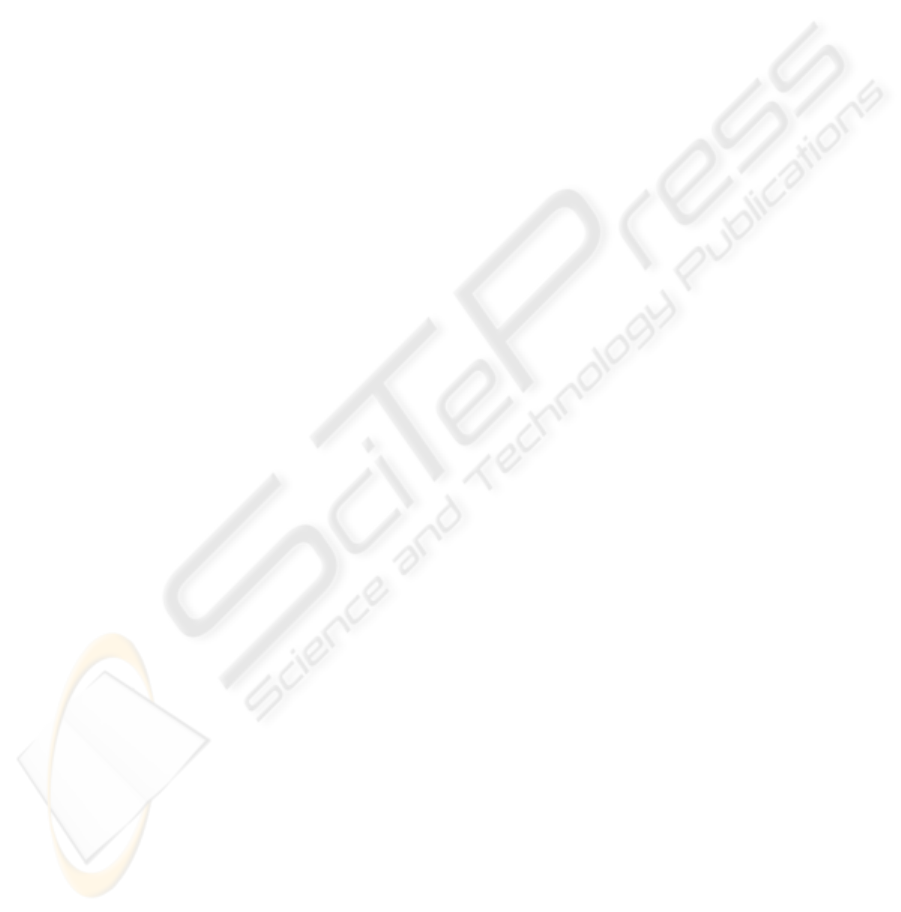
(Poynard et al., 2007) which pooled 6,378 subjects
with both FibroTest and biopsy (3,501 chronic hep-
atitis C). The mean standardized AUROC was 0.85
(0.82-0.87). The robustness of these results is clearly
demonstrated by this cross-validation, while i-Biopsy
results need to be cross-validated. The fact that i-
Biopsy , in its actual setting, relies on routine ul-
trasound features is both a strong point and a weak
one, because of the subjectiveness in ultrasound im-
ages interpretation. It is worth to note that in certain
circumstances the result of the liver i-Biopsy could
be superior to that of real biopsy. When building
the i-CDSS, the results of the potentially erroneous
biopsies, which are not fulfilling some technical re-
quirements, were eliminated from the data set. Thus,
the i-Biopsy predicted results correspond only to the
results of the correctly performed biopsies, while a
number of of the real biopsy results are wrong, be-
cause they were not correctly performed. Due to the
invasive and unpleasant nature of the biopsy, is very
improbable that a patient will accept a technically in-
correct biopsy to be repeated. Unlike real biopsy,
i-Biopsy can be used to evaluate fibrosis evolution,
which is of interest in various biomedical and pharma-
ceutical studies, because, being non-invasive,painless
and without any risk, can be repeated as many time as
needed.
Also, in the early stages of liver diseases, often the
symptoms are not really harmful for the patient, but
the treatment is more effective than in more advanced
fibrosis stages. The physician will hesitate to indicate
an invasive, painful and risky liver biopsy, and the pa-
tients are not as worried about their disease as they are
about the pain of the biopsy. However, i-Biopsy can
be performed and an early start of the treatment could
be much more effective.
