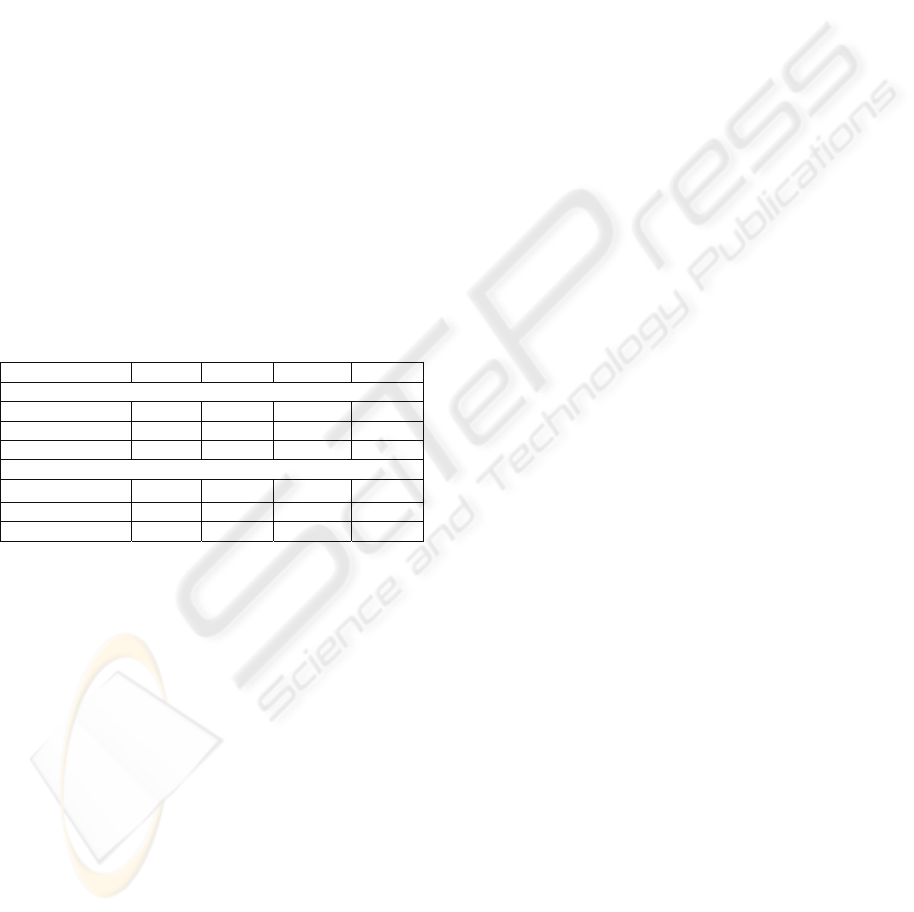
for determining the polarity of a word. The proposed
method detects a higher number of positive and
negative headlines (better recall), commits few
mistakes (better precision) and detects more neutral
headlines (better accuracy).
Finally, we compare our method with the
systems participating in SemEval 2007 Task 14 (see
Table 3). The results obtained by the unsupervised
systems CLaC and UPAR7, have very low recall and
high precision and, therefore, a very low value of
F1, indicating that few headlines (about 35 of 410)
are classified as positive and negative. Most
headlines are classified as neutral; therefore, the
accuracy is artificially high due to the imbalance of
classes in the data (155 Positives, 255 Negatives and
590 Neutrals).
On the other hand, the supervised systems
(except the SWAT that obtains very bad results)
show a different behavior with respect to
unsupervised systems, they have high recall but low
precision. These systems detect a greater number of
positive and negative headlines, but many neutral
ones are misclassified. Hence, they achieve a low
accuracy.
Table 3: Results of the valence annotation.
Acc. Prec. Rec. F1
Unsupervised methods
ClaC 55.10 61.42 9.20 16.00
UPAR7 55.00 57.54 8.78 15.24
Our method 44.3 37.66 72.11 49.41
Supervised methods
SWAT 53.20 45.71 3.42 6.36
CLaC-NB 31.20 31.18 66.38 42.43
SICS 29.00 28.41 60.17 38.60
As we can observe, the proposed method
outperforms both supervised and unsupervised
systems. Notice that it obtains the best F1 score and
recall while achieving acceptable values of precision
and accuracy. Therefore, we can conclude that our
method presents a more balanced behaviour, that is,
it performs well in the three classes: positive,
negative and neutral.
5 CONCLUSIONS
In this paper, a new unsupervised method to opinion
polarity detection has been introduced. Its most
important novelty is the use of word sense
disambiguation together with standard external
resources for determining the polarity of the
opinions. These resources allow the method to be
extended to other languages and be independent of
the knowledge domain.
The experiments carried out over the data of
SemEval Task No. 14 validate the useful of word
sense disambiguation for determining the polarity of
opinions. We have also shown that the proposed
method outperforms both unsupervised and
supervised systems participating in the competition.
Future work includes testing alternative
resources for polarity detection. We believe that in
many cases our approach fails because the wrong
annotations of SentiWordNet. We also plan to
evaluate the proposed method in other test
collections of different knowledge domain.
REFERENCES
Agirre, E., Soroa, A., (2007). Semeval-2007 task 02:
Evaluating word sense induction and discrimination
systems. Proceedings of the 4th International
Workshop on Semantic Evaluations (SemEval-2007),
7-12.
Anaya-Sánchez, H., Pons-Porrata, A., Berlanga-Llavori,
R. (2006). Word Sense Disambiguation based on
Word Sense Clustering. In J. Simão; H. Coelho and S.
Oliveira (Eds.), Lecture Notes in Artificial
Intelligence: Vol. 4140. IBERAMIA-SBIA (pp. 472-
481). Ribeirão Preto, Brazil: Springer.
Esuli, A., Sebastiani, F. (2006). SentiWN: A Publicly
Available Lexical Resource for Opinion Mining.
Proceedings of the Fifth international conference on
Language Resources and Evaluation (LREC 2006),
417-422.
Gil-García, R., Badía-Contelles, J. M., Pons-Porrata, A.
(2003). Extended Star Clustering Algorithm. In A.
Sanfeliu and J. Ruiz-Shulcloper (Eds.), Lecture Notes
in Computer Sciences: Vol. 2905. 8
th
Iberoamerican
Congress on Pattern Recognition (CIARP) (pp. 480–
487). Berlin, Heidelberg: Springer-Verlag.
Miller, G.A., Beckwith, R., Fellbaum, C., Gross, D.,
Miller, K. (1993). Introduction to WordNet: An On-
line Lexical Database. International Journal of
Lexicography, 3(4), 235- 244.
Schmid, H. (1994). Probabilistic Part-of-speech Tagging
Using Decision Trees. Proceeding of the Conference on
New Methods in Language Processing, 44-49.
Stone, P. J., Dunphy, D. C., Smith, M. S., Ogilvie, D. M.
(1966). The General Inquirer: A Computer Approach
to Content Analysis. The American Journal of
Sociology, 73(5), 634-635.
Strapparava, C., and Mihalcea, R. (2007). SemEval-2007
Task 14: Affective Text. Proceedings of the 4th
International Workshop on Semantic Evaluations
(SemEval 2007), 70-74.
ICAART 2010 - 2nd International Conference on Agents and Artificial Intelligence
486