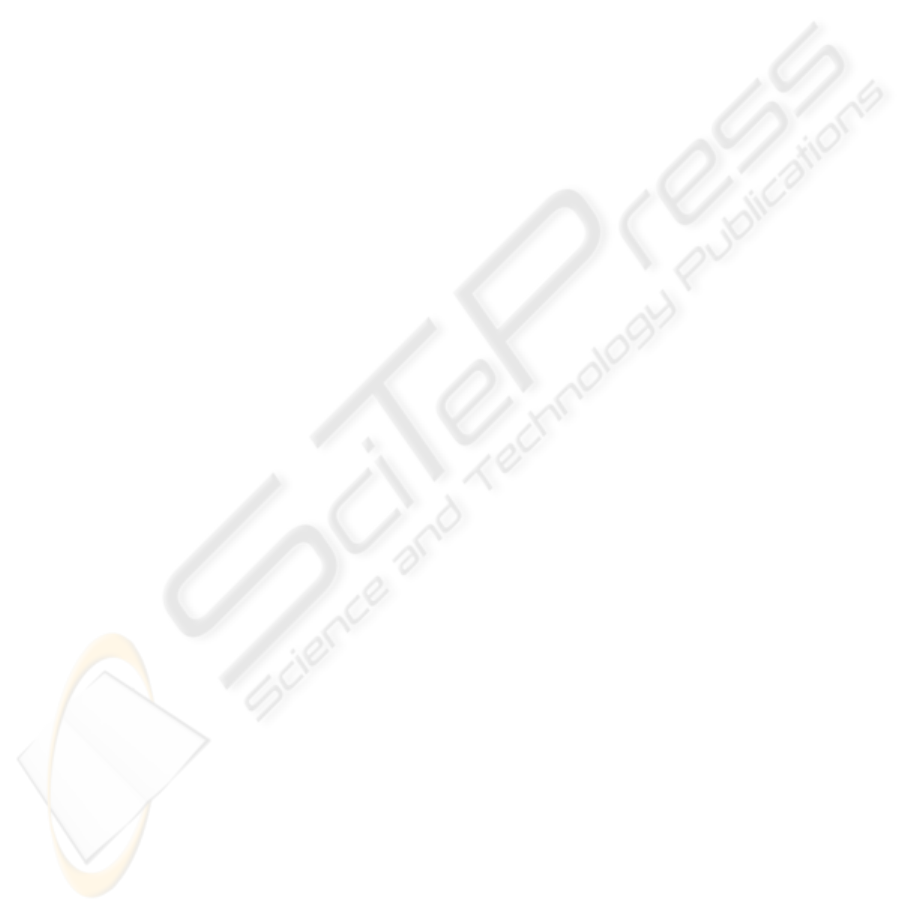
sification scheme as very useful in the classification of
ischemic and HR related transient ST-episodes. They
indicate the possibility of a future online application
which allows the usage of the method in monitoring
devices.
REFERENCES
Blanchett, T., Kember, G. C., and Fenton, G. A. (1998). Klt-
based quality controlled compression of single-lead
ecg. IEEE Trans Biomed Eng, 45(7):942–945.
Castells, F., Laguna, P., Sornmo, L., Bollmann, A., and
Millet-Roig, J. (2007). Principal component analysis
in ecg signal processing. EURASIP Journal on Ad-
vances in Signal Processing, 1:1–21.
Dranca, L., Goni, A., and Illarramendi, A. (2006). Us-
ing decisiontrees for real-time ischemia detection. In
CBMS, pages 719–726.
Exarchos, T. P., Papaloukas, C., Fotiadis, D. I., and
Michalis, L. K. (2006). An association rule mining-
based methodology for automated detection of is-
chemic ecg beats. IEEE Trans Biomed Eng,
53(8):1531–1540.
Faganeli, J. and Jager, F. (2008). Automatic distinguishing
between ischemic and heart-rate related transient st
segment episodes in ambulatory ecg records. In CinC,
pages 381–384.
Goldberger, A. L., Amaral, L. A., Glass, L., Hausdorff,
J. M., Ivanov, P. C., Mark, R. G., Mietus, J. E., Moody,
G. B., Peng, C. K., and Stanley, H. E. (2000). Phys-
iobank, physiotoolkit, and physionet: components of
a new research resource for complex physiologic sig-
nals. Circulation, 101(23):E215–E220.
Jager, F. (2006). Advanced Methods And Tools for ECG
Data Analysis, Chapter 9: Introduction to Feature Ex-
traction, pages 245–264. Artech House.
