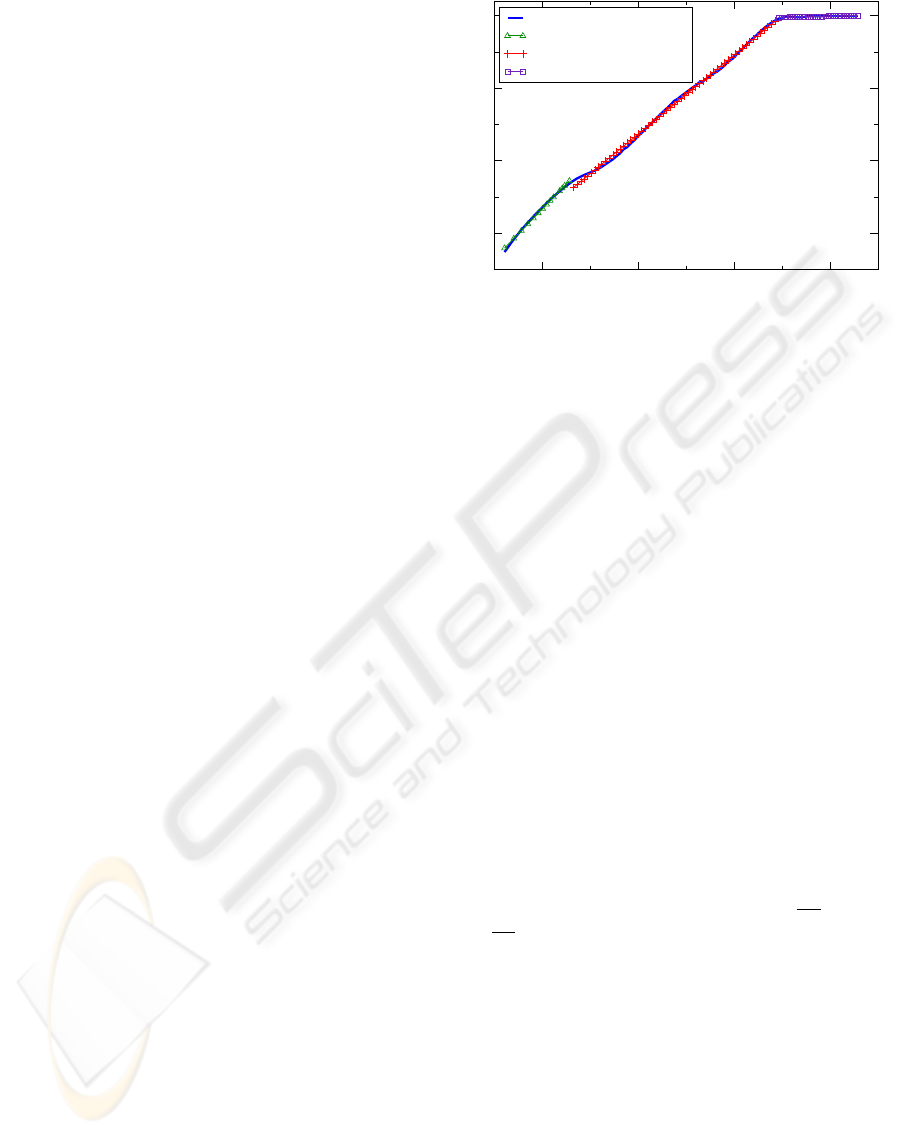
C
m+1
/C
m
is commonly thought of as the informa-
tion gained as the trajectory moves from time mτ to
(m + 1)τ. A larger difference between C
m
and C
m+1
results in more information, i.e., a higher value of SE.
For a fixed value of m the graph of the sample entropy
over a range of τ’s provides a measure of the amount
of long range correlation in the time series. A rela-
tively constant amount of entropy across many values
of τ signifies correlations amongst data points over
multiple time scales. For instance, 1/ f noise which is
highly correlated across time scales yields a constant
MSE curve. In contrast, white noise is monotonically
decreasing since it possesses no long range correla-
tions.
