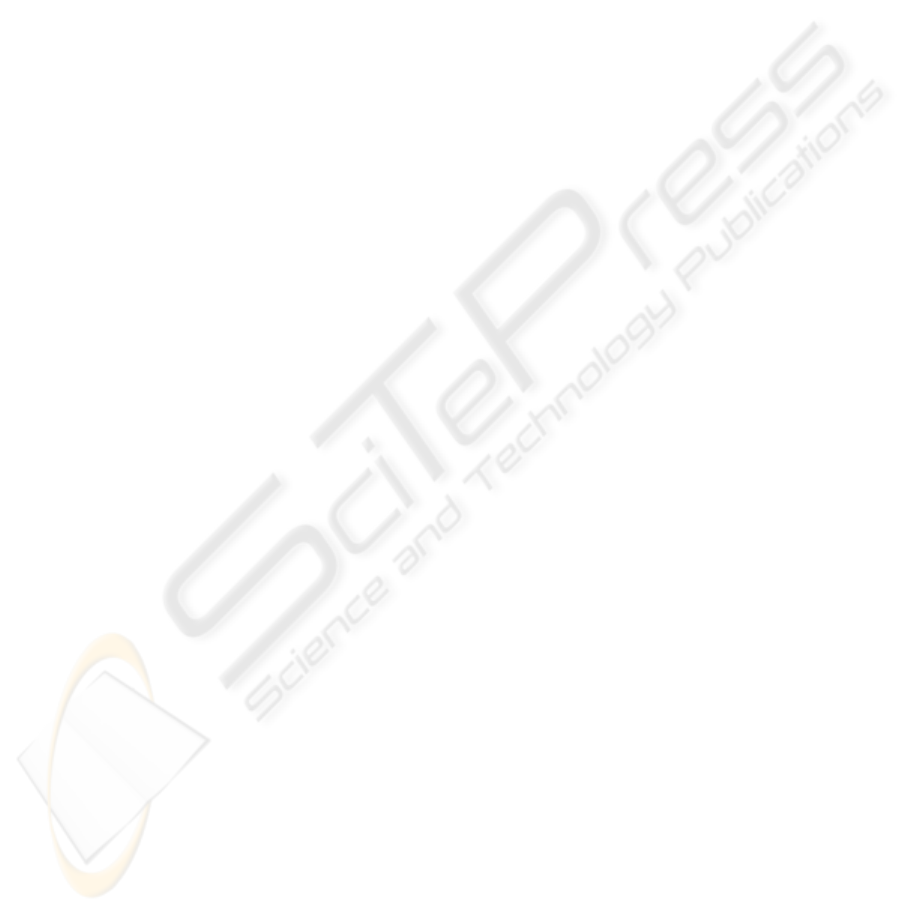
ing, were proposed. The limit of the vast majority of
these previous approaches is that they were focused
exclusively on the concept of probability where the
inference itself requires large numbers of prior and
conditional probabilities. For example, in the context
of assistive cognition within smart homes, requiring
humans to specify the habitat’s object involvement
probabilities is time consuming and difficult when
we consider all the potential objects involved in each
stage of an activity, given the large numbers of activ-
ities performed. Moreover, the probabilities do not
allow us to represent complete ignorance; besides,
there are numerous situations where it is not possi-
ble to give the agent probabilities based on statistical
measures, but only qualitative information provided
by expertsor deduced frompreviousexperiences. Our
proposed model, by using possibility theory, allows to
mitigate those limitations by taking into account par-
tial belief and by handling the behaviour hypotheses
as a partially ordered set.
4 CONCLUSIONS
This paper has presented a formal framework of ac-
tivities recognition based on possibilistic DL as the
semantic model of the observed agent’s behaviour.
This framework constitutes a first step toward a more
expressive ambient agent recognizer, which will fa-
cilitate to support the fuzzy and uncertainty con-
straints inherently to the smart environment. Cur-
rently, the proposed is under implementation in the
software framework of our smart home infrastructure,
which consists of a standard apartment with a kitchen,
living room, dining room, bedroom, and bathroom,
equipped with multiple sensor devices. Moreover, the
next logical step consists in conducting an extension
of this framework inorder to simultaneously dealwith
the vagueness of an activity’s duration and the noises
of the sensors. Finally, we clearly believe that consid-
erable future work and large scale experimentations
will be necessary, in a more advanced stage of our
work, to help evaluate the effectiveness of the model
in the field.
REFERENCES
Augusto, J. C. and Nugent, C. D., editors (2006). Design-
ing Smart Homes: The Role of Artificial Intelligence,
volume 4008 of LNAI. Springer.
Avrahami-Zilberbrand, D. and Kaminka, G. A. (2007).
Utility-based plan recognition: an extended abstract.
In Proc. of AAMAS’07, pages 858–860.
Baader, F., Calvanese, D., McGuinness, D. L., Nardi, D.,
and Patel-Schneider, P. F., editors (2007). The De-
scription Logic Handbook: Theory, Implementation,
and Applications. Cambridge University Press, sec-
ond edition.
Casas, R., Marín, R. B., Robinet, A., Delgado, A. R., Yarza,
A. R., Mcginn, J., Picking, R., and Grout, V. (2008).
User modelling in ambient intelligence for elderly and
disabled people. In Proc. of the 11th ICCHP, number
5105 in LNCS. Springer-Verlag.
Dubois, D. and Prade, H. (1988). Possibility Theory: An
Approach to Computerized Processing of Uncertainty.
Plenum Press.
Geib, C. (2007). Plan recognition. In Kott, A. and McE-
neaney, W. M., editors, Adversarial Reasoning: Com-
putational Approaches to Reading the Opponent’s
Mind, pages 77–100. Chapman & Hall/CRC.
Kautz, H. A. (1991). A formal theory of plan recognition
and its implementation. In Allen, J. F., Kautz, H. A.,
Pelavin, R. N., and Tenenberg, J. D., editors, Reason-
ing About Plans, chapter 2, pages 69–126. Morgan
Kaufmann.
Liao, L., Fox, D., and Kautz, H. (2004). Learning and infer-
ring transportation routines. In Proc. of the AAAI’04,
pages 348–353.
Mihailidis, A., Boger, J., Canido, M., and Hoey, J. (2007).
The use of an intelligent prompting system for peo-
ple with dementia: A case study. ACM Interactions,
14(4):34–37.
Philipose, M., Fishkin, K. P., Perkowitz, M., Patterson,
D. J., Fox, D., Kautz, H., and Hähnel, D. (2004). In-
ferring activities from interactions with objects. IEEE
Pervasive Computing: Mobile and Ubiquitous Sys-
tems, 3(4):50–57.
Pollack, M. E. (2005). Intelligent technology for an aging
population: The use of AI to assist elders with cogni-
tive impairment. AI Magazine, 26(2):9–24.
Roy, P., Bouchard, B., Bouzouane, A., and Giroux,
S. (2009). A hybrid plan recognition model
for Alzheimer’s patients: Interleaved–erroneous
dilemma. Web Intelligence and Agent Systems: An
International Journal, 7(4):375–397.
Zadeh, L. A. (1978). Fuzzy sets as a basis for a theory of
possibility. Fuzzy Sets and Systems, 1(1):3–28.
POSSIBILISTIC ACTIVITY RECOGNITION
189