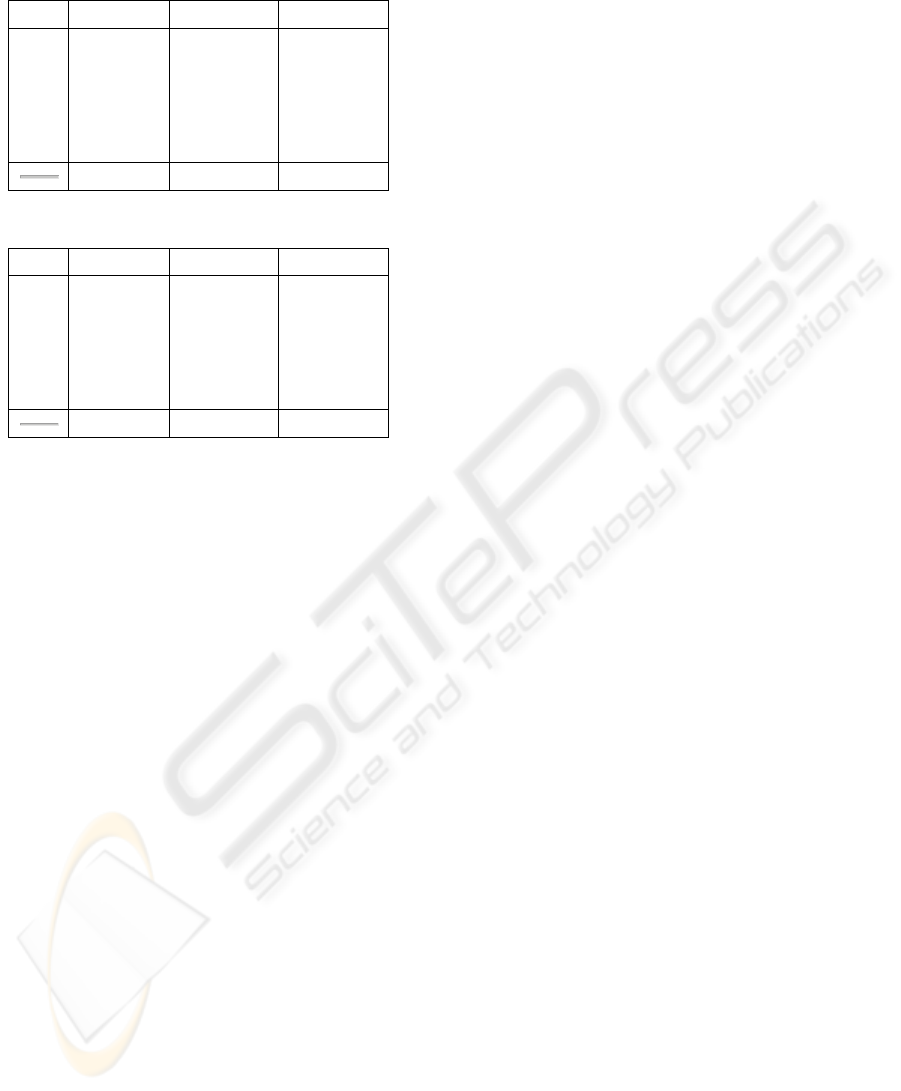
Table 1: Math vs. Nursery rhyme discrimination accuracy
results.
Subj. 61 elect. 19 elect. 10 elect.
1 75.8% 78.3% 61.5%
2 56.7% 63.3% 62.5%
3 59.2% 68.3% 53.3%
4 63.3% 73.3% 55.0%
5 73.3% 75.0% 70.8%
65.7% 71.7% 60.6%
Table 2: Left vs. Right discrimination accuracy results.
Subj. 61 elect. 19 elect. 10 elect.
1 72.5% 90.0% 75.0%
2 55.8% 76.7% 51.7%
3 55.8% 65.0% 50.8%
4 45.0% 58.3% 63.3%
5 53.4% 73.3% 63.3%
56.5% 72.6% 60.8%
For each subject (denoted with a number, for the
take of privacy), the mean values of results were
computed for two different sessions, considered
separately. No mixing of data was allowed from
different subjects, or from different sessions for the
same subject, as the results appear very different.
The accuracy in the case of usage of all the 61
electrodes is shown in the first column of the tables:
for some subject, as subject 1, it appears very high,
while it can be extremely low for some other
subjects. For instance, for subject 4 in table 2, it is
less than 50%: in this case, it could mean that,
paradoxically, a random selection between the two
choices would have given better results.
In the second column, the accuracy in the case of
19 electrodes is shown. As discussed above, an
accurate selection of best electrodes was done, in
function of the cortical areas mainly involved in the
four tasks of interest. Best results were carried out in
this case, obtaining accuracies over 70%. An error of
about 27% - 28% can be considered quite low,
accounting for the difficulty involved in the
experiment of interest: indeed, in every case, the
subject was required not to move any muscle, but
just to think of moving it. By the way, if a limb is or
is going to be really moved, the electrical activity in
the brain would become much more clear and could
be easily detected, as is shown in (Blankertz, 2006).
The accuracy in the cases of 10 electrodes is
shown in the third column. Presently, the number of
electrodes taken into account appears not sufficient
to get to good results. In particular, results appear
not useful for the discrimination between
mathematical operation and nursery rhyme, since the
selected electrodes are all around C
3
and C
4
, which
are mainly related to hand movements.
6 CONCLUSIONS
A classification method for brain-computer interface
is presented, which was able to discriminate among
different kind of mental tasks performed by a
subject. The method is based on a SVM classifier,
trained by the power frequency spectrum of EEG
signals coming from 61 electrodes set in the head
surface.
The experimental tests proved quite useful
results in case of 19 electrodes, while poor results
were obtained for 61 electrodes. This occurence is
likely to depend from the small number of trials, as
SVM method always requires a high number of
them, accounting for the large number of features to
be considered. In addition, large accuracy disparity
was found in the cases of different subjects: for
instance, in the case of 19 electrodes, accuracy up to
90% was obtained with subject 1, but just a little
over 58% with subject 4.
The results appear quite interesting compared
with other similar works, as in (Schogl, 2005), in
which different methods of classification are
considered. It was also shown SVM method to get
the best result, with accuracy average of about 63%.
The essential rules of the electrode number and
position are here pointed out, as they can
dramatically affect the classifier performance.
Future developments will include the time
domain analysis, in addition to the frequency
domain here examinated. It could be also interesting
to investigate the effect of data artefacts. They can
arise, for example, if the subject sometime can blink,
and this can produce noise in the EEG, getting worse
the performance of the classifier. Significant
improvements could be carried out cleaning the data
from this kind of noise.
REFERENCES
Huan N. J. and Palaniappan R., 2004. Neural network
classification of autoregressive features from
electroencephalogram signals for brain–computer
interface design. In Journal of Neural Engineering
vol. 1, 142-150.
Wolpaw J. R., Birbaumer N. McFarland D. J, Pfurtscheller
G. and Vaughan T. M. 2002. Brain-computer
SVM EVALUATION FOR BRAIN COMPUTER INTERFACE SYSTEMS
243