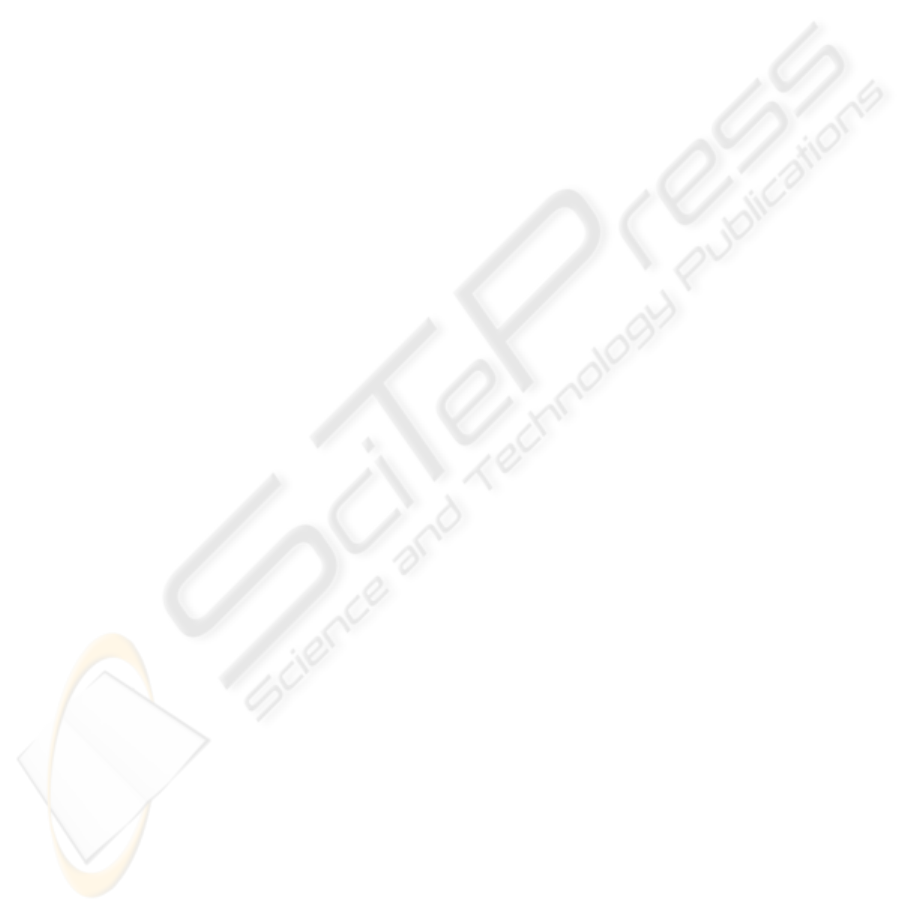
5 CONCLUSIONS
This article deals with outcome prediction of com-
bined models. We combined microarray and clini-
cal data. We described LOG/Z+BB/X approach and
its extension pre-LOG/Z+BB/X designed for redun-
dant datasets. In contrast to LOG/Z+BB/X, pre-
LOG/Z+BB/X includes pre-validationof models built
with microarray and clinical data followed by weights
calculation. Weights set relevance of microarray and
clinical models for data combination. We evalu-
ated LOG/Z+BB/X with non-redundant and redun-
dant simulated datasets for different predictive powers
of microarray and clinical variables. LOG/Z+BB/X
increases AUCs on non-redundant simulated datasets
and it does not decrease AUCs on redundant sim-
ulated datasets. Then we evaluated LOG/Z+BB/X
and pre-LOG/Z+BB/X on two benchmark breast can-
cer datasets. LOG/Z+BB/X increases AUCs on
Pittman dataset. Compared to LOG/Z+BB/X, pre-
LOG/Z+BB/X improves outcome of the prediction
up to 4% in the case of van’t Veer dataset. Aver-
age AUC for pre-LOG/Z+BB/X is 0.82. In conclu-
sion, LOG/Z+BB/X performs with combined mod-
els well—both with non-redundant data and redun-
dant data. When this approach does not perform well,
it is possible to apply pre-LOG/Z+BB/X approach
or evaluate the quality of data or models separately.
Plans to the future include incorporation of other
data sources into combination and deriving biomark-
ers significantly involved in outcome prediction.
