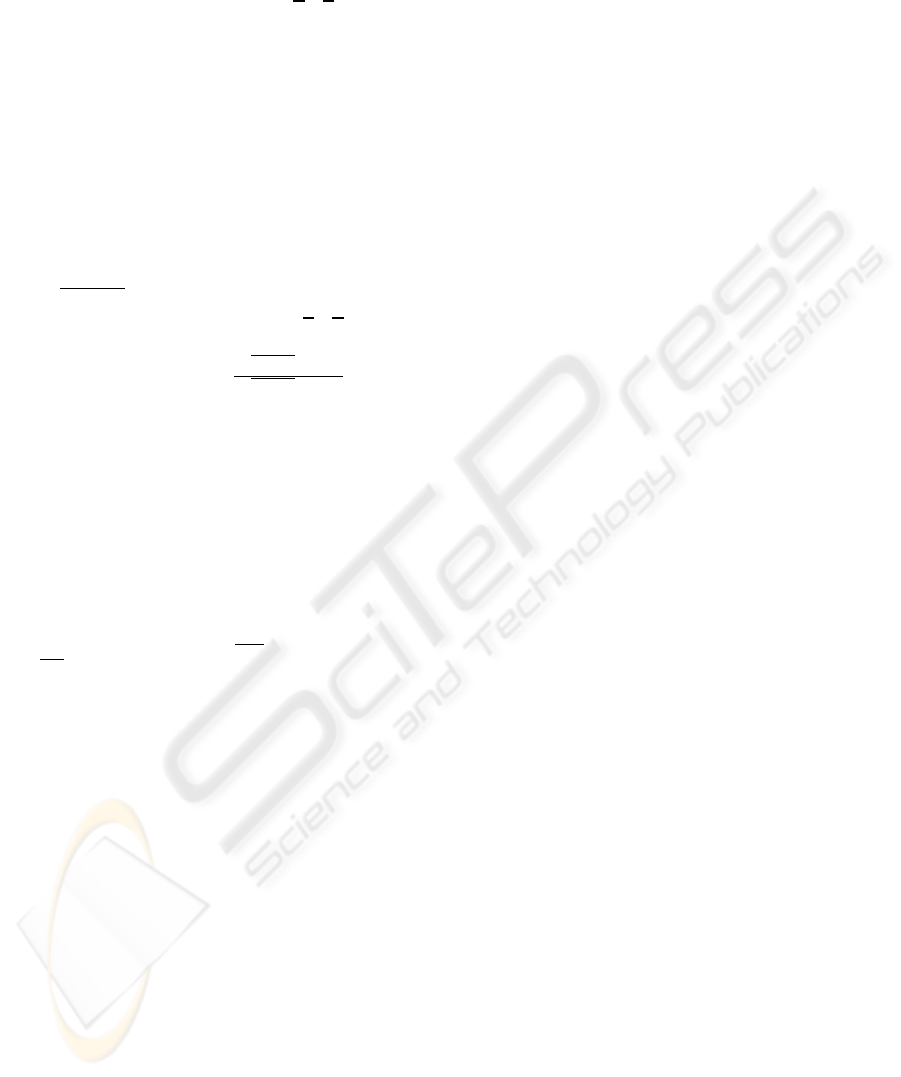
is obtained by the following strict separable system:
[k −1/2; k,k −1,k −2,...,1,1,...,1
| {z }
n−k
]
and such a tolerance is τ = 1/(2n + k
2
+ k −1).
Again, any other strict linearly (natural or not)
separating system (of non null weights) with tolerance
τ
0
fulfills τ
0
≤ τ.
In the same vein, we establish the corresponding
conjecture for the G-tolerance:
Conjecture 3.6 The maximum G-tolerance for n
variables and k types (k > 1) of distinguished vari-
ables is given by the following strict separable sys-
tem:
[
p
k(k −1);k,k −1,k −2,..., 1, 1, . . . , 1
| {z }
n−k
].
and such a G-tolerance is χ =
√
k(k−1)−(k−1)
√
k(k−1)+(k−1)
.
