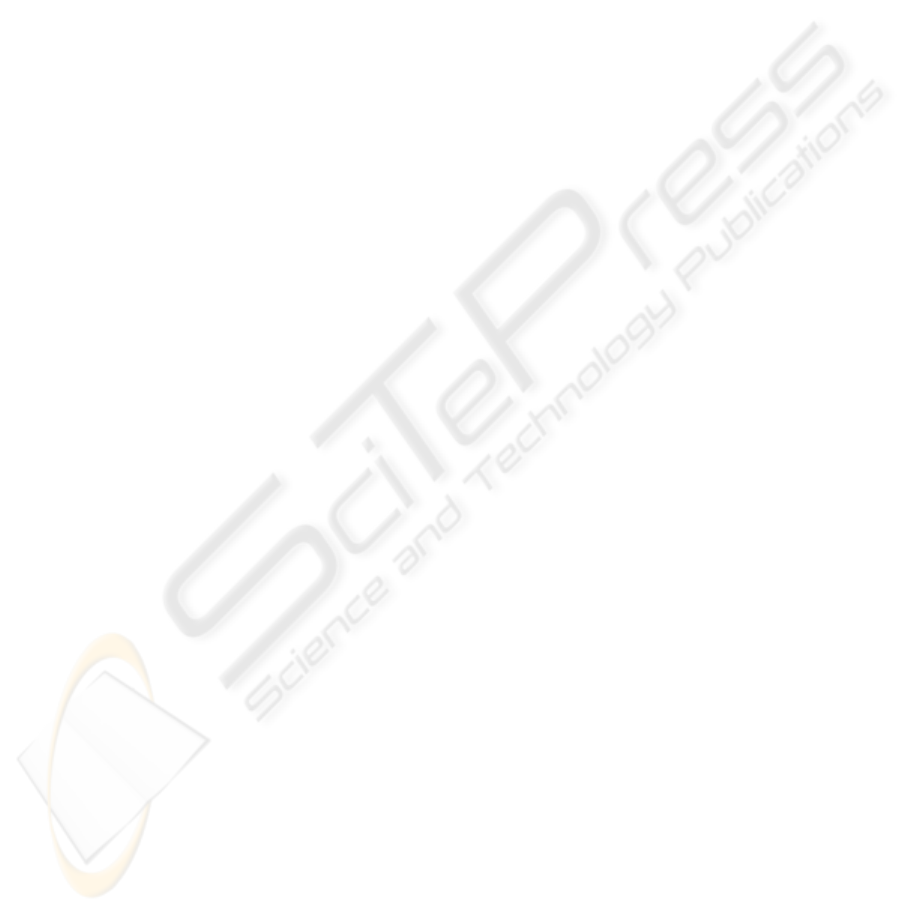
In the same manner as the indices used to define
aspects of the real world system when using a Deci-
sion Support System, the attributes of an individual
may not all be of the same type, nor may they all
be static during the course of the simulation. Some
values may be numeric in nature, such as how much
blood a patient has lost, others may require fuzzy
states to more accurately represent naturally occur-
ring, non-numeric conditions such as human concen-
tration.
These factors can be defined as either mutable or
immutable attributes, representing factors that may or
may not change during the course of the simulation
respectively. Mutable attributes may represent such
factors as the level of pain or stress experienced by
an individual at a given moment or the short term
memory of an individual with regard to their expe-
riences within the simulated period. Immutable at-
tributes may represent the level of training a nurse or
doctor has, or the individuals ability to communicate
based on their competence in the local language, these
form the properties of the agent which are unlikely to
change during the course of the simulation.
6 CONCLUSIONS
In the field of healthcare operational management it
has been shown that simulation can be a useful tool.
A number of examples exist in the literature, although
the lack of results from complete implementations
shows that simulation in this area has not reached its
full potential.
A number of requirements for a general and ver-
ifiable simulation are presented. Starting from these
requirements a new model has been created specifi-
cally to work towards creating a tool that can be used
as a basic component of a Decision Support System
which will be easily usable to healthcare managers
to gain insight into the inner workings of current or
planned emergency departments.
A key feature of this project is the use of Agent-
Based Modelling techniques to both take into account
the important social and psychological factors that
come into play during human interactions in high
stress environments such as emergency departments,
but also to aid to reducing the dehumanising appear-
ance of simulation tools from the point of view of
healthcare managers.
The work on this model continues to proceed
based on ongoing investigation and partnership with
a number of hospitals.
ACKNOWLEDGEMENTS
We would like to thank Montse Edo for the detailed
and invaluable assistance provided in describing the
operations of the triage department where she works.
Without the help afforded, this work would not have
been possible.
REFERENCES
Ayatollahi, H., Bath, P. A., and Goodacre, S. (2009). It
in the emergency department - what is the impact of
technology? Proceedings of the International Con-
ference of Health Informatics (HEALTHINF), pages
454–457.
Brailsford, S. (2007). Tutorial: Advances and challenges
in healthcare simulation modeling. Proceedings of the
Winter Simulation Conference, pages 1436 – 1448.
Brailsford, S., Sykes, J., and Harper, P. (2006). Incorpo-
rating human behavior in healthcare simulation mod-
els. Proceedings of the Winter Simulation Conference,
pages 466–472.
Cederman, L. (2002). Endogenizing geopolitical bound-
aries with agent-based modeling. Proceedings of the
National Academy of Sciences of the United States of
America, 9(3):7296–7303.
Connelly, L. and Bair, A. (2004). Discrete event simu-
lation of emergency department activity: a platform
for system-level operations research. Academic Emer-
gency Medicine, 11(11):1177–1185.
Fone, D., Hollinghurst, S., Temple, M., and Round, A.
(2003). Systematic review of the use and value of
computer simulation modelling in population health
and health care delivery. Journal of Public Health,
25(4):325–335.
Gonz´alez, J., Dalforno, C., Suppi, R., and Luque, E. (2009).
A fuzzy logic fish school model. Procc. ICCS 2009,
LNCS, 5544:13–22.
Lowery, J. (1996). Introduction to simulation in health
care. Proceedings of the Winter Simulation Confer-
ence, pages 78 – 84.
Stainsby, H., Taboada, M., and Luque, E. (2009). Towards
an agent-based simulation of hospital emergency de-
partments. Services Computing, 2009. SCC ’09. IEEE
International Conference on, pages 536 – 539.
Taylor, K. and Dangerfield, B. (2005). Modelling the feed-
back effects of reconfiguring health services. Journal
of the Operational Research Society, 56(6):659 – 675.
Walker, D., Southgate, J., Hill, G., and ..., M. H. (2004).
The epitheliome: agent-based modelling of the social
behaviour of cells. Biosystems, 76:89–100.
Wang, J., Gwebu, K., Shanker, M., and Troutt, M. (2009).
An application of agent-based simulation to knowl-
edge sharing. Decision Support Systems, 46:532–541.
AGENT-BASED SIMULATION TO SUPPORT DECISION MAKING IN HEALTHCARE MANAGEMENT
PLANNING
441