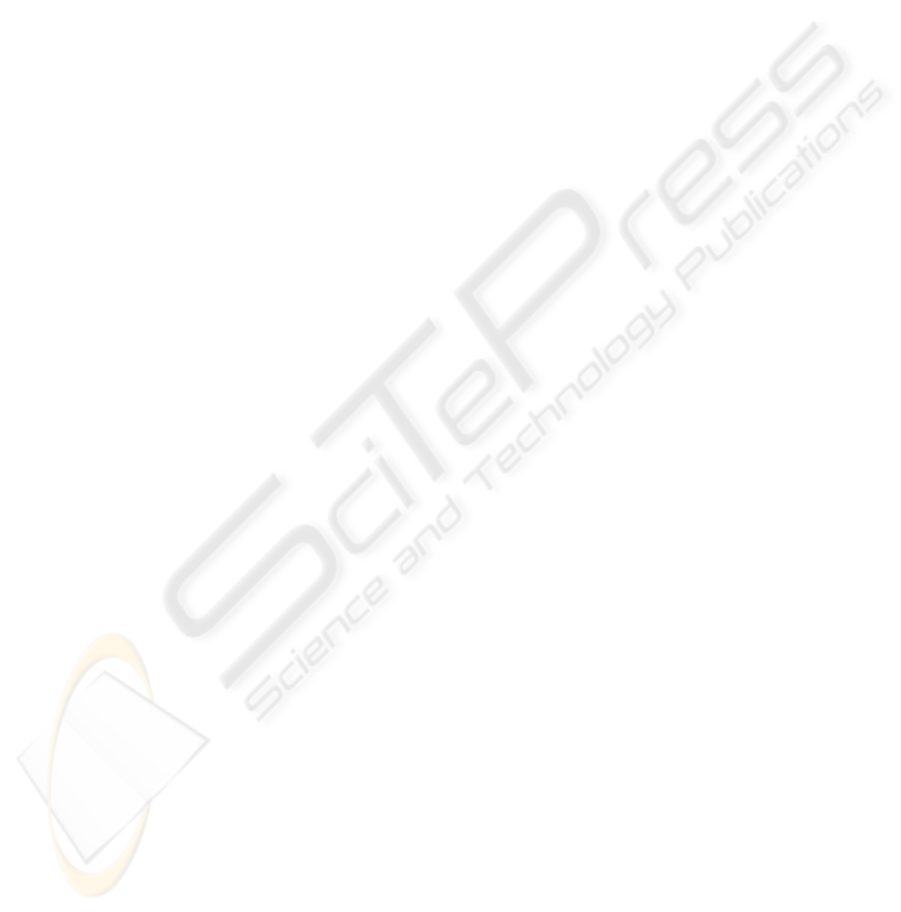
RATIO-HYPOTHESIS-BASED FUZZY FUSION WITH
APPLICATION TO CLASSIFICATION OF CELLULAR
MORPHOLOGIES
Tuan D. Pham
School of Engineering and Information Technology, University of New South Wales, Canberra, ACT 2600, Australia
Xiaobo Zhou
Center for Biotechnology and Informatics, Methodist Hospital Research Institute, Houston, TX 77030, U.S.A.
Keywords:
Permanence of ratio, Information fusion, Pattern classification, Cellular phenotypes, Bio-imaging.
Abstract:
Fusion of knowledge from multiple sources for pattern recognition has been an active area of research in
many scientific disciplines. This paper presents a fuzzy version of a probabilistic fusion scheme, known
as permanence-of-ratio-based combination, with application to analysis of cellular imaging for high-content
screening. Classification of cellular phenotypes has been carried out to illustrate the usefulness of the
permanence-of-ratio-based fuzzy fusion.
1 INTRODUCTION
Information or data fusion can be defined as the use of
mathematical methods that combine data from multi-
ple sources to obtain the resultant knowledge in or-
der to achieve inferences, which will be more effi-
cient and potentially more accurate than if they were
achieved by means of considering separate single
sources. Information fusion can be performed on a
low-level or high-level process depending on the pro-
cessing stage at which fusion takes place. Low-level
fusion combines several sources of raw data to pro-
duce new raw data (Pellizzeri et al., 2002). The ex-
pectation is that fused data is more informative and
synthetic than the original raw data. High-level fusion
typically combines features from multiple classifiers,
or signals from multiple sensors for logical decision
making (Muller et al., 2001; Das, 2008).
There are many mathematical operators developed
for data fusion such as the averaging rule, multiplica-
tion rule, probabilistic models, mathematical theory
of evidence, machine learning methods, and fuzzy in-
tegral (Chi et al., 1996). For high-level fusion, the ra-
tionale of combining knowledge from various sources
is that it is always difficult or impossible to design
a single classifier or to use a single feature for pat-
tern classification to achieve the best results, because
a particular classifier or feature can only be robust for
handling a particular identity of an object, which may
vary under different settings. Furthermore, different
problems may require different data fusion methods
to obtain effective solutions depending on the types
of features.
This paper discusses the use of fuzzy measures for
combining evidences from multiple sources, where
the strong assumption of data independence is relaxed
(Journel, 2002). The utilization of such novel idea ap-
pears to be promising for pattern classification but it is
still rarely explored in the new field of bioinformatics.
We are interested in applying an information fusion
scheme for combining output from multiple classi-
fiers in order to improvethe results for classifying var-
ious cellular phenotypes for robust automated analy-
sis of genome-wide high-content screening of fluores-
cent microscopy images of cells.
The rest of this paper is organized as follows. Sec-
tion 2 introduces the concept of the permanence of
ratio hypothesis for knowledge combination. Sec-
tion 3 proposes a ratio-hypothesis-based fuzzy fusion
scheme. Section 4 illustrates the application of the
proposed fusion model for classifying cellular pheno-
types for genome-wide screening, such screening is
essential to the rapid discovery of basic biological cell
principles such as control of cell cycle and cell mor-
202
D. Pham T. and Zhou X. (2010).
RATIO-HYPOTHESIS-BASED FUZZY FUSIONWITH APPLICATION TO CLASSIFICATION OF CELLULAR MORPHOLOGIES.
In Proceedings of the Third International Conference on Bio-inspired Systems and Signal Processing, pages 202-207
DOI: 10.5220/0002707902020207
Copyright
c
SciTePress