ACKNOWLEDGEMENTS
We thank to the following medical teams: Dr. Mon-
ica Lupsor, Dr. T. Suteu and Prof. Dr. R. Badea, from
Medical Imaging Department, Dr. H. Stefanescu and
Dr. Z. Sparchez, from Hepatology Department, Dr.
A. Serban from Pathology Department, Dr. N. Crisan,
Dr. B. Feciche and Prof. Dr. I. Coman, from Urology
Department, University of Medicine and Pharmacy
Cluj-Napoca, Romania, Dr. Carmen Floares, Dr. O.
Balcescu, Dr. Ioana Neagoe Dr. Loredana Balacescu,
Dr. Oana Tudoran, and Prof. Dr. A. Irimie, from Can-
cer Institute Cluj-Napoca, Romania. We also thank
to the computer science team: Dr. F. Manolache, E.
Suica, and T. Popa.
REFERENCES
Balacescu, O., Neagoe, I., Balacescu, L., Crisan, N., Feci-
che, B., Tudoran, O., Coman, I., and Irimie, A. (2008).
Angiogenesis serum protein quantifcation for prostate
pathology. Curr Urol, (2):181–187.
Berner, E., editor (2007). Clinical decision support systems.
Springer: New York, NY.
Fawcett, T. (2004). Roc graphs: Notes and practical con-
siderations for researchers. technical report. Technical
report, Palo Alto, USA: HP Laboratories.
Floares, A. G. (2008). Intelligent systems for interferon
treatment decision support in chronic hepatitis c based
on i-biopsy. In IDAMAP - Intelligent Data Analysis in
Biomedicine and Pharmacology, Washington DC.
Floares, A. G. (2009a). Intelligent clinical decision supports
for interferon treatment in chronic hepatitis c and b
based on i-biopsy. In International Joint Conference
on Neural Networks, 2009, Atlanta, Georgia, USA.
Floares, A. G. (2009b). Liver i-Biopsy and the Correspond-
ing Intelligent Fibrosis Scoring Systems: i-Metavir F
and i-Ishak F, pages 253–264. Lecture Notes in Com-
puter Science. Springer Berlin / Heidelberg.
Floares, A. G., Lupsor, M., Stefanescu, H., Sparchez, Z.,
Serban, A., Suteu, T., and Badea, R. (2008). Toward
intelligent virtual biopsy: Using artificial intelligence
to predict fibrosis stage in chronic hepatitis c patients
without biopsy. Journal of Hepatology, 48(2).
Freund, Y. and Schapire, R. E. (1997). A decision theoretic
generalization of on line learning and an application to
boosting. Journal of Computer and System Sciences,
55(1):119–139.
Guyon, I., Gunn, S., Nikravesh, M., and Zadeh, L. (2006).
Feature Extraction: Foundations and Applications.
Studies in Fuzziness and Soft Computing. Springer.
Lindor, A. (1996). The role of ultrasonography and
automatic-needle biopsy in outpatient percutaneous
liver biopsy. Hepatology, 23:1079–1083.
Poynard, T., Morra, R., Halfon, P., Castera, L., Ratziu, V.,
Imbert-Bismut, F., Naveau, S., Thabut, D., Lebrec, D.,
Zoulim, F., Bourliere, M., Cacoub, P., Messous, D.,
Muntenau, M., and de Ledinghen, V. (2007). Meta-
analyses of fibrotest diagnostic value in chronic liver
disease. BMC Gastroenterology, 7(40).
Quinlan, J. (1993). C4.5 : Programs for Machine Learning.
Morgan Kaufmann.
Shaheen, A., Wan, A., and Myers, R. (2007). Fibrotest and
fibroscan for the prediction of hepatitis c-related fibro-
sis: a systematic review of diagnostic test accuracy.
Am J Gastroenterol, 102(11):2589–2600.
Tobkes, A. and Nord, H. J. (1995). Liver biopsy: Review of
methodology and complications. Digestive Disorders,
13:267–274.
INTELLIGENT CLINICAL DECISION SUPPORT SYSTEMS
287