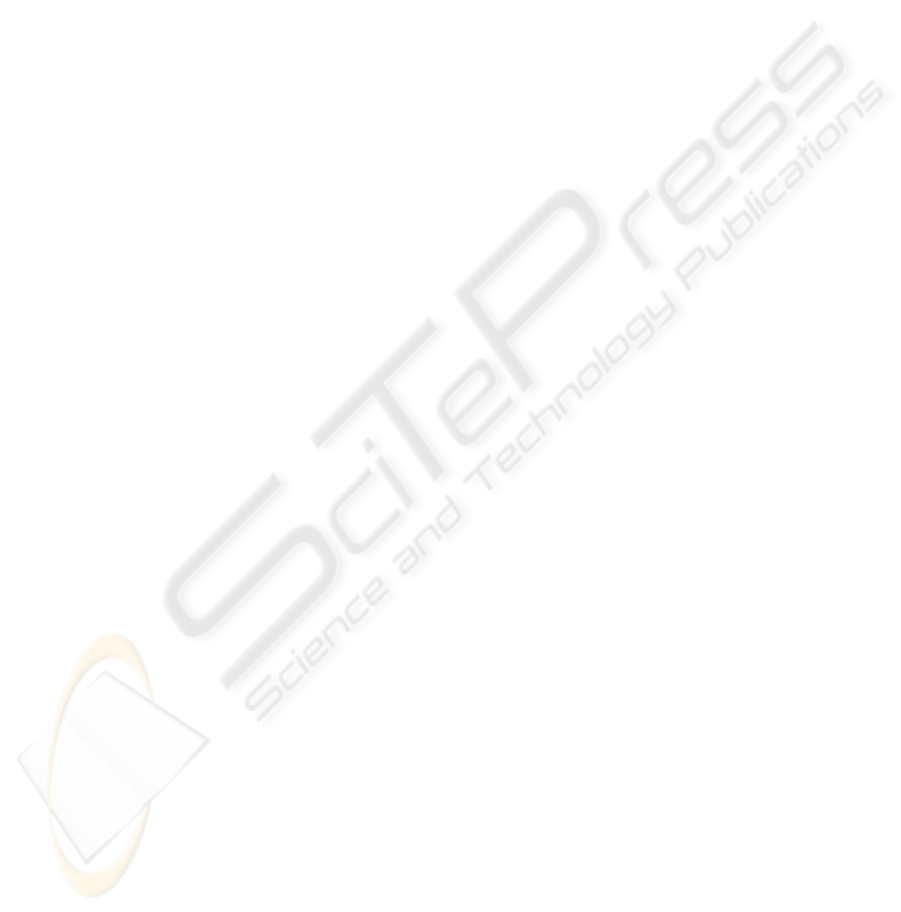
independent of the chip types and the normalization
methods too.
In the OMIM evaluation with both PCC and SRC
less than or equals to -0.5, five pairs of negative
correlated microRNA and its target genes matched
with OMIM records, in which four of them belong
to leukemia and the rest one is lung cancer. In these
five pairs, only one of them is an OCG, i.e.
MAP3K8. Besides, we also got five pairs of
significantly negative correlated microRNA and its
target in prostate cancer in which both of PCC and
SRC are under or equals to -0.5. Among these five
pairs, only one gene is a TSG, i.e. CDKN2A, and
only two genes are OCGs, i.e. CCND1 and
MAP3K8. These five pairs can be browsed in Table
5 in which they are denoted with italic and bold font.
Similar conclusions are obtained for the KEGG
evaluation.
Given that more than half of the probes’
correlation coefficients are negative correlated, we
identified certain putative pairs of microRNA and its
cancer related targets in different cancer types, such
as, hsa-mir-24-1:CDKN2A and hsa-mir-24-
2:CDKN2A in prostate cancer and hsa-mir-
19a:PTEN in both leukemia and prostate cancer.
It is suggested that those negative correlated pairs of
microRNA and target can be subjected to further
investigation, such as performing in vivo
experiments to valid the hypothesis that microRNA
could possibly play an important role in human
cancer.
ACKNOWLEDGEMENTS
K-L Ng work is supported by the National Science
Council of R.O.C. under the grant of NSC 98-2221-
E-468-013.
