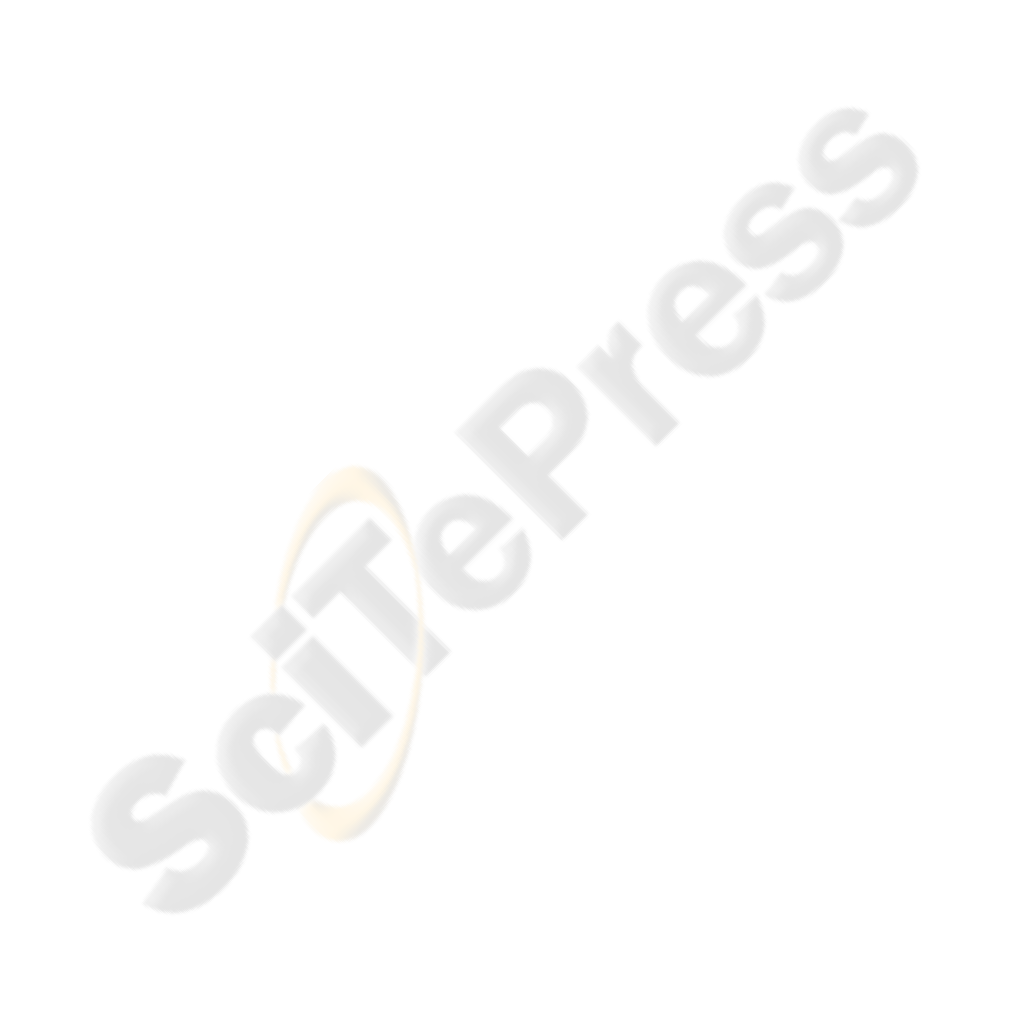
3.2 Determination of Carotenoid
Concentrations in Foods
Lycopene and β-carotene chemicals belong to the
carotenoids family. These are widespread in nature
being the main group of pigments with important
metabolic functions. Due to its antioxidant activity,
these chemicals show a strong correlation between
carotenoid intake and a reduced risk of some
diseases, such as cancer, atherogenesis, bone
calcification, eye degeneration, neuronal damages
etc. Due to their characteristics of solubility and
instability, the analytical methods for measuring
carotenoids in vegetables are limited which makes
necessary a very careful handling process and a
short analysis time to avoid degradation and
isomerization. Because of this, a reliable and rapid
analysis method for carotenoid quantification in
vegetable products is required (Schoefs, 2002;
Bicanic et al., 2003).
