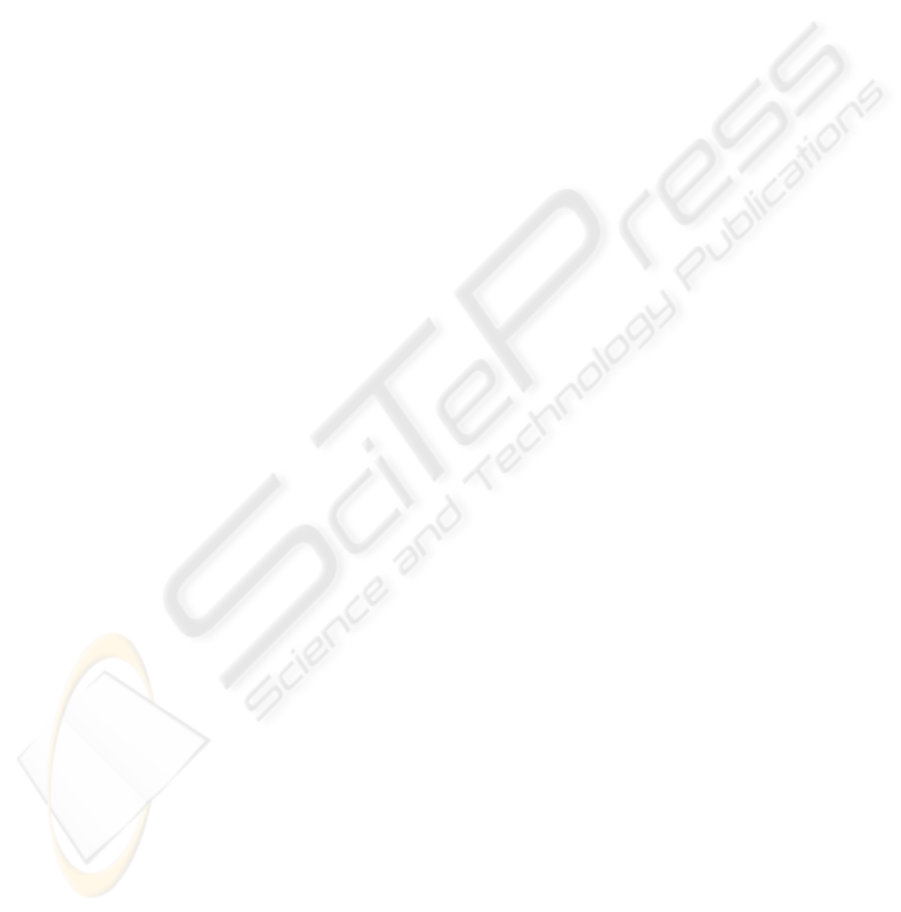
Models has been demonstrated. The experimental
results have shown that by using Hidden Markov
Models as a contextual analysis model, overall
performance can be greatly improved, demonstrating
its excellent potentials for further development.
While the precision of this technique is only 0.80
with a false positive rate of 0.27, this technique is
evaluated over 220 retina images obtained from
various source, thus demonstrating the ability to
overcome diversity usually found in a large-scale
database.
REFERENCES
Cree M. J., Olson J. A., McHardy K. C., Forrester J. V.,
Sharp P. F., 1996, “Automated Microaneurysms
Detection”, Proceedings of the International
Conference on Image Processing.
Fu Y. and Browne A., 2007, “Using Ensembles of Neural
Networks to Improve Automatic Relevance
Determination”, Proceedings of International Joint
Conference on Neural Networks, Orlando, Florida,
USA.
HTK Toolkit, [Internet], >Available from
http://htk.eng.cam.ac.uk> Last Access [17/08/09].
Kittler J., Mohamad H., Duin R. P. W., Matas J., 1998,
“On Combining Classifiers”, IEEE Transactions on
Pattern Analysis and Machine Intelligence. Vol. 3 (3).
Niemeijer M., Ginneken B., Stal J., Suttorp-Schulten M. S.
A., Abramoff M. D., 2005, “Automatic Detection of
Red Lesions in Digital Color Fundus Photograph”,
IEEE Transaction on Medical Imaging, Vol. 25(5).
Optimal Detection and Decision –Support Diagnosis of
Diabetic Retinopathy (2005), Internet, <Available
from http://www.it.lut.fi/project/imageret/> Last
Accessed [17/08/08]
Parui S. K., Guin K., Bhattacharyam U., Chaudhuri B. B.,
2008, “Online Handwritten Bangla Character
Recognition Using HMM”, IEEE Transaction 2008.
Rabiner L. R., 1989, “A Tutorial on Hidden Markov
Models and Selected Applications in Speech
Recognition”, Proceedings of the IEEE, Vol. 77 (2).
Sagar A. V., S. Balasubramaniam, V. Chandrasekaran,
2007, “A Novel Integrated approach using dynamic
thresholding and edge detection for automatic
detection of exudates in digital fundus retina images”,
Proceedings of the International Conference on
Computing: Theory and Application.
Sinthanayothin C., Boyce J. F., Williamsom T.H., Cool
H.L., Mensah E., Lai S., Usher D., 2002, “Automated
Detection of Diabetic Retinopathy on Digital Fundus
Images”, Diabetic Medicine, Vol. 19, ppl105-112.
Spencer T., Olson J. A., McHardy K. C., Sharp P. F.,
Forrester J. V., 1996, “An Image Processing strategy
for the Segmentation and Quantification of
Microaneurysms in Fluorescein Angiograms of the
Ocular Fundus”, Computers and Biomedical Research,
Vol. 29, pp284-302.
Walter T., Klein J., 2000, “Automatic Detection of Micro
aneurysms in Color Fundus Images of the Human
Retina by means of the Bounding Box Closing”,
Proceedings of the Third International Symposium on
Medical Data Analysis, Rome, Italy.
World Health Organisation. [Internet], <Available from
http://www.who.int/blindness/causes/priority/en/index
6.html Last Access [17/08/09]
Won K., Prugel-Bennet A., Krogh A., 2006, “Evolving the
Structure of Hidden Markob Models”, IEEE
Transaction on Evoutionary Computation, Vol. 10 (1).
HEALTHINF 2010 - International Conference on Health Informatics
274