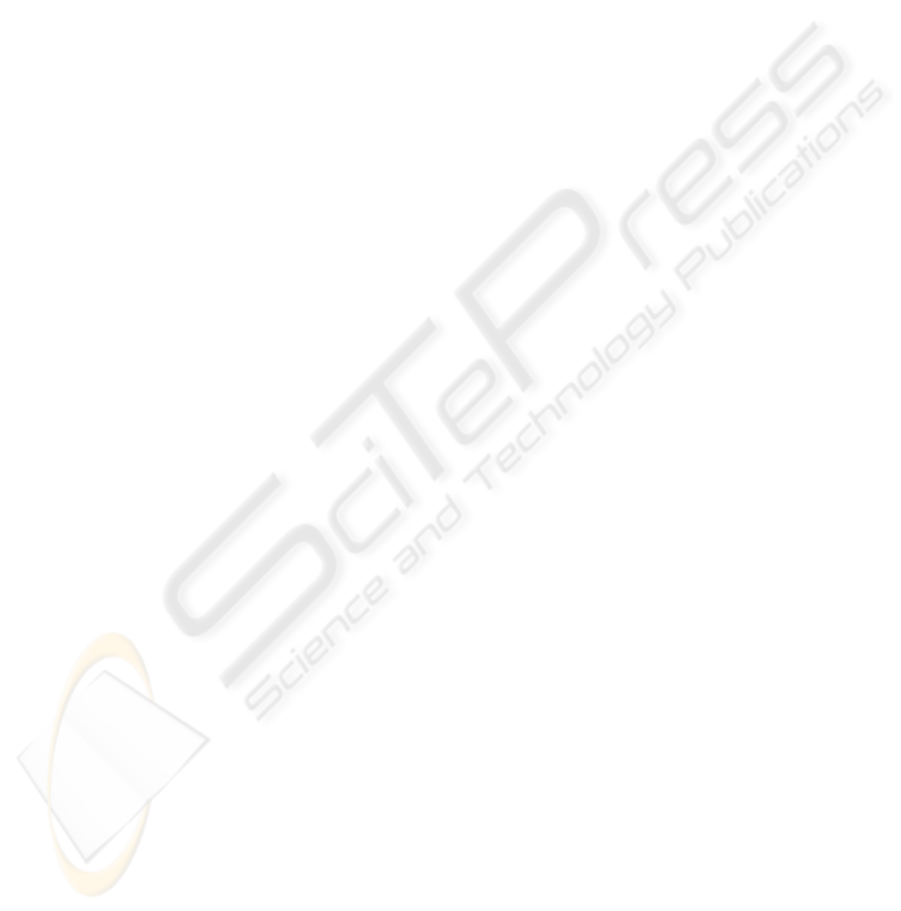
5 CONCLUSIONS
One of the challenges of scene interpretation is the
use of structural information for improving the inter-
pretation of a scene. This paper describes an approach
for integrating two separate sources of structure and
shows that this combination improves the detection
of windows in the fac¸ade domain. The low-level
structure detector typically computes a large number
of potential primitive and structured evidences. A
middle-layer component called “Matchbox” reduces
this number by selecting the best primitives and struc-
tures. High-level reasoning creates hypotheses of
missing objects that are caused by the context of the
surrounding scene objects. These hypotheses are con-
firmed or refuted by comparing them to the low-level
results. Thus, the Matchbox mediates between both
sources of structures, and relates high-level concepts
to low-level detections.
The approach was tested on a set of fac¸ade im-
ages, which are rich in structure. The results show
that combining visual and compositional structure can
considerably improve the detection of windows in
this domain compared to pure bottom-up approach
based on visual structure alone. Not all the correct
high-level hypotheses were confirmed by low-level
evidence, mostly due to poor contrast and partially
occluded windows. Further improvements might be
possible by using additional low-level detectors for
confirming or refuting high-level hypotheses.
