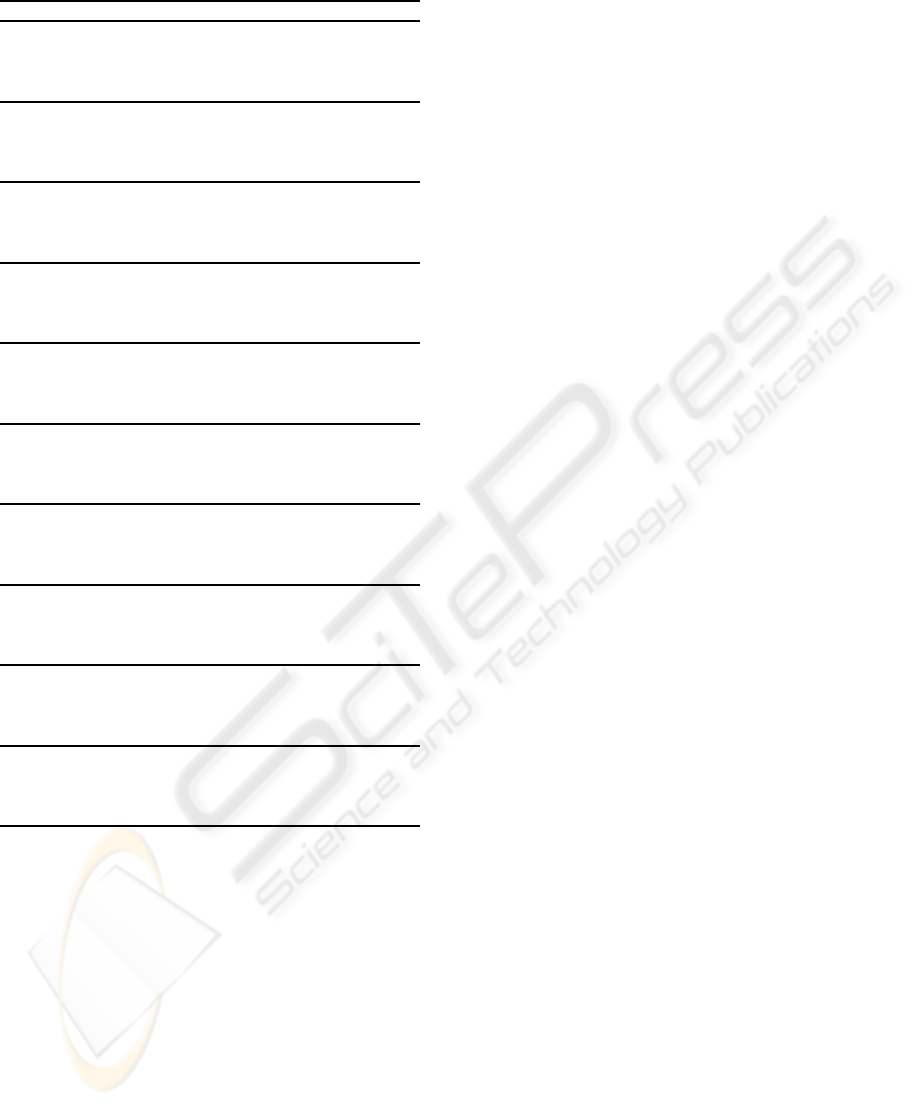
Table 4: Emotional targets found in the middle Z-score set.
Range of P
pos.
