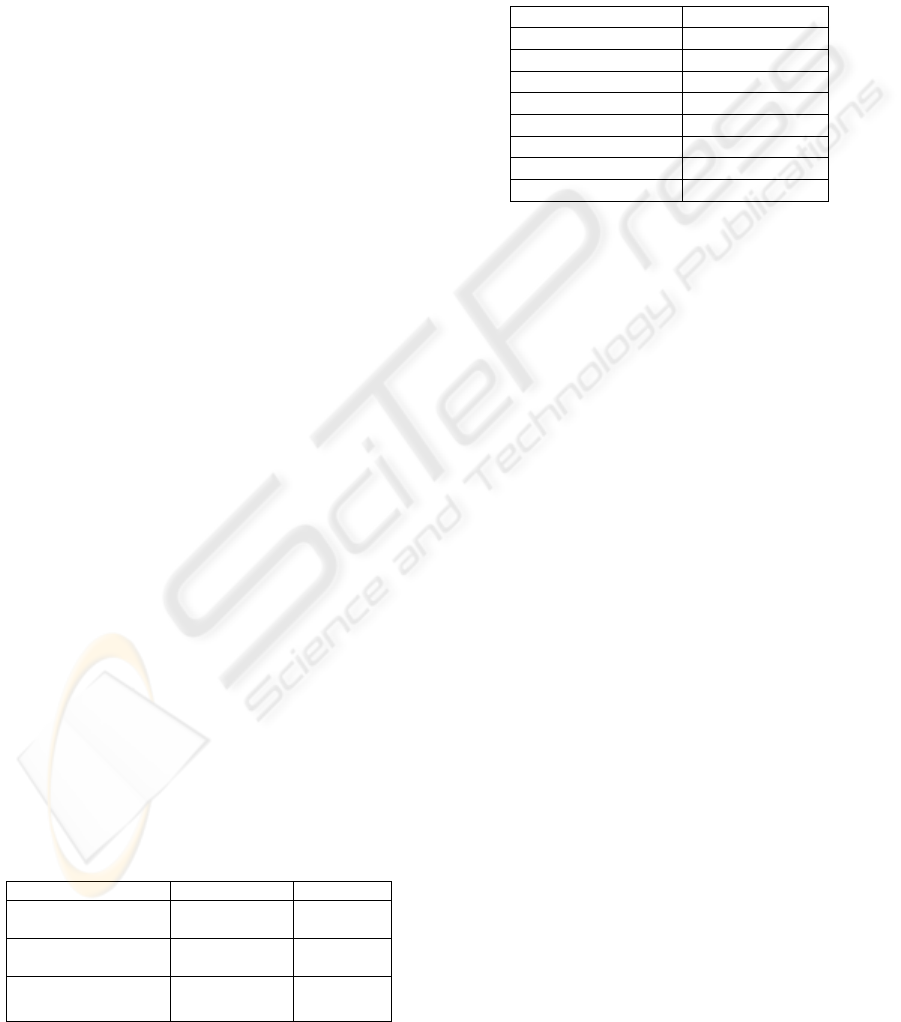
selection procedure does not allow identifying the
best class separation. When the tumour size and the
number of infected lymph nodes are added to these
10 selected features, an increase of 4% is achieved
in both cases but it remains under the 86% obtained
with the 32 features. In the case of PCA, the results
given in Table 2 seem better. Nevertheless, even if
the overall rate of prediction is more than 83%, the
prediction of relapse (poor prognosis) is very low
(36.36%).
3.2 Ljubljana Prognosis Dataset
For roughly 30% of the patients who undergo an
operation on breast cancer, the disease reappears
after five years. Regarding this dataset, the aim is to
predict whether patients are likely to relapse, which
may influence the treatment they will receive.
The Ljubljana Prognosis dataset contains a total of
286 patients for whom 201 have not relapsed after
five years and 85 who have relapsed (Clark &
Niblett, 1987). For these patients, 9 features are
available (six qualitative and three interval types):
1. Age: 10-19, 20-29, 30-39, 40-49, 50-59, 60-69,
70-79, 80-89, 90-99
2. Menopause: >40, <40, pre-menopause.
3. tumour size: 0-4, 5-9, 10-14, 15-19, 20-24,
25-29, 30-34, 35-39, 40-44, 45-49, 50-54, 55-59.
4. invaded nodes: 0-2, 3-5, 6-8, 9-11, 12-14, 15-17,
18-20, 21-23, 24-26, 27-29, 30-32, 33-35, 36-39.
5. Ablation ganglia: yes, no.
6. malignancy Degree: I, II, III
7. Breast right, left
8. Quadrant: sup. left, inf. left sup. right, inf. right,
center.
9. Irradiation: yes, no
3.2.1 Methods and Results
A cross-validation (50% training, 50% test) has been
performed to estimate the accuracy of the proposed
methodology. Patients with missing data were
excluded from this analysis (9 patients). The results
are given in Table 3. In order to compare these
results with those cited in earlier works (Clark &
Niblett, 1987), a first study consisted in classifying
Table 3: LAMDA results with Ljubljana dataset.
Feature selection Training Test
Whole original
dataset
91% 89.89%
8 features with
interval grade
91.33% 90%
Without irradiated
patients
93% 92.1%
the 277 patients with 9 features as given in the
original dataset: 6 qualitative features including the
degree of malignancy (feature No. 6 given by
modalities I, II or III) and 3 interval features. A
second study was done by treating the grade data as
intervals (I: [3,5], II: [6,7], III: [8,9]). This allows
expressing the linguistic distance between grades,
such as oncologists do naturally.
Table 4: Ljubljana comparative results.
Method Accuracy
MEPAR-miner 92.8%
LAMDA 90%
Isotonic separation 80%
EXPLORE 76.5%
C4.5 72%
AQR 72%
Assist 86 68%
NaiveBayes 65%
The results obtained by considering the grade
type as an interval show the effectiveness of this
method, which gives an accuracy of 91.33% in
training and 90% in test. Figure 6, 7 and 8 show the
class parameters of interval features obtained in
these two studies. It can be observed that the interval
features “Tumour size” and “Lymph nodes” are
more discriminatory between classes in the two
studies than the “Age” feature. This fact was
established in many previous studies (Deepa et al.,
2005), where it was noted that these two features
still to date are considered as important prognostic
factors. While for the feature “Grade” which makes
the difference between the two studies, even if in the
first study (Figure 7, where it was considered as
qualitative) the difference in the three modalities
frequencies between the two classes can be
observed, the interpretation is still quite ambiguous
since the two classes contains the three grades with a
slight difference. In the second study (Figure 8,
when the grade is considered as interval feature) the
interpretation becomes easier and straightforward.
A third part of the study was to consider only
patients who have not yet undergone an irradiation
treatment (215). This treatment had been applied
systematically to patients with a positive number of
lymph nodes. This implies that the two features:
“irradiation” and the “number of affected lymph
nodes” are correlated with each other. The objective
here is to validate the method precisely to help
physicians on the decision of treatment based on the
results of prognosis beyond 5 years. The results (3rd
line of Table 3) are quite satisfactory, 93% of
accuracy for learning and 92.1% for test. Comparing
these results (Table 4) with those obtained with
BIOINFORMATICS 2010 - International Conference on Bioinformatics
128