ACKNOWLEDGEMENTS
This work was partly supported by the Czech Min-
istry of Education research grants 2B06052 and
MSM0021630528. We thank Petr Holub and the re-
viewers for their constructive comments.
REFERENCES
Boulesteix, A. L., Porzelius, C., and Daumer, M. (2008).
Microarray-based classification and clinical predic-
tors: on combined classifiers and additional predic-
tive value. Bioinformatics 24, 1698-1706.
Buhlmann, P. and Hothorn, T. (2007). Boosting Algorithms:
Regularization, Prediction and Model Fitting. Statist.
Sci. 22, 477-505.
Dupuy, A. and Simon, R. M. (2007). Critical Review of
Published Microarray Studies for Cancer Outcome
and Guidelines on Statistical Analysis and Reporting.
Journal of the National Cancer Institute 99 (2), 147-
157.
Eden, P., Ritz, C., and Rose, C. (2004). Good Old clinical
markers have similar power in breast cancer progno-
sis as microarray gene expression profilers. Eur. J.
Cancer 40 (12), 1803-1806.
Fernandez-Teijeiro, A., Betensky, R. A., Sturla, L. M., Kim,
J. Y., Tamayo, P., and Pomeroy, S. L. (2004). Combin-
ing gene expression profiles and clinical parameters
for risk stratification in medulloblastomas. J Clin On-
col. 22 (6), 994-998.
Fridlyand, J. and Yang, J. Y. H. (2004). DENMARK-
LAB R package. Advanced microarray data analysis:
Class discovery and class prediction. Available at
http://genome.cbs.dtu.dk/courses/norfa2004/Extras/.
Gajdos, C., Tartter, P. I., and Bleiweiss, I. (1999). Lym-
phatic Invasion, Tumor Size, and Age Are Indepen-
dent Predictors of Axillary Lymph Node Metastases in
Women With T1 Breast Cancers. Ann Surg. 230 (5),
692-696.
Gevaert, O., Smet, F. D., and Timmerman, D. (2006). Pre-
dicting the prognosis of breast cancer by integrating
clinical and microarray data with Bayesian networks.
Bioinformatics 22 (14), 184-190.
Gruvberger, S. K., Ringner, M., and Eden, P. (2003). Ex-
pression profiling to predict outcome in breast cancer:
the influence of sample selection. Breast Cancer Res.
5(1), 23-26.
Hosmer, D. W. and Lemeshow, S. (2000). Applied Logistic
Regression. Wiley, New York, 2nd edition.
Hothorn, T. and Buhlmann, P. (2007). mboost: Model-
Based Boosting. R package version 0.5-8. Bioinfor-
matics, Available at http://CRAN.R-project.org/.
Klijn, J. G. M., Wang, Y., Atkins, D., and Foekens, J. A.
(2005). Prediction of cancer outcome with microar-
rays. Lancet. 365 (9472), 1685-1685.
Ma, S. and Huang, J. (2007). Combining Clinical and Ge-
nomic Covariates via Cov-TGDR. Cancer Inform. 3,
371-378.
Michiels, S., Koscielny, S., and Hill, C. (2005). Prediction
of cancer outcome with microarrays: a multiple ran-
dom validation strategy. Lancet. 365 (9458), 488-492.
Molinaro, A., Simon, R., and Pfeiffer, R. M. (2005). Pre-
diction error estimation: a comparison of resampling
methods. Bioinformatics 21(15), 3301-3307.
Pittman, J., Huang, E., and Dressman, H. (2004). Inte-
grated modeling of clinical and gene expression in-
formation for personalized prediction of disease out-
comes. Proc.Natl.Acad.Sci. 101(22), 8431-8436.
Tibshirani, R. and Efron, B. (2002). Pre-validation and in-
ference in microarrays. Statistical applications in ge-
netics and molecular biology 1, 1.
van’t Veer, L. J., Dai, H., and van de Vijver, M. J. (2002).
Gene expression profiling predicts clinical outcome of
breast cancer. Nature 415, 530-536.
IMPROVED DISEASE OUTCOME PREDICTION BASED ON MICROARRAY AND CLINICAL DATA
COMBINATION AND PRE-VALIDATION
113