Jager, F., Moody, G. B., and Mark, R. G. (1998). Detec-
tion of transient st segment episodes during ambula-
tory ecg monitoring. Comput Biomed Res, 31(5):305–
322.
Jager, F., Taddei, A., Moody, G. B., Emdin, M., Antolic, G.,
Dorn, R., Smrdel, A., Marchesi, C., and Mark, R. G.
(2003). Long-term st database: a reference for the de-
velopment and evaluation of automated ischaemia de-
tectors and for the study of the dynamics of myocar-
dial ischaemia. Med Biol Eng Comput, 41(2):172–
182.
Kohavi, R. (1995). A study of Cross-Validation and Boot-
strap for Accuracy Estimation and Model Selection.
In IJCAI, pages 1137–1145.
Laguna, P., Moody, G. B., Garca, J., Goldberger, A. L., and
Mark, R. G. (1999). Analysis of the st-t complex of
the electrocardiogram using the karhunen–love trans-
form: adaptive monitoring and alternans detection.
Med Biol Eng Comput, 37(2):175–189.
Langley, P., Bowers, E., Wild, J., Drinnan, M., Allen, J.,
Sims, A., Brown, N., and Murray, A. (2003). An al-
gorithm to distinguish ischaemic and non-ischaemic st
changes in the holter ecg. In CinC, pages 239–242.
Maglaveras, N., Stamkopoulos, T., Diamantaras, K., Pap-
pas, C., and Strintzis, M. (1998). ECG pattern recog-
nition and classification using non-linear transforma-
tions and neural networks: a review. Int J Med Inform,
52:191–208.
Minchole, A., Jager, F., and Laguna, P. (2007). Discrimina-
tion between demand and supply ischemia episodes in
holter recordings. In Conf Proc IEEE Eng Med Biol
Soc, volume 2007, pages 2579–2582.
Minchole, A., Skarp, B., Jager, F., and Laguna, P. (2005).
Evaluation of a root mean squared based ischemia de-
tector on the long-term st database with body position
change cancellation. In CinC, pages 853–856.
Moody, G. and Jager, F. (2003). Distinguishing is-
chemic from non-ischemic st changes: the phys-
ionet/computers in cardiology challenge 2003. In
CinC, pages 235–237.
Moody, G. and Marc, R. (1982). Development and evalua-
tion of a 2-lead ecg analysis program. In CinC, pages
39–44.
Papadimitriou, S., Mavroudi, S., Vladutu, L., and Beze-
rianos, A. (2001). Ischemia detection with a self-
organizing map supplemented by supervised learning.
IEEE Trans Neural Netw, 12(3):503–515.
Papaloukas, C., Fotiadis, D. I., Likas, A., Stroumbis, C. S.,
and Michalis, L. K. (2002). Use of a novel rule-based
expert system in the detection of changes in the st seg-
ment and the t wave in long duration ecgs. J Electro-
cardiol, 35(1):27–34.
Rumelhart, D., Hintont, G., and Williams, R. (1986). Learn-
ing representations by back-propagating errors. Na-
ture, 323:533–536.
Smrdel, A. and Jager, F. (2004). Automated detection of
transient st-segment episodes in 24 h electrocardio-
grams. Med Biol Eng Comput, 42(3):303–311.
Smrdel, A. and Jager, F. (2008). An algorithm to esti-
mate the st segment level in 24-hour ambulatory ecg
records. In CinC, pages 701–704.
Stadler, R. W., Lu, S. N., Nelson, S. D., and Stylos,
L. (2001). A real-time st-segment monitoring algo-
rithm for implantable devices. J Electrocardiol, 34
Suppl:119–126.
Taddei, A., Costantino, G., Silipo, R., Emdin, M., and
Marchesi, C. (1995). A system for the detection of
ischemic episodes in ambulatory ecg. In CinC, pages
705–708.
Thakor, N. V., Webster, J. G., and Tompkins, W. J. (1984).
Estimation of QRS complex power spectra for design
of a QRS filter. IEEE Trans Biomed Eng, 31(11):702–
706.
Xing, W., Liang, X., Zhongwei, S., Zibin, Y., and Yi, P.
(2007). Heart rate variability analysis of ischemic and
heart rate related st-segment deviation episodes based
on time-frequency method. In NFSI-ICFBI, pages
162–164.
Zimmerman, M., Povinelli, R., Johnson, M., and Ropella,
K. (2003). A reconstructed phase space approach for
distinguishing ischemic from non-ischemic st changes
using holter ecg data. In CinC, pages 243–246.
DISCRIMINATION BETWEEN ISCHEMIC AND HEART-RATE RELATED ST-EPISODES - Non-linear Classification
for an Online Capable Approach
251