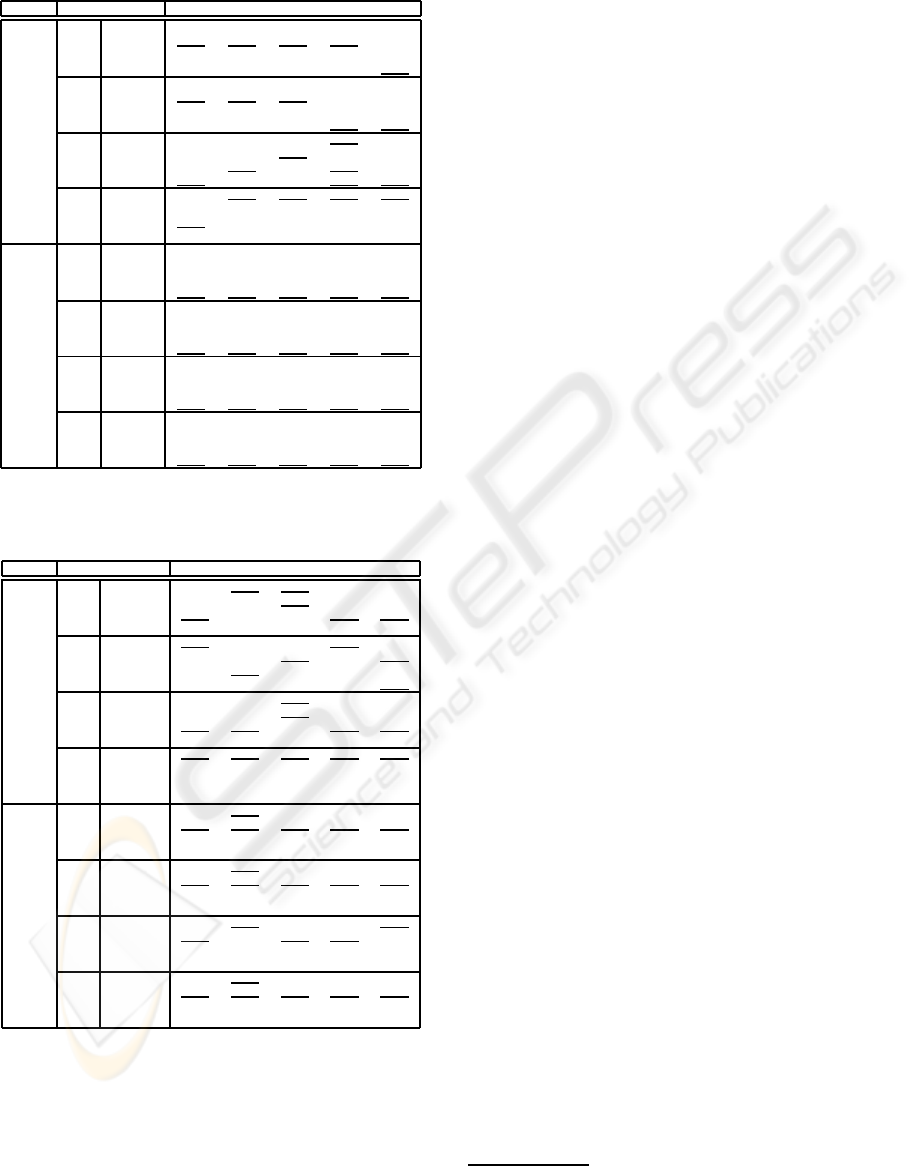
Table 1: Classification accuracies (%) of PS-based DBCs.
data methods knnc nmc ldc qdc svc
Rand 80.00 77.58 84.24 75.76 84.85
ED RandC 80.61 81.82 96.97 79.39 56.97
KCenter 77.58 80.00 89.70 75.76 85.45
ModeS 78.18 78.79 88.48 76.36 89.09
Rand 75.15 73.94 71.52 76.36 76.36
Yale HD RandC 75.76 80.00 89.70 73.33 75.76
KCenter 73.94 74.55 76.97 75.15 80.61
ModeS 75.15 78.18 75.76 80.00 87.88
Rand 77.58 71.52 90.91 76.36 86.06
RD RandC 78.79 70.91 98.79 75.15 86.67
KCenter 78.79 76.97 96.97 76.36 86.67
ModeS 79.39 74.55 97.58 76.36 89.09
Rand 72.12 52.12 80.00 71.52 78.79
WD RandC 71.52 49.09 79.39 70.91 76.36
KCenter 74.55 49.70 79.39 70.30 76.36
ModeS 71.52 49.09 76.97 69.70 74.55
Rand 79.10 77.10 80.80 82.00 71.10
ED RandC 90.40 84.90 90.00 91.00 89.10
KCenter 80.00 79.50 83.60 84.80 76.80
ModeS 97.40 85.40 97.80 99.30 96.50
Rand 80.70 80.20 82.70 84.00 81.70
Nist38 HD RandC 90.00 82.00 88.50 90.00 86.80
KCenter 81.30 78.30 83.00 84.00 73.60
ModeS 97.40 85.40 97.80 99.30 97.80
Rand 84.50 80.70 86.30 85.70 0
RD RandC 93.80 85.10 91.60 93.00 0
KCenter 90.80 86.40 91.10 91.40 90.90
ModeS 97.00 86.90 97.80 98.90 97.70
Rand 78.90 70.50 77.10 78.50 75.10
WD RandC 87.40 73.90 84.40 86.60 80.80
KCenter 79.90 74.70 79.70 81.80 64.00
ModeS 93.70 77.50 95.50 96.80 95.20
Table 2: Classification accuracies (%) of DR-based DBCs.
