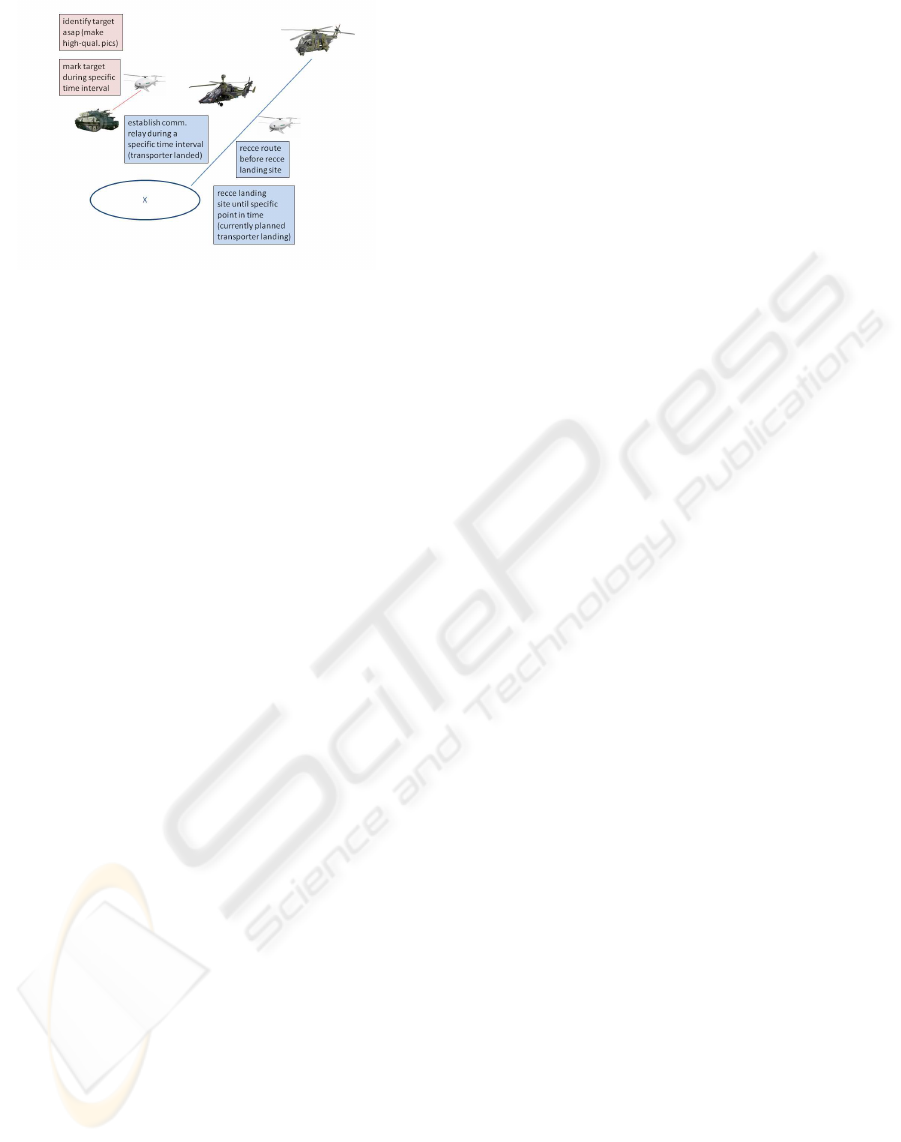
Figure 10: Manned-unmanned teaming use case.
is able to work as communications relay in the given
time frame. In case the temporal constraints cannot
be satisfied, it may be an option to re-plan the whole
mission, e.g. slow down the manned helicopters so
that the constraints become loosened.
4.2 Future Work
To solve such a problem without too much human in-
teraction, the intelligent agent needs to predict the dy-
namic development of the situation. Furthermore, the
agent needs to keep track of time constraints, consum-
able and renewable resources. As already mentioned,
this will require more elaborate planning algorithms,
potentially operating directly on the working memory
graph. Interesting improvements could come from
features of recent AI planners, e.g. strong and soft
constraints and preferences, that were introducedwith
PDDL3 (Gerevini and Long, 2005).
As furthermore mentioned in section 2.5, another
current research activity focuses on the control policy
between reactive and deliberative layer. Finding this
right balance of flexibility is a major question in the
agent community - other hybrid agent architectures
have already introduced sophisticated (but sometimes
for our purposes too complex) concepts.
REFERENCES
Billings, C. E. (1997). Aviation Automation - the Search
for a Human-Centered Approach. Lawrence Erlbaum
Associates, Mahwah, NJ.
Endsley, M. R. and Garland, D. J. (2000). Situation Aware-
ness Analysis and Measurement. Lawrence Erlbaum
Associates, Mahwah, NJ.
Ferguson, I. A. (1992). TouringMachines: An Architecture
for Dynamic, Rational, Mobile Agents. PhD thesis,
University of Cambridge. Clare Hall.
Fikes, R. and Nilsson, N. (1971). Strips: A new approach
to the application of theorem proving to problem solv-
ing. In Artificial Intelligence, volume 2, pages 198–
208, Heidelberg, Germany. Springer.
Freed, M., Bonasso, P., Ingham, M., Kortenkamp, D., Pell,
B., and Penix, J. (2005). Trusted autonomy for space-
flight systems. In AIAA First Space Exploration Con-
ference, Orlando, FL.
Gerevini, A. and Long, D. (2005). Plan constraints and pref-
erences in PDDL3. Technical report, Dipartimento di
Elettronica per l’Automazione, Universita degli Studi
di Brescia.
Laird, J. E., Newell, A., and Rosenbloom, P. S. (1987).
SOAR: An architecture for general intelligence. In
Artificial Intelligence, volume 33, pages 1–64, Hei-
delberg, Germany. Springer.
Matzner, A., Minas, M., and Schulte, A. (2008). Efficient
graph matching with application to cognitive automa-
tion. In Applications of Graph Transformations with
Industrial Relevance, pages 297–312, Berlin, Ger-
many. Springer.
Meitinger, C. and Schulte, A. (2009). Human-uav co-
operation based on artificial cognition. In Engineer-
ing Psychology and Cognitive Ergonomics, pages 91–
100, Heidelberg, Germany. Springer.
Onken, R. and Schulte, A. (2009). System-ergonomic
Design of Cognitive Automation in Work Systems.
Springer, Heidelberg, Germany.
Rasmussen, J. (1983). Skills, rules and knowledge, signals,
signs and symbols, and other distinctions in human
performance models. In IEEE Transactions on Sys-
tems, Man, and Cybernetics, volume SMC-13, pages
257–266, Heidelberg, Germany. Springer.
Rauschert, A., Meitinger, C., and Schulte, A. (2008). Ex-
perimentally discovered operator assistance needs in
the guidance of cognitive and cooperative uavs. In
Proceedings of HUMOUS conference, Brest, France.
Springer.
Schulte, A., Meitinger, C., and Onken, R. (2008). Human
factors in the guidance of uninhabited vehicles: Oxy-
moron or tautology? the potential of cognitive and
co-operative automation. In International Journal on
Cognition Technology & Work, Heidelberg, Germany.
Springer.
Uhrmann, J., Strenzke, R., Rauschert, A., C.Meitinger, and
Schulte, A. (2009). Manned-unmanned-teaming: Ar-
tificial cognition applied to multiple uav guidance. In
NATO RTO SCI Symposium on Intelligent Uninhab-
ited Vehicle Guidance Systems, Neubiberg, Germany.
ICAART 2010 - 2nd International Conference on Agents and Artificial Intelligence
298