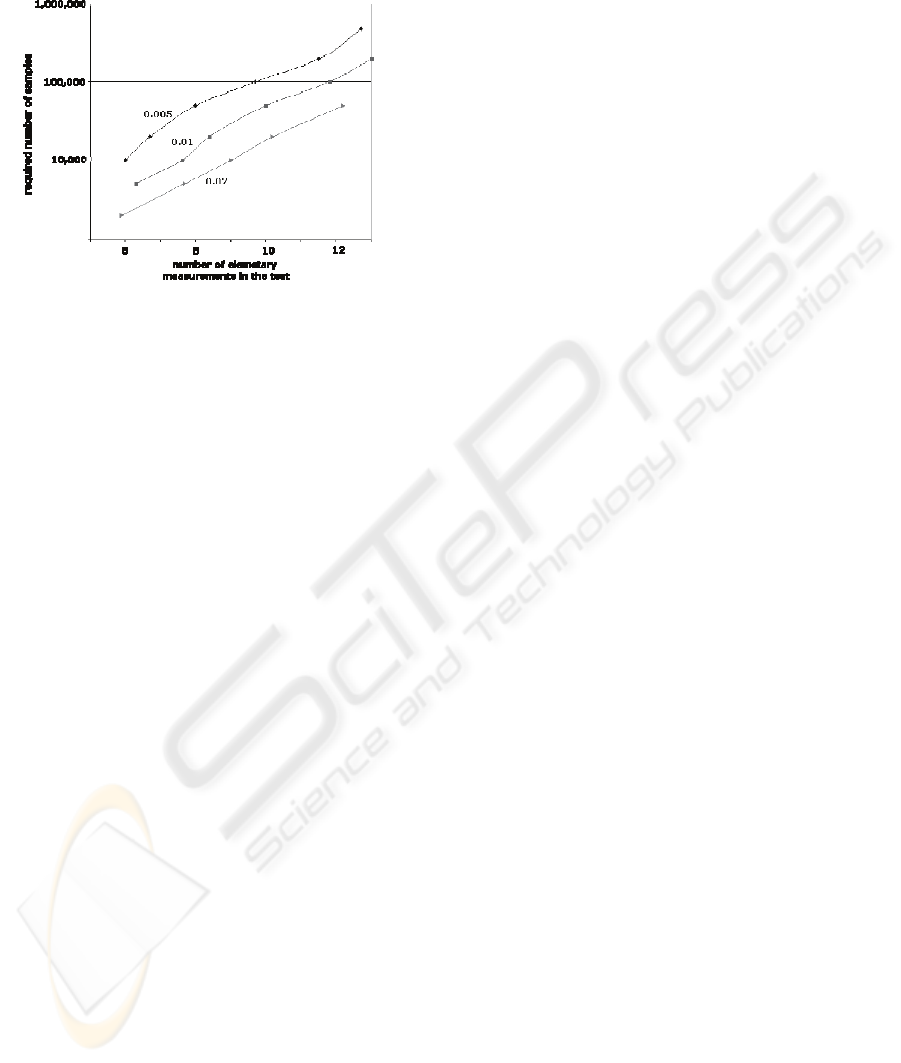
standard error made in calculations will be
somewhere near 1%.
Figure 8: The appropriate number of samples depends on
used number of elementary measurements in the tests and
on chosen error rate.
Figure 8 is indicating a minimum number of
samples one should use depending on sizes of
defined groups in the ontology and on chosen error
rate. E.g., if one is satisfied with error rate of 1% and
has up to 12 elementary measurements in a group
she should use at least 100,000 samples in reasoning
phase. Chart depicts such relation for error rates of
2%, 1% and 0.5%.
7 CONCLUSIONS
In this paper we have demonstrated the approach for
integration of knowledge from BNs and ontologies
in order to calculate the IG of structured medical
test. We strongly believe that the approach is sound
and can be very useful in practical medical decision
support systems.
The main obstacle in the described methodology
appeared to be the combinatorial nature of the
number of outcomes in grouped diagnostic tests.
However, practical experiments indicate that this
obstacle in some cases can be to some extent
avoided by usage of sampling algorithms in the
reasoning phase. The measurements have
demonstrated the dependency of error rate and
required number of samples. On that basis, and
considering some specific system properties such as
number of nodes in the network, sizes of grouped
diagnostic tests, acceptable time of reasoning,
acceptable error rate, and properties of machine that
performs reasoning, one can conclude which number
of samples should she use in the reasoning phase.
Structure of BNs inherently assumes conditional
independency – an assumption that in general case
does not stand for medical diagnostic tests. In spite
of that, vast majority of decision support systems
that make use of BNs ignore this issue. However,
one should be fully aware of this drawback when
using proposed methodology in practice.
Suggested methodology of integration of BNs
and ontologies still calls for more thorough testing
of its overall performance and has yet to
demonstrate its practical utility in real medical
environments. Furthermore, suitability of the
approach in some other domains remains to be
shown. All above mentioned problems seem to be
rather interesting topics for the future work.
REFERENCES
Pan, R., Ding, Z., Yu, Y., Peng, Y. (2005). A Bayesian
Network Approach to Ontology Mapping. Fourth
international Semantic Web Conf. 2005, 563-577.
Devitt, A., Danev, B., Matusikova, K. (2006).
Constructing Bayesian Networks Automatically using
Ontologies. Ontology Meet Industry workshop 2006.
Town, C. (2004). Ontology-driven Bayesian Networks for
Dynamic Scene Understanding. IEEE Computer
Society Conference on Computer Vision and Pattern
Recognition Workshops 2004.
McGarry, K., Garfield, S., Wermter, S. (2007). Auto-
Extraction, Representation and Integration of a
diabetes Ontology using Bayesian Network.
Computer-Based Med. Sys. Symposium ’07, 612-617
Huhns, M.N., Valtorta, M.G. (2007). Ontological Support
for Bayesian Evidence Management. Ontology for the
intelligence community 2007, 47-51.
Wang, W., Zeng, G., Zhang, D., Huang, Y. et al. (2008).
An intelligent Ontology and Bayesian Network based
Semantic Mashup for Tourism. IEEE Congress on
Services, part I, 128-135.
Jeon, B.J., Ko, I.Y. (2007). Ontology-Based Semi-
Automatic Construction of Bayesian Network Models
for Diagnosing Diseases in E-health Applications.
Frontiers in the Convergence of Bioscience and
Information Technologies, fbit, pp.595-602
Zheng, H.T., Kang, B.Y., Kim, H.G. (2005). An
Ontology-based Bayesian Network Approach for
Representing Uncertainty in Clinical Practice
Guidelines, Uncertainty Reasoning for the Semantic
Web workshop 2005.
Jagt, R.M. (2002). Support for multiple cause diagnosis
with Bayesian Networks. Thesis for degree of Master
of Science in Applied Mathematics. Information
Sciences department, University of Pittsburgh, USA.
HEALTHINF 2010 - International Conference on Health Informatics
240