist data set contain-
ing 70000 handwritten digit pictures validate accu-
racy of the proposed approach to both strongly reduce
dimension and to conserve relations between images.
Denoising effect when reducing images dimension
even allows more accurate and intelligible classifica-
tion with low-dimension images descriptors than with
original pictures. Identification of represented digits
on Mnist test set images after a learning process on
the Mnist training set also demonstrates a very good
behaviour of the introduced approach. Such an identi-
fication process leads to a weaker test error rate when
it is performed from the 5 × 5 reduced-order matri-
ces computed for each image than those obtained with
original images of size 28 × 28. Using the proposed
approach, we thus obtain results at least as accurate
and intelligible from reduced data than from original
full-size ones with weaker computation times.
Future works concern the use of reduced images as in-
put data in more complex digit learning systems like
support vector machines or large convolutional nets,
that could improve both their computability and accu-
racy, as much as its combination to non-linear fea-
ture extractors such as LLE or Isomap. Moreover,
it could also be interesting to integrate proposed ap-
proach to complex databases or datawarehouses man-
agement systems.
REFERENCES
Bauzer-Medeiros, C., Joliveau, M., Jomier, G., and De-
Vuyst, F. (2008). Managing sensor data on urban traf-
fic. Advances in Conceptual Modeling – Challenges
and Opportunities, 5232/2008:385–394.
Bauzer-Medeiros, C., Joliveau, M., Jomier, G., and De-
Vuyst, F. (2009). Managing sensor traffic data and
forecasting unusual behaviour propagation. Geoinfor-
matica.
Joliveau, M. (2008). Reduction of urban traffic time series
from georeferenced sensors, and extraction of spatio-
temporal series. PhD thesis, Ecole Centrale Des Arts
Et Manufactures (Ecole Centrale Paris).
Joliveau, M. and DeVuyst, F. (2007). Space-time sum-
marization of multisensor time series. case of miss-
ing data. In Proc. of 2007 International Workshop
on Spatial and Spatio-Temporal Data Mining, pages
631–636.
Joliveau, M. and DeVuyst, F. (2008). Recherche de mo-
tifs spatio-temporels de cas atypiques pour le trafic
routier urbain. Extraction et Gestion de Connais-
sances EGC 08, Revue des Nouvelles Technologies de
l’Information - RNTI - E11, F. Guillet et B. Trousse,
2:523–534.
Jolliffe, I. (1986). Principal component analysis. Springer-
Verlag, New York.
Keysers, D., Deselaers, T., Gollan, C., and Ney, H. (2007).
Deformation models for image recognition. IEEE
Transactions on Pattern Analysis and Machine Intel-
ligence, 29(8):1422–1435.
Kruskal, J. B. and Wish, M. (1978). Multidimensional Scal-
ing. Sage Publications.
Labusch, K., Barth, E., and Martinetz, T. (2008). Simple
method for high-performance digit recognition based
on sparse coding. IEEE transactions on neural net-
works, 19:1885–1889.
Lauer, F., Suen, C., and Bloch, G. (2007). A trainable fea-
ture extractor for handwritten digit recognition. Pat-
tern Recognition, 40:1816–1824.
LeCun, Y., Bottou, L., Bengio, Y., and Haffner, P. (1998).
Gradient-based learning applied to document recogni-
tion. In Proc. of the IEEE, volume 86, pages 2278–
2324.
Ranzato, M., Poultney, C., Chopra, S., and LeCun, Y.
(2006). Efficient learning of sparse representations
with an energy-based model. In Proc. of Advances
in Neural Information Processing System NIPS 2006.
Roweis, T. and Saul, L. (2000). Nonlinear dimensional-
ity reduction by locally linear embedding. Science,
290:2323–2326.
Tenenbaum, J., de Silva, V., and Langford, J. (2000). A
global geometric framework for nonlinear dimension-
ality reduction. Science, 290:2319–2323.
ICAART 2010 - 2nd International Conference on Agents and Artificial Intelligence
162