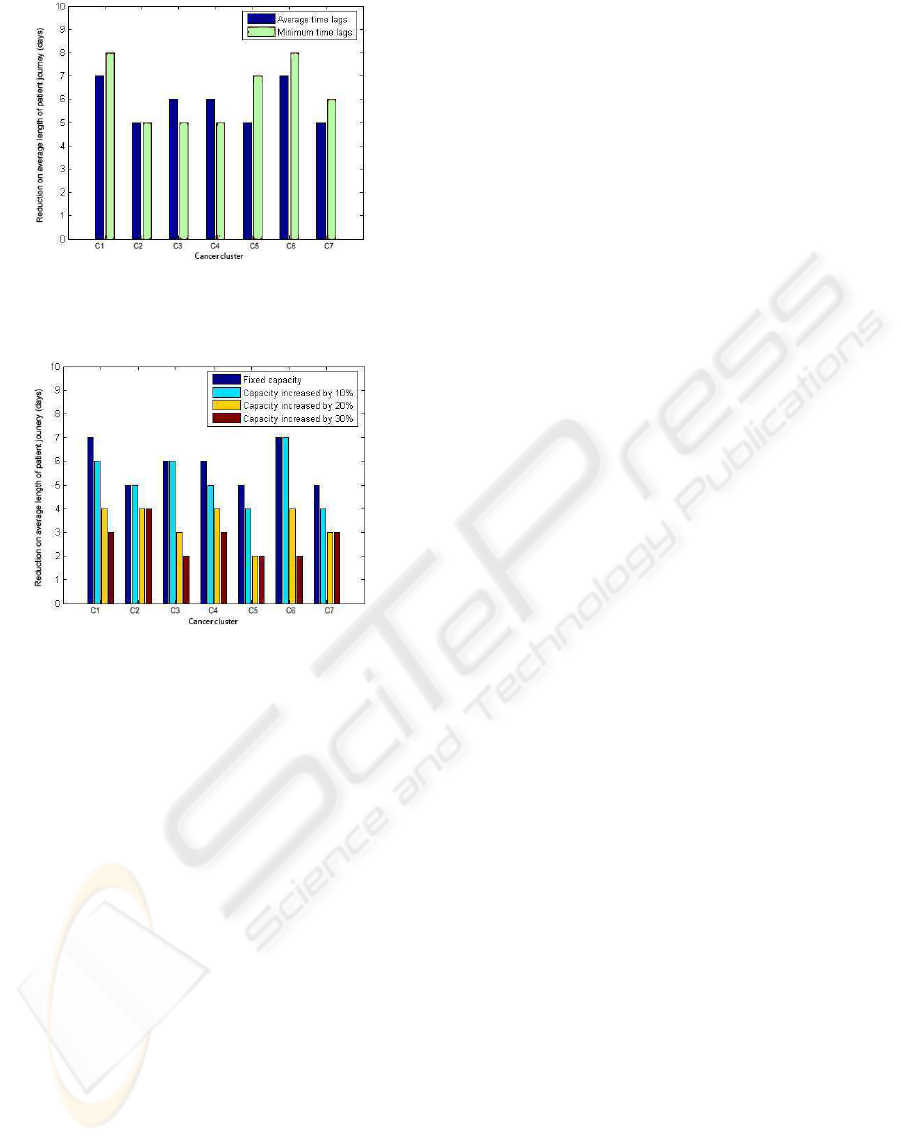
Figure 4: Reduction on average length of patient journey
(Setting 4) by varying the time lags between treatment op-
erations.
Figure 5: Reduction on average length of patient journey
(Setting 4) by varying capacities.
6 CONCLUSIONS
In this paper, a multi-agent approach was proposed
for patient journey optimization. Particularly, by ap-
plying the approach, the shortening of a patient jour-
ney will not lengthen the journeys of the others. Also,
all the temporal constraints among the treatment op-
erations for each patient would not be violated during
the scheduling process.
The effectiveness of the proposed approach has
been demonstrated by applying it to a dataset contain-
ing 5819 scheduled treatment plans of cancer patients
in Hong Kong. The effects of varying the initial as-
signment and the unit capacity on the overall reduc-
tion in length of patient journey are also studied.
Currently, since we are using a Pareto improve-
ment approach, it is assumed that no single patient
(agent) would get a lengthened schedule after swap-
ping timeslots with another. In the future, we are go-
ing to see whether there would be a greater improve-
ment in achieving a reduced average length of patient
journey when the above assumption for individuals is
relaxed.
ACKNOWLEDGEMENTS
This is to acknowledge Hospital Authority in Hong
Kong for providing the dataset to support this study.
REFERENCES
Czap, H. and Becker, M. (2003). Multi-agent systems
and microeconomic theory: A negotiation approach
to solve scheduling problems in high dynamic envi-
ronments. In HICSS ’03: Proceedings of the 36th An-
nual Hawaii International Conference on System Sci-
ences (HICSS’03) - Track 3, page 83.2, Washington,
DC, USA. IEEE Computer Society.
Decker, K., Li, J., and Demazeau, Y. (2000). Coordinating
mutually exclusive resources using gpgp. Autonomous
Agents and Multi-Agent Systems, 3:200–0.
Mao, X., Mors, A., Roos, N., and Witteveen, C. (2007).
Coordinating competitive agents in dynamic airport
resource scheduling. In MATES ’07: Proceedings
of the 5th German conference on Multiagent Sys-
tem Technologies, pages 133–144, Berlin, Heidelberg.
Springer-Verlag.
Patrick, J. and Puterman, M. (2008). Reducing wait times
through operations research: optimizing the use of
surge capacity. Healthc Q, 11(3):77–83.
Paulussen, T. O., Dept, I. S., Decker, K. S., Heinzl, A., and
Jennings, N. R. (2003). Distributed patient scheduling
in hospitals. In Coordination and Agent Technology
in Value Networks. GITO, pages 1224–1232. Morgan
Kaufmann.
Robu, V., Noot, H., La Poutr´e, H., and van Schijndel, W.-J.
(2008). An interactive platform for auction-based al-
location of loads in transportation logistics. In AAMAS
’08: Proceedings of the 7th international joint confer-
ence on Autonomous agents and multiagent systems,
pages 3–10, Richland, SC. International Foundation
for Autonomous Agents and Multiagent Systems.
Vermeulen, I., Bohte, S., Somefun, K., and La Poutre, H.
(2006). Improving patient activity schedules by multi-
agent pareto appointment exchanging. In CEC-EEE
’06: Proceedings of the The 8th IEEE International
Conference on E-Commerce Technology and The 3rd
IEEE International Conference on Enterprise Com-
puting, E-Commerce, and E-Services, page 9, Wash-
ington, DC, USA. IEEE Computer Society.
Vissers, J., Bekkers, J., and Adan, I. (2005). Patient mix op-
timization in tactical cardiothoracic surgery planning:
a case study. IMA Journal of Management Mathemat-
ics, 16.
Wang, M., Liu, J., Wang, H., Cheung, W. K., and Xie, X.
(2008). On-demand e-supply chain integration: A
multi-agent constraint-based approach. Expert Sys-
tems with Applications, 34(4):2683 – 2692.
HEALTHINF 2010 - International Conference on Health Informatics
186