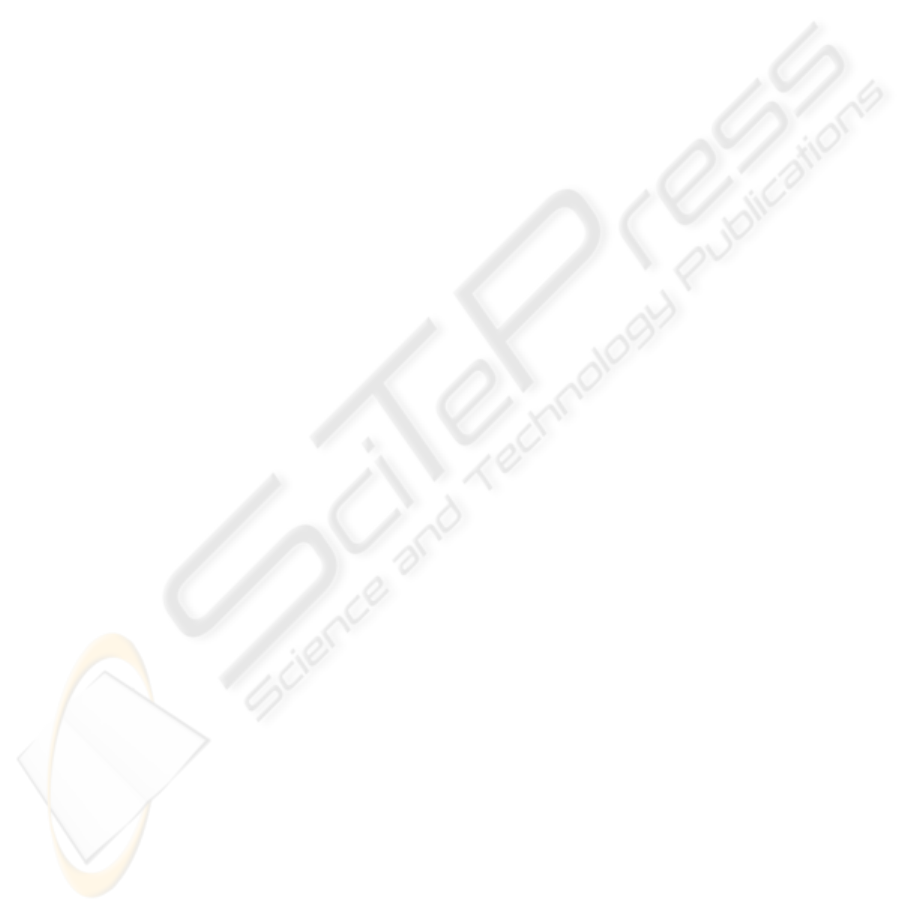
segmentation would not be feasible. In such a
situation, the proposed approach provides real-time
low-latency online speaker tracking at the cost of
little performance degradation.
5 CONCLUSIONS AND FUTURE
WORK
In this paper, an online speaker tracking system,
designed for an Ambient Intelligence scenario, is
presented an evaluated. The system processes
continuous audio streams and outputs a speaker
identification decision for fixed-length (one second)
segments. Speaker detection is done by means of a
MAP-UBM speaker verification backend. A
calibration stage is applied which linearly maps
detection scores to likelihood ratios. Calibration
parameters are estimated beforehand based on
development data, yielding significant performance
improvements without increasing the computational
cost, which is crucial for a real-time low-latency
system. An alternative speaker tracking system,
based on an offline segmentation of the audio stream
has been developed and evaluated for reference.
Experiments have been carried out on a subset of
the AMI Corpus of meeting conversations. Results
demonstrate that better results can be attained when
the UBM is estimated from data matching test
conditions (same room, same speakers), instead of
using general but unrelated data. The calibration
stage provides performance improvements in all
cases. Finally, offline segmentation of audio streams
actually improves speaker tracking performance
with regard to using fixed-length segments.
However, depending on the scenario and the
required latency, offline audio segmentation would
not be feasible. The proposed system provides real-
time low-latency online speaker tracking with little
performance degradation.
Current work involves increasing the robustness
of detection scores (and decisions) by using
information from past segments. Future work
includes using detection scores in a speaker
verification framework (thus allowing the detection
of multiple speakers), and making a smart use of all
the available data through new UBM estimation
strategies.
ACKNOWLEDGEMENTS
This work has been partially funded by the
Government of the Basque Country, under program
SAIOTEK, project S-PE07IK03; and the Spanish
MICINN, under Plan Nacional de I+D+i, project
TIN2009-07446.
REFERENCES
Abowd, G.D., Mynatt, E.D., "Designing for the Human
Experience in Smart Environments" in D.J. Cook and
S.K. Das, Editors, Smart Environments: Technology,
Protocols, and Applications, Wiley, 153-174, 2005.
Bonastre, J.F., Delacourt, P., Fredouille, C., Merlin, T. and
Wellekens, C., "A Speaker Tracking System based on
Speaker Turn Detection for NIST Evaluation", in Pro-
ceeding of the IEEE International Conference on
Acoustics, Speech and Signal Processing, Istanbul,
Turkey, 2000.
Brummer, N. and Preez, J., "Application Independent
Evaluation of Speaker Detection", Computer Speech
and Language, 20:230-275, 2006.
Carletta, J., "Unleashing the killer corpus: experiences in
creating the multi-everything AMI Meeting Corpus".
Language Resources and Evaluation Journal , 41(2):
181-190, 2007.
Chen, S.C. and Gopalakrishnan, P.S., "Speaker, Environ-
ment and Channel Change Detection and Clustering
via the Bayesian Information Criterion", Proceedings
of DARPA Broadcast News Transcription and Under-
standing Workshop, 1998.
Cook, D.J., Augusto, J.C., Jakkula, V.R., "Ambient Intelli-
gence: Technologies, Applications, and Opportuni-
ties", Pervasive and Mobile Computing, 5(4): 277-298,
2009.
ISTAG, "Scenarios for Ambient Intelligence in 2010".
European Commission Report, 2001.
Istrate, D., Scheffer, N., Fredouille, C. and Bonastre, J.F.,
"Broadcast News Speaker Tracking for ESTER 2005
Campaign", in Proceedings of the International Con-
ference on Speech and Language Processing, Lisboa,
2005.
Liu, D., Kiecza, D., Srivastava, A. and Kubala, F., "Online
Speaker Adaptation and Tracking for Real-time
Speech Recognition", in Proceedings of the Interna-
tional Conference on Speech and Language
Processing, Lisboa, 2005.
Lu, L. and Zhang H.J., “Unsupervised Speaker Segmenta-
tion and Tracking in Real-time Audio Content Analy-
sis”, Multimedia Systems,10:332-343, 2005.
Martin, A., Doddington, G., Kamm, T., Ordowski, M., and
Przybocki, M., "The DET curve in assessment of de-
tection task performance", in Proceedings of the 5th
European Conference on Speech Communication and
Technology (Eurospeech), Vol. 4, pp. 1895-1898,
1997.
ICAART 2010 - 2nd International Conference on Agents and Artificial Intelligence
348