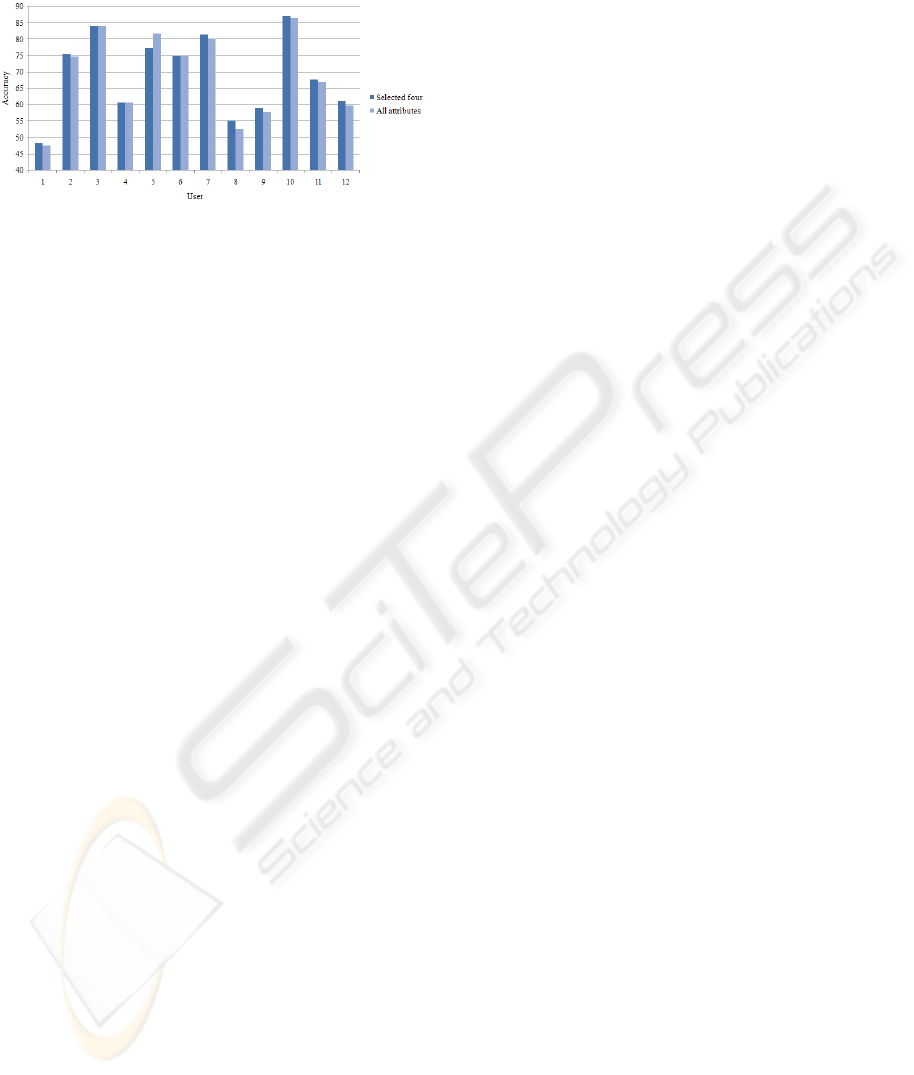
thought that the preprocessing part, which excludes
insignificant attributes, is effective.
Figure 6: Prediction accuracy comparison with one using
all attributes.
5 CONCLUDING REMARKS
This paper proposed the prediction method of user
activity in the sequence of mobile context for
ambient intelligence environment. We collected user
activity, place, time, day of week, call record, MP3,
SMS and photo as mobile context, and modeled the
patterns in the context sequence to predict the user’s
next activity. For better modelling, we used the
activity classification method in GSS and modified it
to college students, which provided context data in
this paper, and used the place classification method
in NHAPS. We selected four attributes of activity,
place, time and day of week among eight attributes
considering the significance, and learned dynamic
Bayesian network model with collected data. We
also made models both for individual users and all
users for new users. In experiments, we evaluated
the proposed prediction method with the collected
data, and confirmed the proposed method provided
good performance.
For future work, we are planning to cover two
more issues. One is user clustering and the other is
recommendation. To deal with general users’
context and activity patterns, user clustering is
required before prediction modeling. It is also useful
for recommender service from perspective of
marketing. After prediction, it will be interesting to
provide useful information to each user based on
predicted user activity. For example, the system can
recommend restaurant information if the model
predicts the following activity is restaurant meals
with friends. A service like this will make ambient
intelligence a smarter one.
ACKNOWLEDGEMENTS
This research was supported by Basic Science
Research Program through the National Research
Foundation of Korea (NRF) funded by the Ministry
of Education, Science and Technology (R01-2008-
000-20801-0)
REFERENCES
Cai, H., Hu, X., Lu, Q., Cao, Q., 2009. A novel intelligent
service selection algorithm and application for
ubiquitous web services environment. Expert Systems
with Applications. Elsevier.
Silva, G. C., Yamasaki, T., Aizawa, K., 2005. Evaluation
of video summarization for a large number of cameras
in ubiquitous home. ACM International Conference on
Multimedia.
Gemmell, J., Bell, G., Lueder, R., Drucker, S., Wong, C.,
2002. MyLifeBits: Fulfilling the Memex vision. ACM
International Conference on Multimedia.
Bellavista, P., Kupper, A., Helal, S., 2008. Location-based
services: Back to the future. IEEE Pervasive
Computing. IEEE.
Korpipaa, P., Mantyjarvi, J., Kela, J., Keranen, H., Malm,
E.-J., 2003. Managing context information in mobile
devices. IEEE Pervasive Computing. IEEE.
Cho, S.-B., Kim, K.-J., Hwang, K.-S., Song, I.-J., 2007.
AniDiary: Daily cartoon-style diary exploits Bayesian
networks. IEEE Pervasive Computing. IEEE.
Ermes, M., Parkka, J., Mantyjarvi, J., Korhonen, I., 2008.
Detection of daily activities and sports with wearable
sensors in controlled and uncontrolled conditions.
IEEE Transactions on Information Technology in
Biomedicine. IEEE.
Han., S.-J., Cho, S.-B., 2006. Learning trajectory
information with neural networks and the Markov
model to develop intelligent location-based services.
Journal of Information and Knowledge Management.
World Scientific.
Dey, A. K., 2001. Understanding and using context.
Personal and Ubiquitous Computing. Springer.
Kleiter, G. D., 1996. Propagating imprecise probabilities
in Bayesian networks. Artificial Intelligence. Elsevier.
Korpipaa, P., Koskinen, M., Peltola, J., Makela, S.-M.,
Seppanen, T., 2003. Bayesian approach to sensor-
based context awareness. Personal and Ubiquitous
Computing. Springer.
Hrovitz, E., Kadie, C. M., Paek, T., Hovel, D., 2003.
Models of attention in computing and
communications: From principles to applications.
Communications of the ACM. ACM.
Klepeis, N. E., et al., 2001. The national human activity
pattern survey (NHAPS): A resource for assessing
exposure to environmental pollutants. Journal of
Exposure Science and Environmental Epidemiology.
Nature Publishing Group.
Murphy, K., 2002. Dynamic Bayesian networks:
Representation, inference and learning. PhD Thesis.
University of California Berkley.
ICAART 2010 - 2nd International Conference on Agents and Artificial Intelligence
316