4 RESULTS
Consider an in-silico neural network N as defined in
Section 2.2. We construct the time series of voltage
potentials ¯g(n) by choosing a set of initial conditions
and solving the system of differential equations over
the time interval [0, N], then performing the binning
procedure described in (3). The units of time are ar-
bitrary.
Figure 2 shows the time series, DFA curve, and
MSE curve for a simulation of N run for N = 15000.
In Figure 2(b), the scaling exponent β = 1.063 over
the range of window sizes w = 10
1.3
to w = 10
2.7
.
Thus, running N for a relatively short simulation pro-
duces power law scaling similar to a physiological
system over the range (1.3,2.7) for the total length of
range 1.4 . This range of scales where β ≈ 1 is shorter
than that typically seen in biological systems, where
the range typically has length greater than 3. The DFA
curve extracted from our simulations has three dis-
tinct regions. In the first region, where w < 10
1.3
, the
linear regression deviates from the power law β ≈ 1
due to autocorrelation effects on short scales, which
are caused by the deterministic ODE solver. These
effects dominate at scales much smaller than the high-
est frequency cellular oscillations, which can be esti-
mated from the largest ε. Figure 3 illustrates this ef-
fect; we compare the DFA curve in Figure 4(b) to the
curve obtained after dividing by 10 all of the ε’s used
in generating Figure 4(b). The deterministic portion
extends to higher scales because the highest oscilla-
tion frequency decreased by a factor of ten. This also
illustrates the importance of the choice of ε’s on the
DFA curve. In order to avoid these deterministic ef-
fects we focus (for the original set of ε’s listed in the
Appendix) on power law scaling in the second region,
where w > 10
1.3
.
In Figure 2(b) we see that N produces physiologi-
1 2 3 4
log(w)
-1
0
1
2
log F(w)
log F(w)
Linear reg slope = 1.37
Linear reg slope = 1.091
Linear reg slope = 0.031
Figure 5: The DFA curve after simulating N for N =
400000. The region of complexity remains unchanged from
that seen in Figure 4(b). This implies that N has an inherent
limit for generating long range correlations.
cally complex behavior in the region w > 10
1.3
, which
ends at w = 10
2.7
. By increasing the length of the
time series (Figure 4(b)) the second region extends
past w = 10
2.7
to w = 10
3.5
showing that N continues
to introduce complexity into the time series past the
scale limits imposed by the short simulation in Fig-
ure 2. The second region terminates in Figure 4(b)
at w = 10
3.5
, where a third region with no long range
correlation begins. Extending the length of the sim-
ulation to N = 400000 yields the DFA curve in Fig-
ure 5, where this third region extends to larger win-
dow sizes. Clearly the extension of the time series
fails to find longer range correlations in the time se-
ries. We conclude that the system N has an upper
limit w = 10
3.5
on the length of long range correla-
tions it can generate.
The longer time series yields an MSE curve that is
relatively constant, mimicking the behavior observed
in simulation of 1/ f -noise as well as free-running
physiological systems. It maintains an entropy level
nearly identical to the MSE curve in Figure 2(c). In-
deed, the average entropies for τ ≥ 5 are SE = 0.34
and SE = 0.35, respectively. The MSE curve in Fig-
ure 2(c) derived from the shorter time series suffers
larger variations due to coarse graining effects on the
relatively low number of data points in the original
series. Nevertheless, as the comparison of the aver-
ages shows the MSE and sample entropy measures
for shorter simulations are consistent with the results
from longer simulations, and still provide good in-
sight into the complexity of the network.
Furthermore, the behavior of N does not depend
on initial conditions as we confirm by choosing ran-
dom initial conditions for excitatory cells uniformly
in the interval (−5,5). To illustrate, we present a
typical case in which we alter the initial condition of
MODELING COMPLEXITY OF PHYSIOLOGICAL TIME SERIES IN-SILICO
65