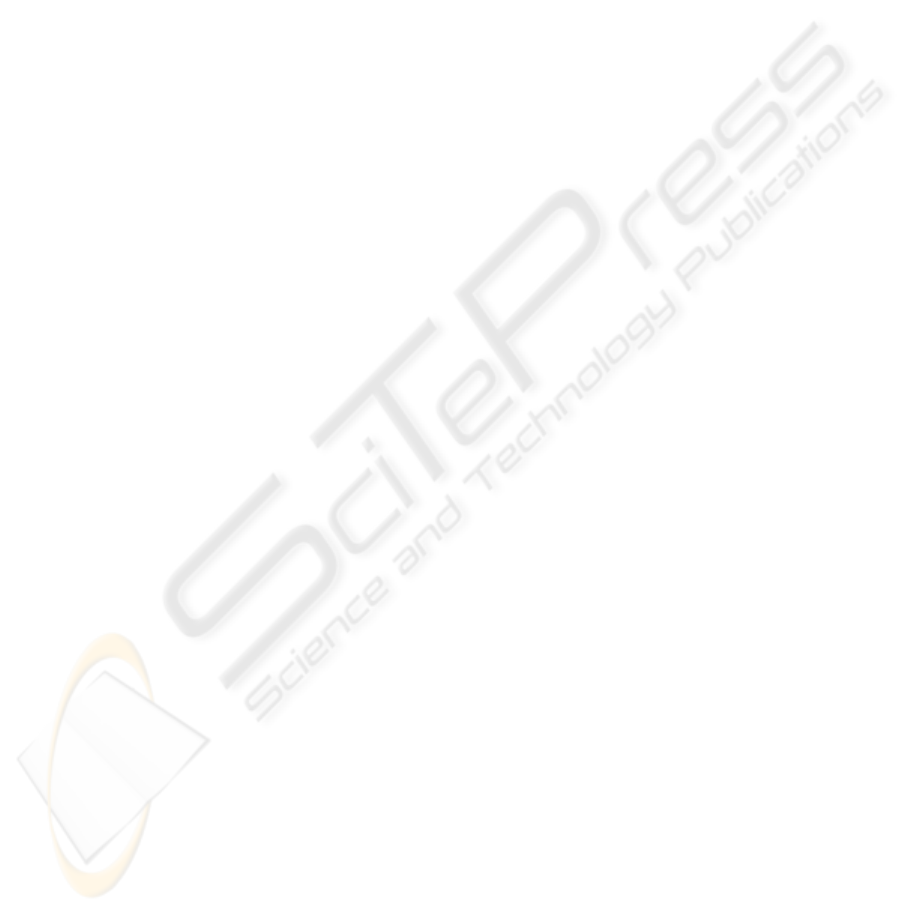
Even though Bayesian Networks give good
results compared to the current existing techniques,
there is still the possibility to improve them. A new
network is been adjusted. The first idea is that the
network will be able to adjust its probabilities faced
with the arrival of new data after some flights.
6 CONCLUSIONS
Conditional View function is an important task for
operational support and operational risk assessment
for aerospace industry. This function involves many
types of aircraft components (brake wear, electronic
components, actuators, fuel pump, etc) to be
evaluated.
Because of the fact that is not possible to achieve
this task with an only type of technique it is
necessary to look for different techniques which
involve many issues that lead to the need to cope
with uncertainties, and the need to re-assess and
adapt initial models.
Bayesian Networks are useful technologies.
Even tough most of the efforts so far have been
focused on diagnosis for Bayesian Networks, this
paper demonstrates the usage concerning prognosis,
in particular for the Conditional View in order to
improve aircraft maintenance with a new type of
decision support.
ACKNOWLEDGEMENTS
The authors gratefully acknowledge the support of
the European Commission Sixth Framework
program for Research and Technological
Development. This paper summarizes work
performed as part of FP6 project TATEM
"techniques and technologies for new maintenance
concepts" (Integrated Project AIP3-CT-2004-
502909).
The authors also acknowledge the support of
Brian Bbell and Bert Bullen of BAE Systems for
their support on the provision of data for the Brake
Wear use case.
REFERENCES
Byington, C.S, Roemer, M.J, Galie, T., 2002. “Prognostic
enhancements to diagnostic systems for improved
condition-based maintenance”. Aerospace Conference
Proceedings. IEEE Publication. Vol 6, 2815-2824.
Díez, F. J., 2000. “Introduction to Approximate
Reasoning”. In Spanish. PhD Course, UNED, Madrid.
Gilabert, E., Arnaiz, A., 2006. “Intelligent automation
systems for predictive maintenance. A case study”.
Robotics and Computer Intergrated Manufacturing
(RCIM). Vol22 numbers 5-6. pp543-549.
Dietterich, T., 1999. “Learning in Graphical Models”.
First MIT Press edition.
Neapolitan, R.E., 2004. “Learning Bayesian Networks”.
Pearson Prentice Hall.
Goode, K.B., Roylance, B., 1999. “Predicting the Time to
Failure of Critical Components- A Software Package
Strategy”. Proc. Condition Monitoring and diagnostic
engineering management (COMADEM) 99 pp. 547-
555.
Onisko, A., Druzdzel, M. J., Wasyluk, H., 1998. “A
probabilistic Causal Model for Diagnosis of Liver
Disorders”. Intelligent Information Systems VII.
Proceedings of the Workshop held in Malbork,
Poland, June 15-19.
Sierra, B., Larrañaga, P., 1998. “Predicting survival in
malignant skin melanoma using Bayesian Networks
automatically induced by genetic algorithms. An
empirical comparison between different approaches.”
Artificial intelligence in Medicine, 14:215-230.
Lazkano, E., Sierra, B., Astigarraga, A., Martínez-Otzeta,
J.M., 2007. “On the use of Bayesian Networks to
develop behaviours for mobile robots” Robotics and
Autonomus Systems 55 (2007) 253-265.
Ibargüengoytia, P. H., Vadera, S., Sucar, L. E., 2006. “A
Probabilistic Model for Information and Sensor
Validation”. The Computer Journal 2006 49(1):113-
126.
Doguc, O., Ramirez-Marquez, J. E., 2009. “A generic
method for estimating system reliability using
Bayesian networks”. Reliability Engineering and
System Safety 94 (2009) 542-550.
Oukhellou, L., Come, E., Bouillaut, L., Aknin, P., 2008.
“Combined use of sensor data and structural
knowledge processed by Bayesian network:
Application to a railway diagnosis aid scheme”.
Transportatino Research Part C 16 (2008) 755-767.
Barco, R., Díez, L., Wille, V., Lázaro, P., 2009.
“Automatic diagnosis of mobile communication
networks under imprecise parameters”. Expert
Systems with Applications 36 (2009) 489-500.
IMPROVING AIRCRAFT MAINTENANCE WITH INNOVATIVE PROGNOSTICS AND HEALTH MANAGEMENT
TECHNIQUES - Case of Study: Brake Wear Degradation
575