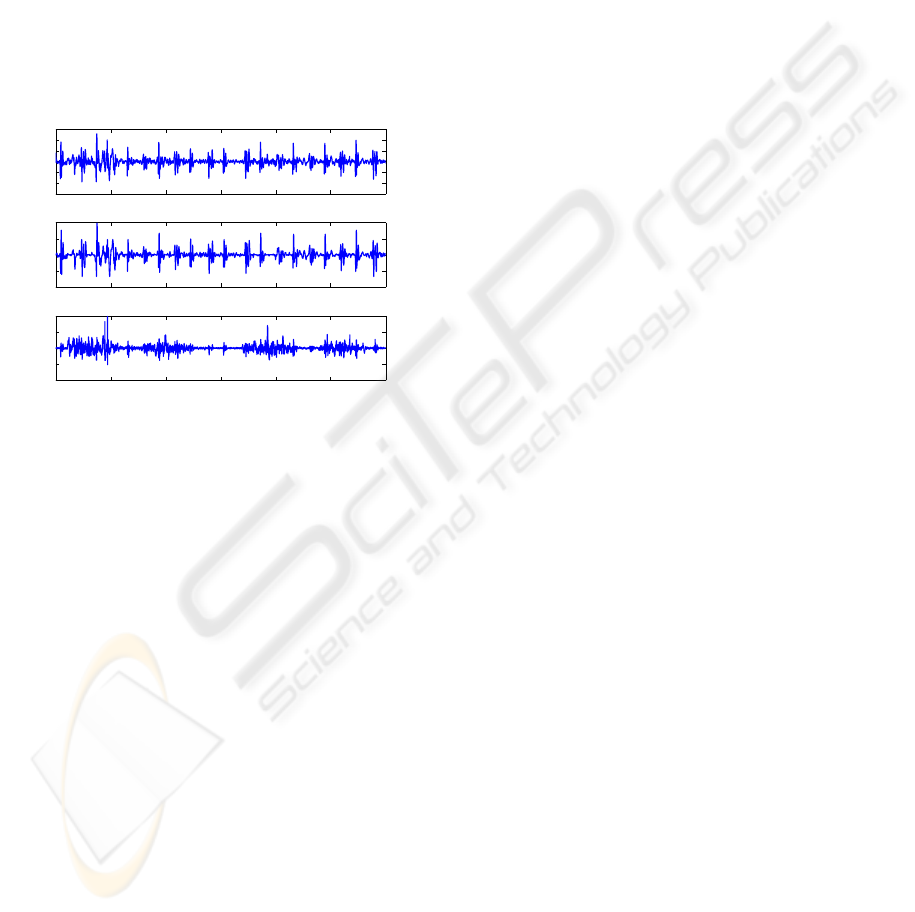
frequency F
s
=11.025 kHz. Test subjects were asked
to breathe normally with no targeted flow. The char-
acteristics due to sex, age, weight were not taken into
consideration.
5.2.2 Evaluation
To evaluate the effectiveness of the NMF based tech-
nique, the separation performance are tested on sin-
gle channel separation of heart and breath sounds. As
shown in Fig. 5, the separation performance is quite
good and is shown to pass the subjective test for sepa-
ration. This proves that the proposed model and asso-
ciated parameters are consistent with those required
for separating the real recorded data.
0 0.5 1 1.5 2 2.5 3
x 10
5
−0.2
−0.1
0
0.1
0.2
(a)
0 0.5 1 1.5 2 2.5 3
x 10
5
−1
−0.5
0
0.5
1
(b)
Magnitude
0 0.5 1 1.5 2 2.5 3
x 10
5
−1
−0.5
0
0.5
1
Samples
(c)
Figure 5: Single channel source separation using NMF. (a)
Observed signal; (b) Separated HS; (c) Separated RS.
6 CONCLUSIONS
Non-negative matrix factorization techniques are
shown to perform well in case of single channel
source separation. The convolutive mixing model for
respiratory sounds has been verified based on the sep-
aration performance. The NMF technique, when used
on respiratory sounds, provides an SIR improvement
of over 10 dB for optimal sensor positions. This, on
the other hand, suggests an optimal sensor position
for sound capturing. Due to the good separation per-
formance, this has potential medical applications for
accurate detection of pulmonary and heart diseases
based on the separated RS and HS respectively.
REFERENCES
Abrard, F. and Deville, Y. (2005). A time-frequency blind
signal separation method applicable to underdeter-
mined mixtures of dependent sources. Signal Process-
ing, 85(7):1389–1403.
Bingham, E., Hyvrinen, A., and rinen, A. H. (2000). A
fast fixed-point algorithm for independent component
analysis of complex valued signals. Int. J. of Neural
Systems, 10:1–8.
Cardoso, J. F. and Souloumiac, A. (1993). Blind beam-
forming for non Gaussian signals. IEE Proceedings,
140(6):362–370.
Charleston-Villalobos, S., Aljama-Corrales, A. T., and
Gonzalez-Camarena, R. (2006). Analysis of simu-
lated heart sounds by intrinsic mode functions. Proc.
of 28th IEEE EMBS Conf., pages 2848–2851.
Choi, S., Cichocki, A., Park, H. M., and Lee, S. Y. (2005).
Blind source separation and independent component
analysis: A review. Neural Information Processing -
Letters and Reviews, 6(1):1–57.
Cort´es, S., Jan´e, R., Fiz, J. A., and Morera, J. (2005). Mon-
itoring of wheeze duration during spontaneous respi-
ration in asthmatic patients. Proc. 27th IEEE EMBS
Conf.
Gavriely, N. (1999). Automatic detection and analysis of
breath sounds. Eur. Patent, (EP 0 951 867 A2).
Jang, G.-J. and Lee, T.-W. (2003). A maximum likelihood
approach to single-channel source separation. J. Ma-
chine Learning Res., 4:1365–1392.
Kompis, M., Pasterkamp, H., Motai, Y., and Wodicka, G. R.
(1998). Spatial representation of thoracic sounds.
Proc. 20th IEEE EMBS Conf., pages 1661–1664.
Lee, D. and Seung, H. (1999). Learning the parts of ob-
jects by non-negative matrix factorization. Nature,
401(6755):788–791.
Lehrer, S. (2002). Understanding Lung Sounds. Philadel-
phia, PA: Saunders, Audio CD.
MIT (1999). ICA Synthetic Benchmarks.
Schmidt, M. N. and Mørup, M. (2006). Nonnegative ma-
trix factor 2-D deconvolution for blind single channel
source separation. In Int. Conf. on ICA and Signal
Separation.
Sovij¨arvi, A. R. A., Vanderschoot, J., and Eavis, J. R.
(2000). Standardization of computerized respira-
tory sound analysis. European Respiratory Review,
10(77):585–649.
Van der Veen, A. J. and Paulraj, A. (1996). An analytical
constant modulus algorithm. IEEE Trans. Signal Pro-
cessing, 44(5):1136–1155.
Wilkins, R. L., Hodgkin, J. E., and Lopez, B. (2004). Fun-
damentals of Lung and Heart Sounds. Mosby, Audio
CD.
Zhao, Z. D. and Wang, Y. (2007). Analysis of diastolic
murmurs for coronary artery diseasebased on hilbert
huang transform. Proc. of Int. Conf. Machine Learn-
ing and Cybernetics, 6.
BIOSIGNALS 2010 - International Conference on Bio-inspired Systems and Signal Processing
224