In general, given a strict separating system for an
arbitrary separable switching function, we are able to
find an equivalent strict separating system (with nat-
ural weights having minimum integer sum) achiev-
ing the maximum tolerance and the maximum G-
tolerance. Moreover, it is known, see (Freixas and
Molinero, 2008b), that for less than 8 variables all
linearly separable switching functions have, up to iso-
morphism, a unique strict separating system with nat-
ural weights having minimum sum. So, we only
need to adjust the threshold,
A+B
2
for the tolerance
and
√
AB for the G-tolerance, for these systems to
achieve the maximum tolerance and the maximum
G-tolerance, respectively. Note that for 8 variables
the situation changes: There are 154 linearly separa-
ble switching functions, up to isomorphism, with two
strict separating systems having natural weights and
minimum sum.
4 FUTURE WORK
Generating a huge number of random strict separating
natural systems and then to conjecturate what distri-
bution follows the tolerance, the maximum tolerance,
the G-tolerance and the maximum G-tolerance.
It is also interesting to provide tables of all, up
to isomorphism, linearly separable switching func-
tions of a reasonable high number of variables with:
a strict separating system achieving its tolerance; its
tolerance; a strict separating system achieving its G-
tolerance; and its G-tolerance.
Although the computational limitation (complex-
ity) of finding the tolerance and the G-tolerance of a
given strict separating system (both are NP-hard), it
will be of interest to develop an efficient algorithm
able to calculate the tolerance and the greatest toler-
ance for strict separating systems with a reasonable
high number of variables.
REFERENCES
Beiu, V., Quintana, J. M., and Avedillo, M. J. (2003).
Vlsi implementation of threshold logic-a comprehen-
sive survey. IEEE Transactions on Neural Networks,
14(5):1217–1243.
Elgot, C. C. (1961). Truth functions realizable by single
threshold organs. In AIEE Conference Paper 60-1311
(October), revised November 1960; paper presented
at IEEE Symposium on Switching Circuit Theory and
Logical Design.
Elizondo, D. (2004). Searching for linearly separable sub-
sets using the class of linear separability method. In
Proceedings of the 2004 International Joint Confer-
ence on Neural Networks (IJCNN 04), volume 2 of
IEEE, pages 955–959.
Elizondo, D. (2006). The linear separability problem: some
testing methods. IEEE Transactions on Neural Net-
works, 17(2):330–344.
Freixas, J. and Molinero, X. (2008a). The greatest allowed
relative error in weights and threshold of strict separat-
ing systems. IEEE Transactions on Neural Networks,
19(5).
Freixas, J. and Molinero, X. (2008b). On the existence of
a minimum integer representation for weighted voting
systems. Annals of Operations Research, 166(1):243–
260.
Hu, S. T. (1960). Linearly separable switching functions.
Technical report, Technical report, LMSC, Technical
Document, LMSD-703024.
Hu, S. T. (1965). Threshold Logic. Univ. of California
Press. xiv + 338 pp. Let xx, x2.
Myhill, J. and Kautz, W. H. (1961). On the size of weights
required for linear–input switching functions. IRE-
Transactions, EC-10.
Ozdemir, H., Kepkep, A., Pamir, B., Leblebici, Y., and
Cilingiroglu, U. (1996). A capacitive threshold logic
gate. IEEE J. Solid–State Circuits, 31.
Picton, P. D. (1991). Neural Networks. Palgrave, second
edition.
Roychowdhury, V., Siu, K., and (Eds.), A. O. (1994). The-
oretical Advances in Neural Computation and Learn-
ing. Kluwer Academic Publishers, Stanford, USA.
Sang-Hoon, O. and Lee, Y. (1995). Sensitivity analysis
of single hidden-layer neural networks with threshold
functions. IEEE Trans. Computers, 6(4):1005–1007.
Siu, K., Roychowdhury, V., and Kailath, T. (1995). Dis-
crete Neural Computation: A Theoretical Foundation.
Prentice Hall, New Jersey, USA.
Winder, R. O. (1962). Threshold logic. PhD thesis, Depart-
ment of Mathematics, Princeton University.
Yao, S. S. and Ostapko, D. L. (1968). Realization of a
class switching functions by threshold-logic networks.
IEEE Transactions on Computers, C-17(4):391–399.
ICAART 2010 - 2nd International Conference on Agents and Artificial Intelligence
514