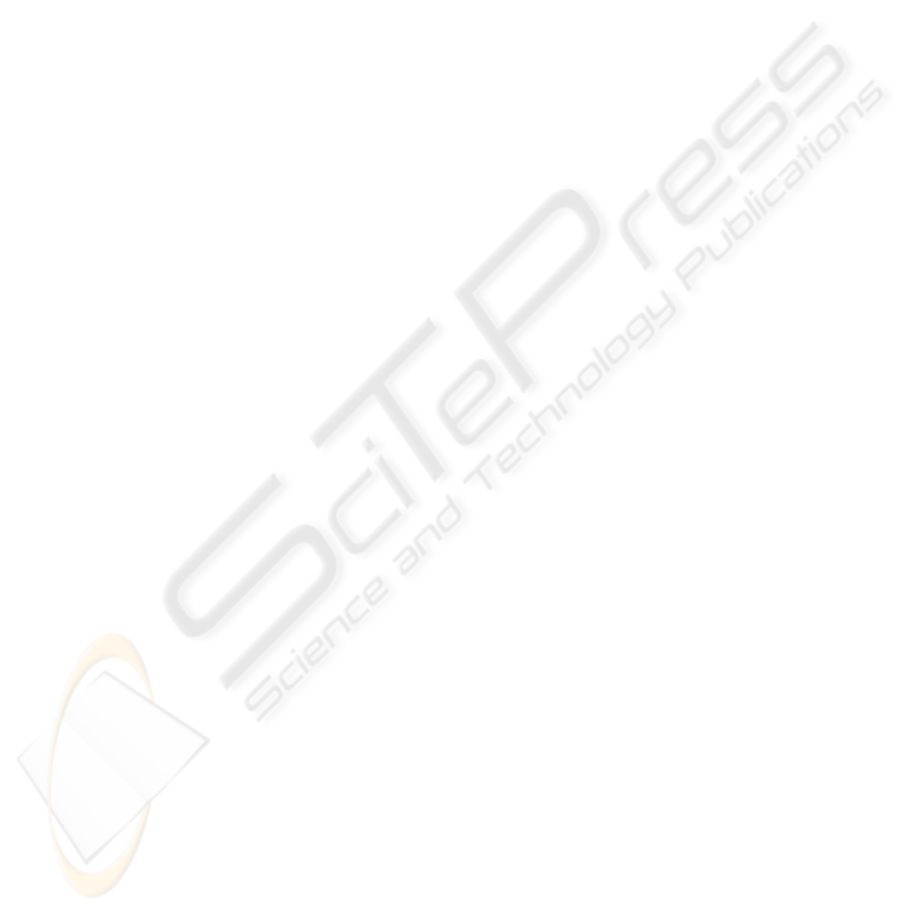
were: release date (20%), action (18.46%), comedy
(18.52%) and drama (27.65%) movie genres. The dis-
pensable movie attributes (0%) were animation, chil-
drens, crime, documentary, fantasy, film noir, musi-
cal, mystery, war and western movie genres.
4 CONCLUSIONS
This work shows the application of a genetic algo-
rithm for capturing user preferences in Recommender
Systems. More specifically, the genetic algorithm al-
lows setting an appropriate weight for each attribute
of a product or service, thus defining the preferences
of one user or a set of users over these attributes.
These obtained attribute weights permit to determine
which product or service attributes are more or less
valuables for users in their selection process when
buying products or paying for services.
All this ranking information given by the GA
could be employed by companies which want to of-
fer their clients those products which best fit their de-
sired attributes. Also, as it has been shown in this
paper, the obtained weights could be employed to pre-
dict user ratings for a test set of movies with a highly
good rate of right predictions. Moreover, the GA re-
sults could be used for offering good recommenda-
tions even when there is not enough informationof the
current user. In this way, the GA could be employed
for capturing the preferences of the users of the sys-
tem that are similar to the current user, and then for
applying the learned weights in order to provide suit-
able recommendations to the current user. This aspect
is especially useful when new users enter into a Rec-
ommender System, since the genetic approach per-
mits offering acceptable recommendations even when
few starting data for a user is available.
ACKNOWLEDGEMENTS
Thanks to GroupLens Research Group to allow the
use of MovieLens data for researching purposes.
This work is partially supported by CONSOLIDER-
INGENIO 2010 under grant CSD2007-00022 and
TIN2008-04446/TIN project, which is co-funded by
the Spanish government and FEDER funds.
REFERENCES
Goldberg, D. (1991). Real-coded genetic algorithms, virtual
alphabets, and blocking. Complex Systems, 5:139–
157.
Guan, S., Ngoo, C., and Zhu, F. (2002). Handy broker: an
intelligent product-brokering agent for m-commerce
applications with user preference tracking. Electron.
Commer. Res. Appl., 1(Issues 3-4):314–330.
Herlocker, J. L., Konstan, J. A., Borchers, A., and Riedl,
J. (1999). An algorithmic framework for performing
collaborative filtering. In ACM SIGIR ’99 Proceed-
ings, pages 230–237, New York, NY, USA. ACM.
Ortiz, D., Hervas, C., and Mu˜noz, J. (2001). Genetic algo-
rithm with crossover based on confidence interval as
an alternative to traditional nonlinear regression meth-
ods. In ESANN’2001 Proceedings, pages 193–198,
Bruges,Belgium.
Sarwar, B., Karypis, G., Konstan, J., and Riedl, J.
(2000). Analysis of recommendation algorithms for e-
commerce. In ACM EC ’00 Proceedings, pages 158–
167, New York, NY, USA. ACM Press.
Sarwar, B. M., Konstan, J. A., Borchers, A., Herlocker,
J., Miller, B., and Riedl, J. (1998). Using filtering
agents to improve prediction quality in the grouplens
research collaborative filtering system. In CSCW ’98
Proceedings, pages 345–354, New York, NY, USA.
ACM Press.
Shardnand, U. and Maes, P. (1995). Social information file-
tering: Algorithms for automating “word of mouth”.
In ACM CHI’95 Proceedings, pages 210–217.
Shibata, H., Hoshiai, T., Kubota, M., and Teramoto, M. (6-
7 Nov. 2002). Agent technology recommending per-
sonalized information and its evaluation. In 2nd In-
ternational Workshop on Autonomous Decentralized
System, 2002, pages 176–183.
Valero, S., Argente, E., Botti, V., Serra, J., and Corma, A.
(2004a). A soft computing technique applied to in-
dustrial catalysis. In ECAI2004 Proceedings, pages
765–769. IOS Press.
Valero, S., Argente, E., Botti, V., Serra, J., and Corma, A.
(2004b). Soft computing techniques applied to cat-
alytic reactions. LNAI, 3040:550–559.
Valero, S., Argente, E., Botti, V., Serra, J., Serna, P., Mo-
liner, M., and Corma, A. (2009). Doe framework for
catalyst development based on soft computing tech-
niques. Comput. Chem. Eng., 33:225–238.
ICAART 2010 - 2nd International Conference on Agents and Artificial Intelligence
602