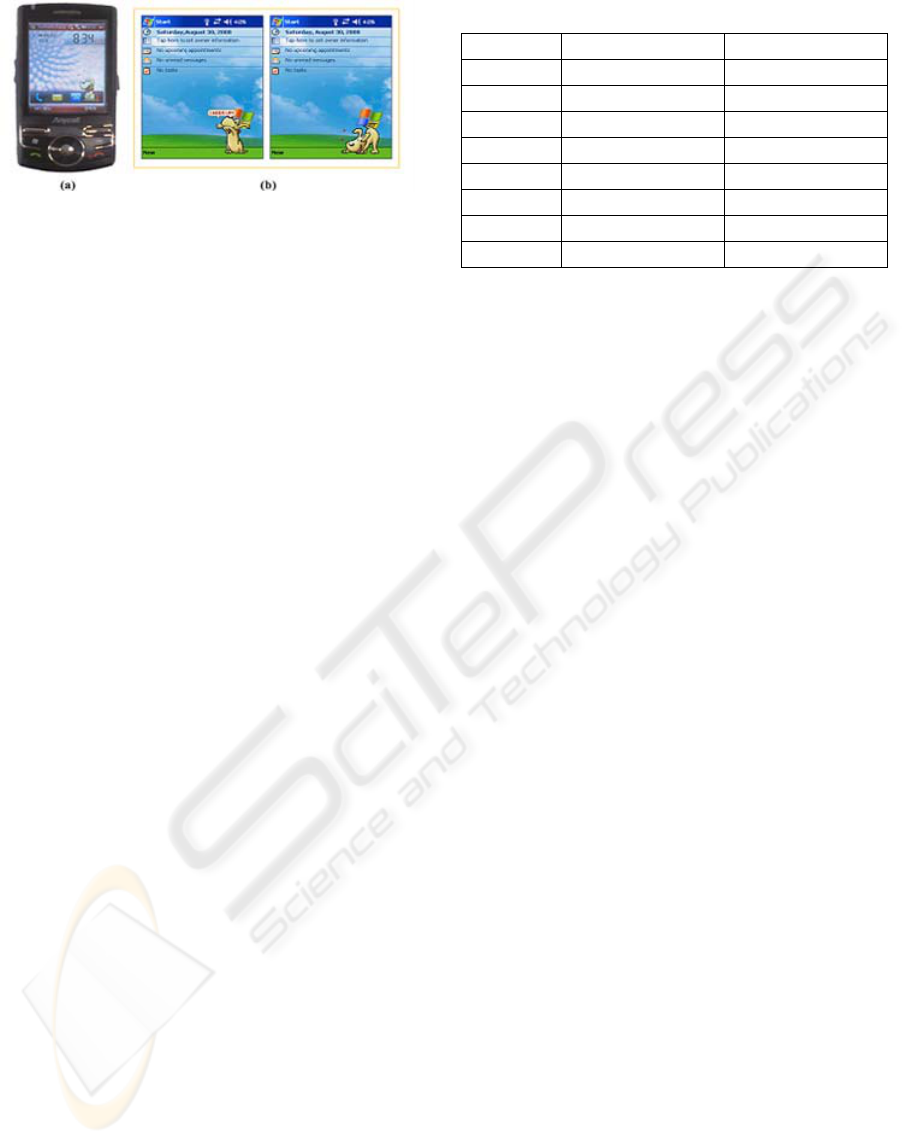
Figure 6: Screen shots of an intelligent synthetic character
on the actual smartphone(a) and the simulator(b).
The participants observed a sequence of five
behaviors for each scenario generated randomly and
another sequence of 5 behaviors generated by the
proposed method, respectively. The random
generation method just selects any behaviors
randomly to the user without understanding
situations
After the previous task, the participants
evaluated the fitness of each behavior on each
situation. The fitness ranged from 1 which means
"Strongly incongruent" to 5 which means "Strongly
suitable." Following the evaluations, we summed up
the fitness scores of each participant distinguishing
two different behaviors generating methods,
randomly generating method and the proposed
method, and measured the average fitness scores of
each method. Table 1 shows the average fitness
scores evaluated by the participants.
In order to analyze the result of the usability test,
we conducted the Wilcoxon signed-rank test with
the fitness scores. As the result of the Wilcoxon
signed-rank test, we got P-value by 0.012, thereby
we accept the alternative hypothesis because P-value
is smaller than 0.05. It confirms that the proposed
method is appropriate to generate the character's
behaviors.
6 CONCLUDING REMARKS
We presented the architecture of the mobile
intelligent synthetic character for its natural
behaviors. In order to provide enhanced intelligent
services, it is necessary for the intelligent synthetic
character to interact with the user and evolve by
itself. To achieve this, we will attempt to develop
algorithms for an interaction and an evolution,
especially the learning system that evolves the
structure of the Bayesian networks and the behavior
generation network with the user’s feedback in the
future work.
Table 1: Average fitness scores.
Participants Random generation Proposed method
1 2.46 4.50
2 2.40 4.22
3 3.00 4.44
4 2.14 4.38
5 2.44 4.40
6 3.12 4.44
7 2.88 4.54
8 2.06 4.20
ACKNOWLEDGEMENTS
This work was supported by the IT R&D program of
MKE/KEIT (10033807, Development of context
awareness based on self learning for multiple
sensors cooperation).
REFERENCES
Y.-D. Kim, Y.-J. Kim, J.-H. Kim and J.-R. Lim,
“Implementation of artificial creature based on
interactive learning,” In Proc. of 2002 FIRA Robot
World Congress, pp. 369-373, 2002.
S. Schiaffino and A. Amandi, “On the design of a software
secretary,” In Proc. of the Argentine Symp. on
Artificial Intelligence, pp. 218-230, 2002.
Y.-D. Kim, J.-H. Kim and Y.-J. Kim, “Behavior
generation and learning for synthetic character,”
Evolutionary Computation, vol. 1, pp. 898-903, 2004.
I. Marsa-Maestre, M. A. Lopez-Carmona, J. R. Velasco
and A. Navarro, “Mobile agent for service
personalization in smart environments,” Journal of
Networks, vol. 3, no. 5, pp. 30-41, 2008.
B.-K. Sung, “An intelligent agent for inferring user status
on smartphone,” Journal of KIIT, vol. 6, no. 1, pp. 57-
63, 2008.
R. Picard, “Affective Computing,” Media Laboratory
Perceptual Computing TR 321, MIT Media
Laboratory, 1995.
C. Bartneck, “Integrating the OCC model of emotions in
embodied characters,” In Proc. of the Workshop on
Virtual Conversational Characters: Applications,
Methods, and Research Challenges, 2002.
A. Ortony, G. Clore, and A. Collins, The Cognitive
Structure of Emotions, Cambridge University Press,
Cambridge, UK, 1988.
D.-W. Lee, H.-S. Kim and H.-G. Lee, “Research trend on
emotional communication robot,” Journal of Korea
Information Science Society, vol. 26, no. 4, pp. 65-72,
2008.
P. Maes, “How to do the right thing,” Connection Science
Journal, vol. 1, no. 3, pp. 291-323, 1989.
ICAART 2010 - 2nd International Conference on Agents and Artificial Intelligence
318