REFERENCES
Bentwich I. 2005. Prediction and validation of
microRNAs and their targets. FEBS Lett., 579, 5904.
Blower P.E., Verducci JS, Lin S, Zhou J, Chung JH, Dai Z,
Liu CG, Reinhold W, Lorenzi PL, Kaldjian EP, Croce
CM, Weinstein JN, Sadee W., 2007. MicroRNA
expression profiles for the NCI-60 cancer cell panel,
Mol. Cancer Ther., 6, 1483-1491.
Chan Hsiang-Han 2006. Identification of novel tumor-
associated gene (TAG) by bioinformatics analysis.
MSc. Thesis, Institute of Molecular Medicine,
National Cheng Kung University, Taiwan.
Enright, A.J., John, B., Gaul, U., Tuschl, T., Sander, C.,
Marks, D.S., 2003. MicroRNA targets in Drosophila.
Genome Biology, 5(1):R1.
Garzon Ramiro, Fabbri Muller, Cimmino Amelia, Calin
George A. and Croce Carlo M., 2006. MicroRNA
expression and function in cancer. Trends in
Molecular Medicine, 12, 580-588.
Gene Ontology Consortium 2006. The Gene Ontology
(GO) project in 2006. Nucl. Acids Res, 34, D322–326.
Griffiths-Jones S., Grocock Russell J., van Dongen Stijn,
Bateman Alex, Enright Anton J., 2006. miRBase:
microRNA sequences, targets and gene nomenclature.
Nucl. Acids Research, 34, 140-144.
He Xiaoting, Cao Xiufeng 2007. MicroRNA and
esophageal carcinoma. J.N.M.U., 21, 201-206.
John B., Enright A.J., Aravin A., Tuschl T., Sander C.,
Marks D.S., 2004. Human MicroRNA targets. PLoS
Biol. 2(11), e363.
Kanehisa M., Araki M., Goto S., Hattori M., Hirakawa M.,
Itoh M., Katayama T., Kawashima S., Okuda S.,
Tokimatsu T., and Yamanishi Y., 2008. KEGG for
linking genomes to life and the environment. Nucl.
Acids Res., 36, 480-484
Kruger Jan and Rehmsmeier Marc 2006. RNAhybrid:
miRNA target prediction easy, fast and flexible,
Nucleic Acids Research, 34, 451-454.
Lin Kuan-Ting, Liu Chia-Hung, Chiou Jen-Jie, Tseng
Wen-Hsien, Lin Kuang-Lung, Hsu Chun-Nan 2007.
Gene Name Service: No-Nonsense Alias Resolution
Service for Homo Sapiens Genes. Proceedings of the
2007 IEEE/WIC/ACM International Conferences on
Web Intelligence and Intelligent Agent Technology-
Workshops, 185-188.
Sethupathy P., Corda B., Hatzigeorgiou A. 2005 TarBase:
A comprehensive database of experimentally
supported animal microRNA targets. RNA, 12, 192-
197.
Shankavaram U.T., Uma T., Reinhold William C.,
Nishizuka Satoshi, Major Sylvia, Morita Daisaku,
Chary Krishna K., Reimers Mark A., Scherf Uwe,
Kahn Ari, Dolginow Douglas, Cossman Jeffrey,
Kaldjian Eric P., Scudiero Dominic A., Petricoin
Emanuel, Liotta Lance, Lee Jae K., Weinsteinu John
N, 2007. Transcript and protein expression profiles of
the NCI-60 cancer cell panel: an integromic
microarray study. Molecular Cancer Therapeutics, 6,
820-832.
Shankavaram U.T., Varma S, Kane D, Sunshine M, Chary
KK, Reinhold WC, Pommier Y, Weinstein JN., 2009.
CellMiner: a relational database and query tool for the
NCI-60 cancer cell lines. BMC Genomics,10, 277..
Wu Dan and Hu Lan 2006. Micro-RNA: A New Kind of
Gene Regulators. Agricultural Sci. in China, 5, 77-80.
Zhang Baohong, Pan Xiaoping, Cobb George P.,
Anderson Todd A., 2007. microRNAs as oncogenes
and tumor suppressors. Develop. Biol, 302, 1-12.
BIOINFORMATICS 2010 - International Conference on Bioinformatics
146