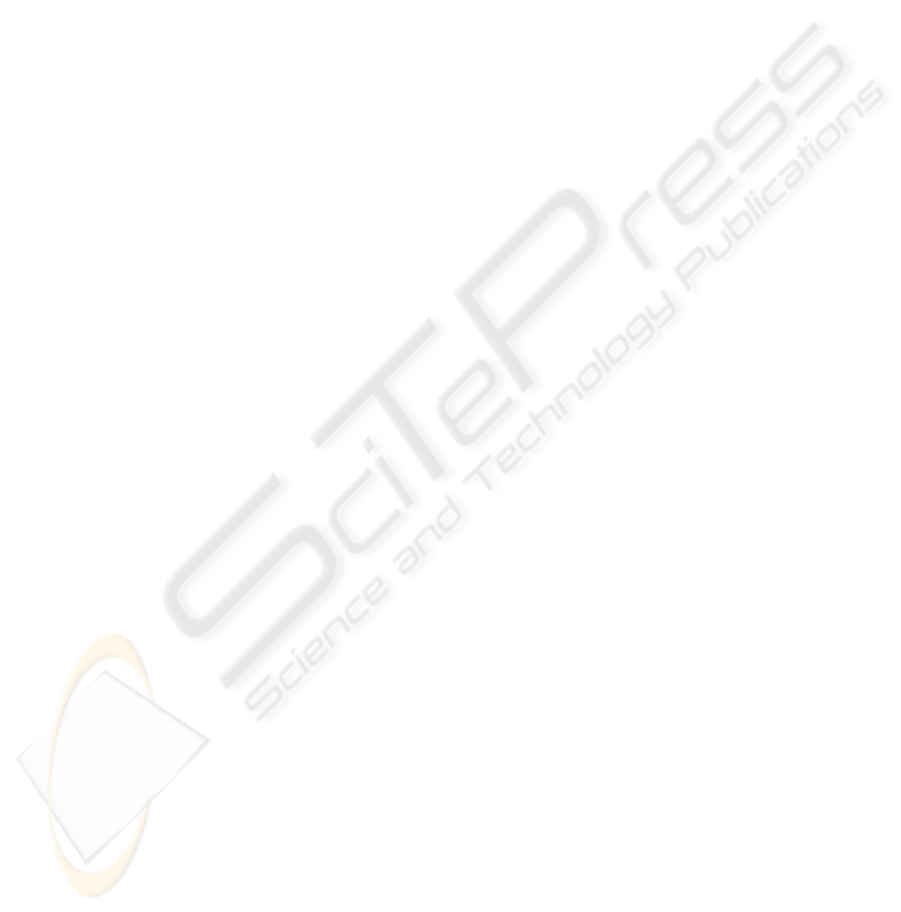
for this technique is the fact that different methods
sometimes produce conflicting predictions for the
same instance. Thus, a system that reliably identifies
the best predictor for a given instance will achieve
better predictive performance than any of the
individual predictors. The experimental evaluation
presented in this paper focuses on predicting
survival time of pancreatic cancer patients based on
attributes such as demographic information, initial
symptoms, and diagnostic test results. The
evaluation results show that the proposed technique
of model selection meta-learning produces
predictions that are better than those of the
individual machine learning methods. Also, the
proposed technique outperforms the standard meta-
learning techniques of bagging, boosting, and
stacking in the experiments conducted for this paper.
Further work is needed to better establish the
magnitude of observed performance differences, and
to determine whether any particular machine
learning predictors are best suited to being combined
through the model selection meta-learning technique
introduced in this paper.
REFERENCES
Bhanot, G., Alexe, G., Venkataraghavan, B., Levine, A.J.
A robust meta-classification strategy for cancer
detection from MS data, Proteomics 2006, 6:592-604.
Breiman, L.. Bagging predictors, Machine Learning 24(2):
123-140, 1996.
Floyd, S., Alvarez, S. A., Ruiz, C., Hayward, J., Sullivan,
M., Tseng, J., and Whalen, G. Improved survival
prediction for pancreatic cancer using machine
learning and regression, Society for the Surgery of the
Alimentary Tract 48th Annual Meeting (SSAT 2007),
Washington DC, USA, May 19-23, 2007.
Freund, Y. and Schapire, R.E. A decision-theoretic
generalization of on-line learning and an application to
boosting, Journal of Computer and System Sciences,
55(1):119--139, 1997.
Ge, G. and Wong, G.W. Classification of premalignant
pancreatic cancer mass-spectrometry data using
decision tree ensembles, BMC Bioinformatics 2008,
9:275
Hayward, J., Alvarez, S.A., Ruiz, C., Sullivan, M., Tseng,
J., and Whalen, G. Knowledge discovery in clinical
performance of cancer patients, IEEE International
Conference on Bioinformatics and Biomedicine
(BIBM08), Philadelphia, PA, USA, Nov. 3-5, 2008.
Honda, K., Hayashida, Y., Umaki, T., Okusaka, T.,
Kosuge, T., Kikuchi, S., Endo, M., Tsuchida, A.,
Aoki, T., Itoi, T., Moriyasu, F., Hirohashi, S.,
Yamada, T. Possible detection of pancreatic cancer by
plasma protein profiling. Cancer Res. 2005 Nov 15;
65(22):10613-22.
Horner, M.J., Ries, L.A.G., Krapcho, M., Neyman, N.,
Aminou, R., Howlader, N., Altekruse, S.F., Feuer,
E.J., Huang, L., Mariotto, A., Miller, B.A., Lewis,
D.R., Eisner, M.P., Stinchcomb, D.G., Edwards, B.K.
(eds). SEER Cancer Statistics Review, 1975-2006,
National Cancer Institute. Bethesda, MD,
http://seer.cancer.gov/csr/1975_2006/, based on
November 2008 SEER data submission, posted to
SEER web site, 2009.
Mitchell, T. Machine Learning, McGraw-Hill, 1997.
Qu, Y., Adam, B.L., Yasui, Y., Ward, M.D., Cazares,
L.H., Schellhammer, P.F., Feng, Z., Semmes, O.J.,
Wright, G.L. Jr.: Boosted decision tree analysis of
surface-enhanced laser desorption/ionization mass
spectral serum profiles discriminates prostate cancer
from noncancer patients. Clin Chem 2002, 48:1835-
1843.
Witten, I.H and Frank, E. Data Mining. 2
nd
ed. Morgan
Kaufmann Publishers. 2005.
Wolpert, D.H. Stacked generalization, Neural Networks,
Vol. 5, pp 241-259, 1992.
MODEL SELECTION META-LEARNING FOR THE PROGNOSIS OF PANCREATIC CANCER
37