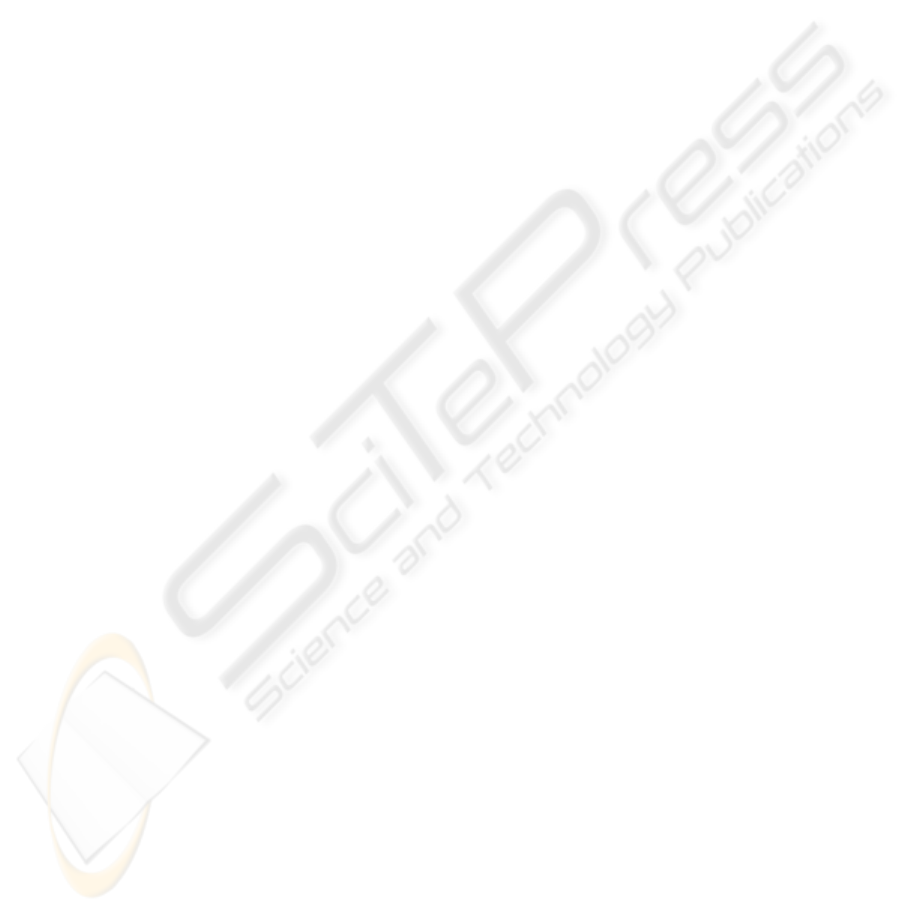
3.2 Classification
The method presented in this subsection is based
on the idea that classification performance between
glomerular activation and odorant features can give
information about this structure–activation relation-
ship. Specifically we take the classification per-
formance to compare relevances of properties to
glomerular coding. We performed classification us-
ing a linear support vector machine (SVM) from
glomerular activations as input vector and each prop-
erty (present vs. not present) as target. In each of 10
iterations we randomly sampled half of the activation
maps as training set and took the other half as test.
We distinguished between two experimental con-
ditions:
1. best points – classification using most representa-
tive points, and
2. random baseline – classification using randomly
sampled points.
For the first experimental condition, for each
property, we ranked points by their signifi-
cance with respect to the property (p–values
from Wilcoxon rank–sum test) and then clas-
sified taking the best n points, with n ∈ N =
[1, 5, 10, 15, 20, 25, 30, 45, 50, 60, 70, 80, 90, 100, 110,
120, 130, 140, 150, 200, 300, 400, 500, 600, 700, 800,
900, 1000, 1100, 1200, 1300, 1400, 1500, 1600, 1700,
1834]. As a random baseline, for each property,
we took the same intervals from N, but randomly
sampled points. We averaged over 250 random
subsamples of points for each interval.
The scarceness of data for some properties
brought about problems. We found that two com-
monly used SVM implementations, Svmlight and lib-
svm, are lacking robustness to tackle our problem.
Because of this we used an in–house SVM classifier
implemented in Matlab. We used the area under the
ROC curve (AUC) as performance criterion. It has
the advantage to be unbiased by skewed class distri-
butions, which are a particular problem in our data
set. An example of such an experimental run for the
aromatic property is shown in figure 3.
4 RESULTS
4.1 Localization of Coding Zones
Figure 2 shows loci of coding zones for the 13 molec-
ular properties.
In figure 2(a), colors indicate where significant
differences with respect to alkane were found. This
subfigure is to illustrate results from the statistical de-
termination of coding zones for a single property.
For the other chemical properties displayed in fig-
ure 2 we grouped properties into molecular bonds, cy-
clization, and functional groups. We created a fac-
torial code so that the color code accounts for all
combinations of coding for properties. For n prop-
erties, numbers from 0 to n− 1 were assigned to each
property. For each point, a binary vector expresses
whether a property was found to significant or not.
The ith position in this vector stands for property i.
Each vector represents a subset of all possible combi-
nations b
prop
∈ { 0, 1}
n
. Each subset was assigned its
distinct color.
Colors in figures in 2 show all combinations of
properties that were encountered. To give an exam-
ple, in 2(b) there are seven kinds of zones that mark
codes for different combinations of properties alkane,
alkene, and alkyne. Zones 1, 2, and 4 code for ex-
clusively one of these properties. Zones 3 encodes
alkane and alkene, zone 5 alkane and alkyne, zone 6
alkene and alkyne, and finally zone 7 codes for all of
the three properties.
Cyclization properties, especially alicyclic, have
a moderate but highly significant Pearson correlation
(ρ = 0.33, p = 2.05e− 9 between alicyclic and poly-
cyclic, ρ = 0.36, p = 6.72e−11 between alicyclic and
heterocyclic, and ρ = 0.21, p = 1.95e − 04 between
aromatic and heterocyclic). As can be seen in 2(c) and
2(d), properties aromatic and heterocyclic and prop-
erties alicyclic and polycyclic, respectively, project to
very similar bulbar regions. Functional groups did not
have a high covariance, however there are many prop-
erties (6). To provide clearer figures, we split coding
zones of both, cyclization and functional groups into
two figures.
4.1.1 Size of Coding Zones
Table 1 shows size of coding zones as estimated.
From the table it can be seen that aromatic is broadly
coded by glomerular activations. Nearly 60% of
points were found to show differences significant at
the 5% level. Alkane covers the second biggest area
with about 40% of points. Carboxylic acid and ketone
are coded by about a third of all points. Coding zones
for properties alkene, alicyclic, and heterocyclic ex-
tend to between about 20 and 30%. For ester+lactone,
alkyne, and alcohol+phenol coding zones we mea-
sured between 10 and 16 percent of total. Properties
polycyclic, sulfur–containing compound and amine
recruit the smallest zones of compared properties with
about 7%, 4%, and 0.6%, respectively.
BIOSIGNALS 2010 - International Conference on Bio-inspired Systems and Signal Processing
40