/ Sample of emotional targets
90% – 100%/ company, foreign exchange, accident,
lunch, newest information, image, coffin, knowledge,
going to sleep, customer, cover, lottery, Yakisoba(fried
noodles), new mail, salary
80% – 90%/ rest, musical, garlic, house, animation,
bumber ticket, ambulance car, dam, soft cream, ticket,
actual place, plan, Mr. Aso, Soumen(fine noodles), 3 days,
revision, high school basebale tournament
70% – 80%/ chance, Gyoza(pot sticker), Obon
(Japanese Summer holidays), clothes, reference book,
sheep, access counts, proposal, boyfriend/girlfriend, son,
once, Japanese people, stairs, guys, past questions, movies
60% – 70%/ cloud, characteristics, senior, process,
short, street stall, milk, potato, world view, flower langu-
age, high school days, panel, course, room, Mr. Kouichi,
response, joy, park, Tokyo, tombstone, TVCM, RPG
50% – 60%/ helmet, boys, Japanese, lecture, environ-
ment problem, curren, strain (feeling), water place, safe
management, game, Yankees, vanilla, category, climbing
Mt. Fuji, Beawanpi(one-piece dress), expected software,
40% – 50%/ fear, triathlon, batted ball, immediately
after, sitting comfort, Osaka Touin(school), young people,
ear, mood, Shun(person), dark, whole life, love, future,
meal time, driving, competition, ultraviolet rays
30% – 40%/ obligation, corner, leukemia, prejudice,
under construction, terrorist, feeling of intimacy, Hikari
(light, fiber-optic cable), natural environment, belly,
member on the regular payroll, symptoms, oneself
20% – 30%/ abnormal weather, contents of work,
darkness, otherwise, fatigue, narrow, crowdedness, life, SAP
for maker, love, elegant, property management, shooting
(film), a liitle happy, aphthous ulcer, drawback, husband
10% – 20%/ HIV, bewilderment, muddy, allow, noon,
twice or more, provery of blood, marriage, put away, 30,
your body, a hip joint, load (of baggage), shame, headache,
next election of Presentative, scarcity of water, hallucination
0% – 10%/ Furafura(dizzily), Japan High School
Baseball Federation, molar tooth, panic, a chief secretary-
Aso Taro, continuation, breast cancer, camp, sleeplessness,
respect, strained back, ant, sleeping posture
* Extracted from the interval Aug. 1st - 7th 2008. Trans-
lated into English by the author of this paper.
6 CONCLUSIONS
This paper proposed an affective blog analyzer
(ABLANA) which crawls blog articles along a time
series from the Web and analyzes people’s emotional
targets. The method for emotion reasoning uses a sen-
tence pattern dictionary. The original dictionary is
A-Japanese-Lexicon. It covers Japanese fundamental
6,000 verbs and consists of 14,800 patterns. In this
paper, the extended dictionary is used, where emo-
tional information is annotated if pattern expresses
emotional processes (arousal, state and response).
In the experiments, the extracted emotional tar-
gets can be filtered and sorted by two parameters,
for instance, the Z-score in terms of the frequency of
the keyword appearance and the probability of emo-
tions. These parameters are so effective that trendy
and emotional targets can be captured. Thus, the do-
main independent affective analyzer is successfully
constructed. It is expected to practically apply this
technique to capture people’s affective statements.
REFERENCES
Elliott, C. (1992). The Affective Reasoner: A process model
of emotions in a multi-agent system. PhD thesis,
Northwestern University.
Hatzivassiloglou, V. and McKeown, K. R. (1997). Predict-
ing the semantic orientation of adjectives. In Pro-
ceedings of the Annual Meeting of the Association for
Computational Linguistics, pages 174–181.
Ikehara, S., Miyazaki, M., Shirai, S., Yokoo, A., Nakaiwa,
H., Ogura, K., Ooyama, Y., and Hayashi, Y. (1997).
Goi-Taikei: A Japanese Lexicon. Iwanami Shoten.
Kanayama, H. and Nasukawa, T. (2008). Textual demand
analysis: Detection of users’ wants and needs from
opinions. In Proceedings of the International Confer-
ence on Computational Linguistics, pages 409–416.
Liu, H., Liberman, H., and Selker, T. (2003). A model of
textual affect sensing using real-world knowledge. In
Proceeding of the International Conference on Intelli-
gent User Interfaces, pages 125–132.
Ortony, A., Clore, G. L., and Collins, A. (1988). The Cog-
nitive Structure of Emotions. Cambridge Univ. Press.
Tokuhisa, M. and Okada, N. (1997). A conceptual analy-
sis of emotional words for an intellectual, emotional
agent. In Proc. of the Int. Conf. on Pacific Association
for Computational Linguistics, pages 307–315.
Tokuhisa, R., Inui, K., and Matsumoto, Y. (2008). Emotion
classification using massive examples extracted from
the web. In Proceedings of the International Confer-
ence on Computational Linguistics, pages 881–888.
Turney, P. (2002). Thumbs up or thumbs down? seman-
tic orientation applied to unsupervised classification
of reviews. In Proc. of the Ann. Meeting of the Associ-
ation for Computational Linguistics, pages 417–424.
ICAART 2010 - 2nd International Conference on Agents and Artificial Intelligence
252