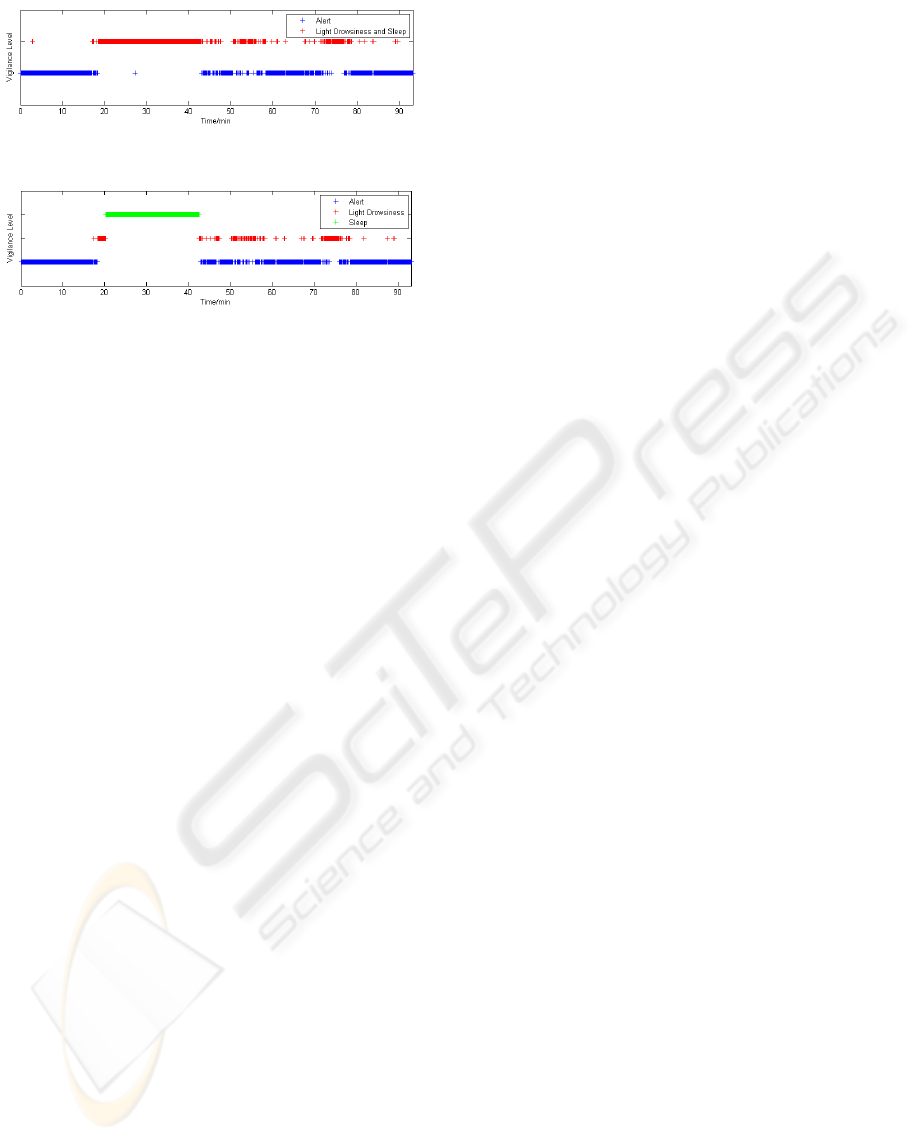
Figure 2: Classification of two vigilance states.
Figure 3: Classification of three vigilance states including
sleep state.
4 CONCLUSIONS
In this paper, an EEG signal processing method is
presented for distinguishing ’light drowsiness’ from
other vigilance level in driving simulation environ-
ment. Firstly, we extract 4 features for each frequency
band in every EEG channel. Then we use a mutual
information based feature selection to reduce the di-
mension of features. Finally, SVM is used to classify
light drowsiness state from alert on labeled EEG data.
Our experiment results give over 91% average accu-
racy with 5s time resolution for five subjects. This
study also shows that the light drowsiness state can
be classified very precisely from alert state. Accord-
ing to the result of this classification, accidents caused
by driver sleep can be prevented efficiently.