data methods knnc nmc ldc qdc svc
LDA 89.70 93.94 93.94 83.03 89.70
ED PCA 79.39 80.61 93.94 79.39 46.67
PCALDA 90.30 92.73 89.09 83.64 96.97
CLDA 89.70 89.70 91.52 82.42 93.33
LDA 82.42 81.21 81.21 79.39 84.85
Yale HD PCA 75.76 77.58 86.67 78.79 89.70
PCALDA 79.39 84.24 80.00 72.12 87.88
CLDA 81.21 81.21 81.21 76.36 89.70
LDA 90.91 96.97 96.97 84.24 94.55
RD PCA 79.39 75.15 96.97 77.58 86.06
PCALDA 98.79 98.79 93.33 89.70 99.39
CLDA 89.09 89.09 87.88 71.52 81.21
LDA 80.00 83.03 83.03 73.33 77.58
WD PCA 70.91 49.70 72.73 70.91 73.33
PCALDA 75.76 76.97 70.91 60.61 63.03
CLDA 64.24 64.24 60.00 48.48 42.42
LDA 84.80 87.50 87.50 87.80 87.00
ED PCA 98.10 87.50 98.00 99.30 96.60
PCALDA 71.50 71.60 71.60 70.80 71.40
CLDA 63.80 63.80 63.80 80.10 63.80
LDA 84.80 87.50 87.50 87.80 87.00
Nist38 HD PCA 98.10 87.50 98.00 99.30 97.70
PCALDA 71.50 71.60 71.60 70.80 0
CLDA 63.80 63.80 63.80 80.10 63.80
LDA 86.90 88.30 88.20 88.90 85.60
RD PCA 97.30 88.20 97.10 98.60 0
PCALDA 58.10 58.10 58.10 58.00 0
CLDA 50.40 50.40 49.10 49.50 50.40
LDA 68.00 76.90 76.90 77.30 76.20
WD PCA 92.50 76.90 97.00 97.00 96.30
PCALDA 55.80 55.80 55.80 55.70 0
CLDA 52.70 52.70 50.10 59.60 52.70
four of DRs) methods per each classifier and ranked
them in the order from the highest to the lowest clas-
sification accuracies. Although this comparison is a
very simplistic model of comparison, we believe that
it is the easiest approach a researcher can employ
when dealing with algorithms that havedifferent char-
acteristics.
From the rankings obtained from Tables 1 and 2,
we can clearly observe the possibility of improving
the performance of DBCs by utilizing the DRs. In
most instances, the averaged classification accuracies
of DR-based DBCs are increased compared to those
of PS-based ones (note that almost all of the highest
rankings are those of DR-based methods.) However,
some DR-based DBCs failed to improve their clas-
sification accuracies
5
. From this consideration, we
can see that it is difficult for us to grade the meth-
ods as they are. Therefore, for simple comparisons,
we first assigned marks of 3, 2, or 1 to all DR and
PS methods according to their ranks; 3 marks are
given to the 1
st
rank; 2 marks for the 2
nd
, and 1
mark for the 3
rd
. Then, we added up all the marks
that each method earned with the five classifiers and
the four measuring methods. For example, the marks
that LDA gained in ED, HD, RH, and WD rows
are 10(= 2 + 3 + 2 + 2 + 1), 8(= 3 + 2 + 1 + 2 + 0),
9(= 2+2+1+2+2),and 14(= 3+3+3+3+2),re-
spectively. Thus, the total mark that the LDA earned
is 41. Using the same system, we graded all the other
DR (and PS) methods, and, as a final ranking, we ob-
tained the followings:
(1) For Yale, 1
st
: LDA (41); 2
nd
: PCALDA (32);
3
rd
: CLDA (17).
(2) For Nist38, 1
st
: PCA (51); 2
nd
: ModeS (45);
3
rd
: RandC (14).
Here, the number (·) of each DR (or PS) method
represents the final grade it obtained. From this rank-
ing, we can see that all of the highest ranks are of DR
methods. Thus, in general, it should be mentioned
that more satisfactory optimization of DBCs can be
achieved by applying a DR after building the dissim-
ilarity space with all of the available samples rather
than by selecting the representative subset from them.
As analyzed in Section 2, choosing the entire
training set as the representative prototypes leads to
higher computational complexity as more distances
have to be calculated. In comparing PS-based and
DR-based methods, we simply measured the process-
ing CPU-times (seconds) of the DBCs designed with
the two databases. In the interest of space, the de-
tails of the measured times are omitted here. From the
measures, however, we can observe that the process-
ing CPU-times increased when DR-based methods
were applied. An instance of this change is the pro-
cessing times of ED for Yale. The processing times
of LDA, PCA, PCALDA, and CLDA methods are, re-
spectively, 0.0250, 0.2161, 0.2521, and 27.9266 (sec-
onds), while those of Rand, RandC, KCenter, and
ModeS are, respectively, 0.0182, 0.0099, 0.0870, and
5
For this failure, we are currently investigating why it
occurs and what the cause is.
ON REDUCING DIMENSIONALITY OF DISSIMILARITY MATRICES FOR OPTIMIZING DBC - An Experimental
Comparison
239