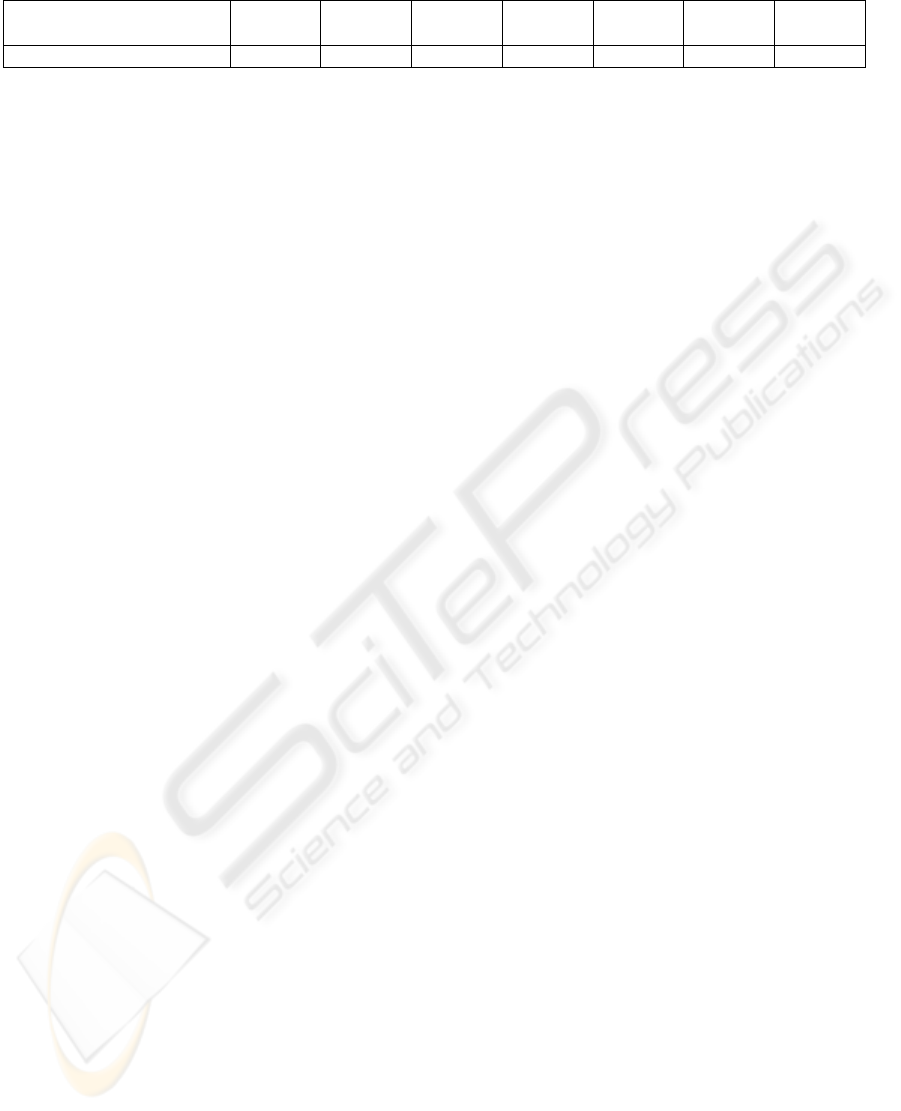
Table 3: The updated probability distribution of the priority level of B’s related goal.
The priority level of the
seller agent’s goals related
R1:
very low
R2:
low
R3:
lower
R4:
medium
R5:
higher
R6:
high
R7:
very high
Probability P
1
(Ri) 0 0 0 0.07 0.263 0.421 0.246
After the buyer agent respectively updated the
certainty level of the knowledge and the priority
level of the seller agent’s goal related to
1
, it will
evaluate the strength of the rest optional arguments
according to the updated values of the main
influencing factors, and thus choose to send a new
argument. The details can be found in (Guorui Jiang
et al., 2009). In the example, as an agent can not
allowed to send two same arguments continuously,
so
2
and 3
are the new optional arguments, we
finally select to send
2
in a new round after the
evaluation. We can find that it is precisely because
the priority level of the B-related goal "buy more
products" has been updated after the first round,
while this goal is also B’s related to
2
, allowing
2
be the better choice; also the choice is more
effective and scientific because of update of agent’s
belief on the dynamic negotiation environment.