ACKNOWLEDGEMENTS
This work was supported by the National High Tech-
nology Research and Development Program of China
(No.2008AA02Z310). The authors also would like to
thank Prof. Bao-Liang Lu and other researchers in his
laboratory for their helpful work on EEG data acqui-
sition.
REFERENCES
Boser, B. E., Guyon, I. M., and Vapnik, V. N. (1992). A
training algorithm for optimal margin classifiers. In
Proceedings of the fifth annual workshop on Compu-
tational learning theory (pp.144-152). ACM.
Cortes, C. and Vapnik, V. (1995). Support-vector net-
works. In Machine Learning 1995 (vol.20,pp.273-
297). Springer.
Doughty, M. J. (2002). Further assessment of gender-
and blink pattern-related differences in the sponta-
neous eyeblink activity in primary gaze in young
adult humans. In Optometry and Vision Science
(vol.79,pp.439-447). Williams Wilkins.
Hori, T., Hayashi, M., and Morikawa, T. (1994). To-
pographical eeg changes and the hypnagogic experi-
ence. In Sleep onset: Normal and abnormal processes
(pp.237-253). American Psychological Association.
Lal, S. K. and Craig, A. (2001). A critical review of the
psychophysiology of driver fatigue. In Biological Psy-
chology (vol.55,pp.173-194). Elsevier.
Li, M., Fu, J.-W., and Lu, B.-L. (2008). Estimating vig-
ilance in driving simulation using probabilistic pca.
In Engineering in Medicine and Biology Society 2008
(pp.5000-5003). IEEE.
Lin, C.-T., Ko, L.-W., Chung, I.-F., Huang, T.-Y., Chen,
Y.-C., Jung, T.-P., and Liang, S.-F. (2006). Adaptive
eeg-based alertness estimation system by using ica-
based fuzzy neural networks. In IEEE Transactions
on circuits and systems (vol.53,pp.2469-2476). IEEE.
Makeig, S., Jung, T.-P., and Sejnowski, T. J. (1996). Us-
ing feedforward neural networks to monitor alertness
from changes in eeg correlation and coherence. In
Advances in Neural Information Processing Systems
(pp.931-937). MIT Press.
Niedermeyer, E. and Silva, F. L. D. (2004). Electroen-
cephalography: basic principles, clinical applica-
tions, and related fields (pp.194-209). Lippincott
Williams & Wilkins.
Noachtar, S., Binnie, S., Ebersole, C., Mauguiere, J.,
Sakamoto, F., Westmoreland, A., and Westmoreland,
B. (2004). A glossary of terms most commonly used
by clinical electroencephalographers and proposal for
the report form for the eeg findings. In Klinische Neu-
rophysiologie (vol.35,pp.5-21). George Thieme Ver-
lag.
Peng, H., Long, F., and Ding, C. (2005). Feature selec-
tion based on mutual information: Criteria of max-
dependency, max-relevance, and min-redundancy. In
IEEE Transactions on Pattern Analysis and machine
Intelligence (vol.27,pp.1226-1238). IEEE.
Schomer, D. L. (2007). The Clinical Neurophysiology
Primer (pp.57-71). Humana Press.
Shen, K.-Q., Ong, C.-J., Li, X.-P., Hui, Z., and Wilder-
Smith, E. P. V. (2007). A feature selection
method for multilevel mental fatigue eeg classifica-
tion. In IEEE Transactions on Biomedical Engineer-
ing (vol.54,pp.1231-1237). IEEE.
Shi, L.-C., Yu, H., and Lu, B.-L. (2007). Semi-supervised
clustering for vigilance analysis based on eeg. In Neu-
ral Networks 2007 (pp.1518-1523).
Weinger, M. B. (1999). Vigilance, boredom, and sleepi-
ness. In Journal of Clinical Monitoring and Comput-
ing (vol.15,pp.549-552). Kluwer.
Yeo, M. V., Li, X., Shen, K., and Wilder-Smith, E. P.
(2009). Can svm be used for automatic eeg detection
of drowsiness during car driving? In Safety Science
(vol.47,pp.115-124). Elsevier.
BIOINFORMATICS 2010 - International Conference on Bioinformatics
134