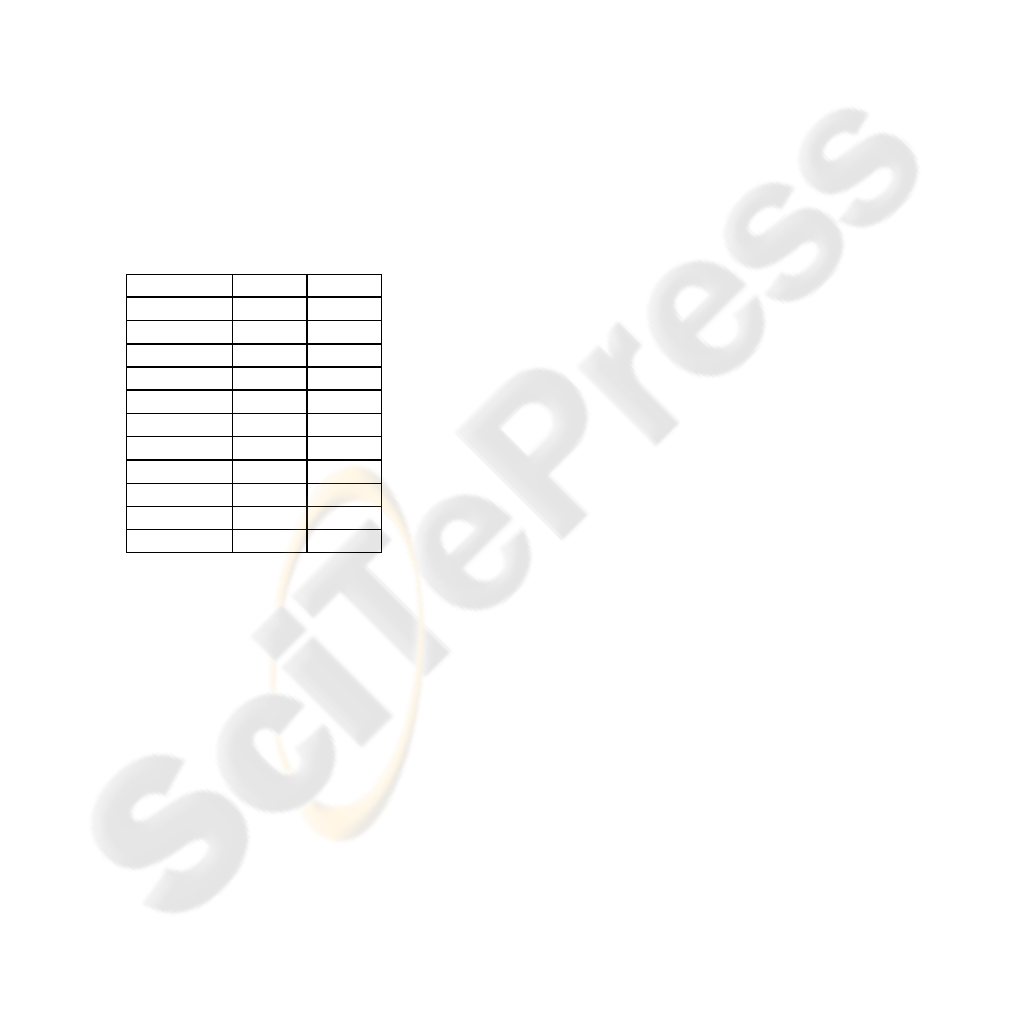
4.3 Experiments
In the experiments realized through this study, two
databases are used for testing the learning process.
The database used for learning contains 200 images
from different nature categories and is used to learn
the correlations between images and semantic
concepts. The database used in the learning process
is categorized into 50 semantic concepts. The system
learns each concept by submitting appreciatively 20
images per category. The testing database contains
500 unclassified images
The performance metrics, precision and average
normalized modified retrieval rate (ANMRR), are
computed to evaluate the efficiency and accuracy of
the rules generation and annotation methods
(Manjunath et al., 2001). These parameters are
computed as average for each image category as in
Table 1:
Table 1: The precision and ANMRR computed for each
image category.
As it can be observed from the experiments, the
results are strongly influenced by the complexity of
each image category. Actually, the results of
experiments are very promising, because they show
a small average normalized modified retrieval rate
and a good precision for the majority of the database
categories, making the system more reliable.
5 CONCLUSIONS
In this study we propose methods for semantic
image annotation based on visual content.
By comparison to other image annotation methods,
our proposed and developed methods have some
advantages:
The entire process is automated, and a great
number of semantic concepts can be defined.
These methods can be easily extended to any
domain, because the visual features, semantic
indicators remain unchanged, and the semantic
rules are generated based on the set of example
labelled images used for learning semantic
concepts.
The spatial information is taken into account
and it offers rich semantic information about the
relationships of the image colour regions (left,
right, center, bottom, and upper).
The Prolog logic programming used to model
images and semantic rules facilitates the
interaction with them in a easier way.
The proposed methods have the limitation that
they can’t learn every semantic concept, due to the
fact that the segmentation algorithm is not capable to
segment images in real objects. Improvements can
be brought using a segmentation method with
greater semantic accuracy.
REFERENCES
Berson, A., Smith, S.J., 1997. Data Warehousing, Data
Mining, and OLAP, McGraw-Hill. New York.
Carneiro, G., Chan, A., Moreno, P., and Vasconcelos, N.,
2007. Supervised learning of semantic classes for
image annotation and retrieval. In IEEE Pattern
Analysis Machine Intelligence, vol. 29(3), pp. 394–
410.
Hoogs, A., Rittscher, J., Stein, G., and Schmiederer, J.,
2003. Video content annotation using visual analysis
and a large semantic knowledge base. In Proceedings
of the IEEE Computer Society Conference on
Computer Vision and Pattern Recognition, pp. 327 –
334.
Frawley, W. J., G. Piatetsky-Shapiro, and C. J. Matheus,
1991. Knowledge Discovery in Databases, chapter
Knowledge Discovery in Databases: An Overview.
MIT Press.
Manjunath, B. S, Salembier, P., and Sikora, T., 2001.
Introduction to MPEG-7: Multimedia Content
Description Standard. Wiley, New York.
Rasiwasia, N., Moreno, P. J., Vasconcelos, N., 2007.
Bridging the Gap: Query by Semantic Example. In
IEEE Transactions On Multimedia, vol. 9(5), pp. 923-
938.
Smith, J. R. and S.-F. Chang, 1996. VisualSEEk: a fully
automated content-based image query system. The
Fourth ACM International Multimedia Conference and
Exhibition, Boston, MA, USA.
Smeulders, A. W., M. Worring, S. Santini, A. Gupta, and
R. Jain, 2000. Content-Based Image Retrieval at the
End of the Early Years. In IEEE Trans. Pattern
Analysis and Machine Intelligence, vol. 22(12), pp.
1349–1380.
RULE BASED MODELLING OF IMAGES SEMANTIC CONCEPTS
543