6 CONCLUSIONS
In this paper, a Bayesian learning model has been
introduced along with Argumentation-based
negotiation, and the process of Bayesian learning for
argument selection has been analyzed with an
example. This could make more scientific and
rational choice of different types and contents of
arguments and increase the possibility of acceptance
of arguments. It gives an agent a certain ability to
learn by Bayesian learning model. So that it can be
continuously adjusted according to dynamic
environment, self-awareness, and effectively
improve the efficiency of argumentation. It
overcomes shortcomings of argument selection
followed a static environment in the previous study,
and appears to be more practical and effective.
But, in the model, more complete prior
knowledge is needed and the reasonableness and
accuracy of prior distribution also need to be further
improved. At the same time, more information from
the opponent and the negotiation environment
should be considered into the learning object of the
agent, to help it to greatly improve its self-adaptive
ability to the dynamic negotiation environment.
ACKNOWLEDGEMENTS
This work is supported by the National Natural
Foundation of China (No: 70940005).
REFERENCES
Amgoud, L., Cayrol, C., 2004. Generation and Evaluation
of Different Types of Arguments in Negotiation. In
Proceedings of International Workshop on Non-
Monotonic Reasoning (NMR'04), pp. 10-15.
Amgoud, L., Prade, H., 2005. Formal Handling of Threats
and Rewards in a Negotiation Dialogue. In
Proceedings of the 4th International joint Conference
on Autonomous Agents and Multi-Agent Systems,
AAMAS'2005, pp. 529-536.
Carabelea, C., 2002. Adaptive Agents in Argumentation-
based Negotiation. Multi-Agent Systems and
Applications. 2322, 87-122.
Guorui Jiang, Xiaoyu Hu, 2009. Research on the
Evaluation Model for Selection of the Argument’s
Type and Content in Argumentation-based
Negotiation of Agent. In Proceedings of the 11th
International Conference on Informatics and
Semiotics in Organisations, pp. 173-180.
Jinghua Wu, Guorui Jiang and Tiyun Huang, 2006. Using
Two Main Arguments in Agent Negotiation. Agent
Computing and Multi-Agent Systems. 4088, 578-583.
Kraus, S., Sycara, K., McBurney, P., and Evenchik, A.,
1998. Reaching Agreements through Argumentation.
Artificial Intelligence. 104(1-2), 1-69.
Nielsen, S. H., Parsons, S., 2007. An Application of
Formal Argumentation: Fusing Bayesian networks in
multi-agent systems. Artificial Intelligence. 171(10-
15), 754-775.
Rahwan, I., Ramchurn, S. D., McBurney, P., Parsons, S.,
and Sonenberg, L., 2003. Argumentation-based
Negotiation. The Knowledge Engineering Review.
18(4), 343-375.
Saha, S., Sen, S., 2004. A Bayes Net Approach to
Argumentation. In Proceedings of the 3rd
International Joint Conference on Autonomous Agents
and Multiagent Systems, pp. 1436-1437.
Saha, S. and Sen, S., 2005. A Bayes Net Approach to
Argumentation Based Negotiation. Artificial
Intelligence. 3366, 208-222.
Vreeswijk, G. A. W., 2005. Argumentation in Bayesian
belief networks. Artificial Intelligence. 3366, 111-129.
Zeng, D., Sycara, K., 1998. Bayesian learning in
negotiation. Human-Computer Studies. 48(1), 125-141.
ICAART 2010 - 2nd International Conference on Agents and Artificial Intelligence
322