ACKNOWLEDGEMENTS
This research has been supported by the European
Community under the grant IST 027113, eTRIMS
- eTraining for Interpreting Images of Man-Made
Scenes.
REFERENCES
Freund, Y. and Schapire, R. (1997). A decision-theoretic
generalization of on-line learning and an application
to boosting. Journal of Computer and System Sci-
ences, 55(1):119–139.
Friedman, J., Hastie, T., and Tibshirani, R. (1998). Ad-
ditive logistic regression: a statistical view of boost-
ing. Technical report, Department of Statistics, Se-
quoia Hall, Stanford Univerity.
Fusier, F., Valentin, V., Bremond, F., Thonnat, M., Borg,
M., Thirde, D., and Ferryman, J. (2007). Video under-
standing for complex activity recognition. Machine
Vision and Applications (MVA), 18:167–188.
Grabner, H., Grabner, M., and Bischof, H. (2006). Real-
time tracking via on-line boosting. In British Machine
Vision Conference, volume 1, pages 47–56.
Hartz, J., Hotz, L., Neumann, B., and Terzic, K. (2009).
Automatic incremental model learning for scene inter-
pretation. In Proc. of the Fourth IASTED International
Conference on Computational Intelligence, Honolulu,
Hawaii.
Hotz, L. and Neumann, B. (2005). Scene Interpretation as a
Configuration Task. K
¨
unstliche Intelligenz, 3:59–65.
Hotz, L., Neumann, B., and Terzic, K. (2008). High-
level expectations for low-level image processing. In
KI 2008: Advances in Artificial Intelligence, volume
5243 of Springer Lecture Notes in Computer Science,
pages 87–94.
Hummel, B., Thiemann, W., and Lulcheva, I. (2008). Scene
understanding of urban road intersections with de-
scription logic. In Cohn, A. G., Hogg, D. C., M
¨
oller,
R., and Neumann, B., editors, Logic and Probability
for Scene Interpretation, number 08091 in Dagstuhl
Seminar Proceedings, Dagstuhl, Germany. Schloss
Dagstuhl - Leibniz-Zentrum fuer Informatik, Ger-
many.
Mohnhaupt, M. and Neumann, B. (1993). Understanding
object motion: recognition, learning and spatiotem-
poral reasoning. Robotics and Autonomous Systems,
pages 65–91.
Russel, S. and Norvig, P. (2003). Artificial Intelligence - A
Modern Approach. Prentice-Hall.
Seo, Y.-W., Ratliff, N., and Urmson, C. (2009). Self-
supervised aerial image analysis for extracting park-
ing lot structure. In Proc. of Twenty-First Int. Joint
Conf. on AI IJCAI-09, pages 1837–1842, Pasadena.
Soininen, T., Tiihonen, J., M
¨
annist
¨
o, T., and Sulonen, R.
(1998). Towards a General Ontology of Configura-
tion. Artificial Intelligence for Engineering Design,
Analysis and Manufacturing (1998), 12, pages 357–
372.
Terzic, K., Hotz, L., and Neumann, B. (2007). Division of
Work During Behaviour Recognition - The SCENIC
Approach. In Schuldt, A., editor, Behaviour Moni-
toring and Interpretation, Workshop Proceedings KI,
Universit
¨
at Bremen.
ˇ
Cech, J. and
ˇ
S
´
ara, R. (2007). Language of the structural
models for constrained image segmentation. Tech-
nical Report Technical Report TN-eTRIMS-CMP-03-
2007, Czech Technical University, Prague.
Yang, C. and Yang, M.-H. (1997). Constraint Networks: A
Survey. In Proc. of the IEEE International Conference
on Systems, Man and Cybernetics, volume 2, Orlando,
Florida, USA. Institute of Electrical and Electronics
Engineers (IEEE).
Zhu, S. and Mumford, D. (2006). A Stochastic Grammar of
Images. Foundations and Trends in Computer Graph-
ics and Vision. Prentice-Hall.
ICAART 2010 - 2nd International Conference on Agents and Artificial Intelligence
364