Given that the lycopene and β-carotene are active in
the same region of UV-vis spectroscopy, their
determination by linear algorithms are not suitable
(Torrecilla et al., 2008). In order to use this fast,
simple analytical technique, a nonlinear algorithm
based on NN algorithm has been applied on the UV
absorbance data at 446 and 502 nm wavelengths.
Using these absorbance values of 25 binary mixtures
composed of lycopene and β-carotene with
concentration between 0.4-3.2 μg mL
-1
and their
respective concentration distributed following an
experimental design, the NN was optimized, Table
2. Once the NN model was optimized, NN/UV-Vis
spectroscopy was applied to determine the
concentration of both chemicals in food samples
such as tomato concentrate, tomato sauce, ketchup,
tomato juice and tomato puree. The mean prediction
error value was 1.5% and the correlation coefficient
was higher than 0.99. The mean prediction error is
fifty times lower than when a linear model is used in
place of non linear algorithms. This improvement in
the results is extremely valuable for its application to
a fast and reliable lycopene and β-carotene
evaluation in food samples without using complex
analytical methods.
3.3 Determination Polyphenolic
Compounds Concentrations
in Olive Oil Mill Wastewater
In the manufacture of extra virgin olive oil, waste is
produced and it has a serious environmental impact
due to its high content of organic substances (sugars,
tannins, polyphenols, polyalcohols, pectins and
lipids, etc.) It is known that caffeic acid (CA) and
catechol (CT) are two of the major contributors to
the toxicity of these wastes. Given their
electrochemical characteristics, laccase biosensor
(LB) is commonly used to determine CA and CT.
Because of the similarities in the produced oxidized
species, the amperometric signal overlapping in the
reduction voltammograms is high, and therefore, a
powerful tool is required to solve this signal.
Using voltammogram profiles of 300 samples and
their respective concentrations of caffeic acid and
cathecol, an NN was optimized. Once the NN model
was optimized, it was validated using real
concentration taken from three different olive oil
mills in Spain (Almendralejo, Badajoz; Martos,
Jaén; Villarejo de Salvanes, Madrid). The mean
prediction error (equation 4) was less than 0.5 % and
the correlation coefficient was higher than 0.999,
these statistical results are even better and more
selective than other non portable commercial
analytical equipment. Therefore, the integrated
NN/LB system is an adequate approach to estimate
both hazardous chemicals in olive oil mill
wastewater.
3.4 Determination of Glucose, Uric
and Ascorbic Acids in Biological
Mixtures
The major obstacle for the amperometric detection
of glucose in real samples is the interference arising
from electro oxidizale substances such as ascorbic
and uric acids existing in a measured system. Here,
an amperometric biosensor based on a colloidal gold
- cysteamine - gold disk electrode with an enzyme
glucose oxidase and a redox mediator,
tetrathiafluvalene, co-immobilized atop the modified
electrode, was used for the simultaneous
determination of glucose, ascorbic and uric acids, in
ternary mixtures. The concentrations of these
chemicals were between 0 and 1 mM.
As a consequence of an experimental design, 125
cyclic voltammograms of ternary mixtures and their
respective concentrations were used to optimize the
NN model, Table 2. Then, the optimized NN was
validated. The mean prediction error (equation 4)
was less than 1.74% and the correlation coefficient
was higher than 0.99. In the light of these results, the
NN model is able to solve the interferences between
glucose, ascorbic and uric acids without any
chemical pre-treatment.
NEURAL NETWORKS FOR THE MODELING OF CONCENTRATION OF CHEMICALS
583