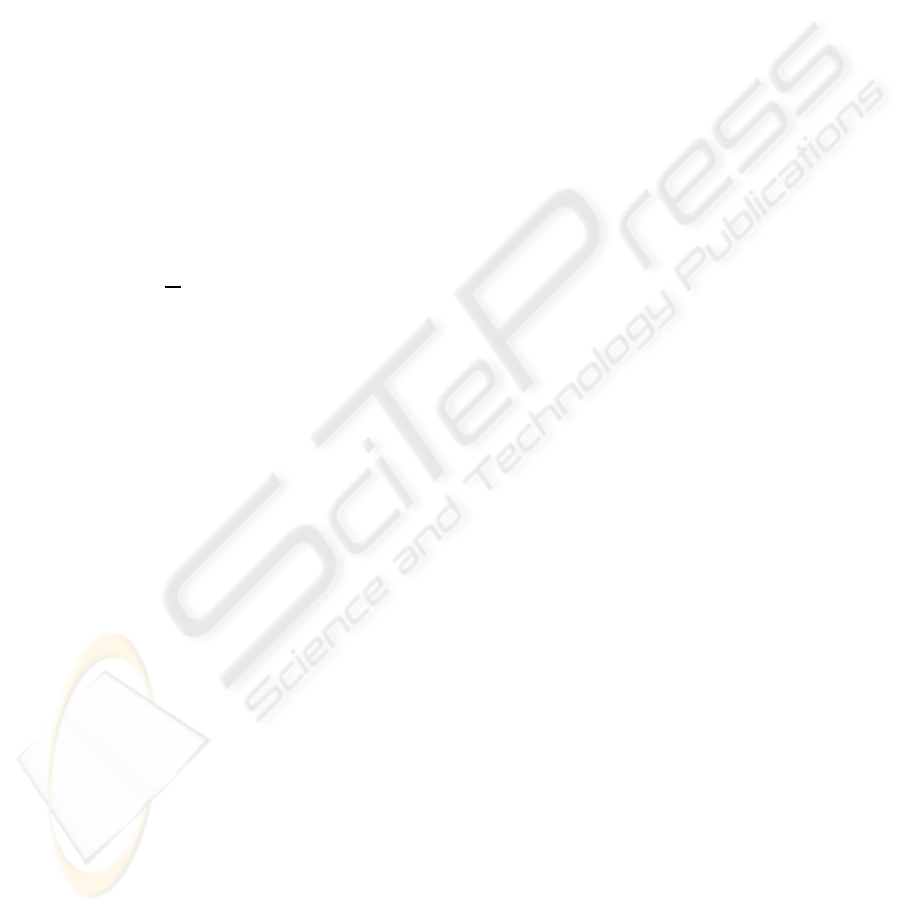
put signal) is very similar to the spectrum of the noise
signal. This suggests that model fit is good, as one
cannot expect the model to be able to predict the ran-
dom measurement noise.
Computer simulations using the “ideal” data set
showed that both parameter estimation methods could
accurately identify the simulated system. The nor-
malised parameter bias error for both methods was
less than 0.1%. The results of the Monte Carlo sim-
ulation using the “low pass with measurement noise”
data set are shown in Figure 5. Under these simu-
lated experimental conditions, neither method could
retrieve the parameters of the simulated system. It is
interesting to note that the DE estimates of the pa-
rameters of h(τ) (Figures 4 and 5 D) contain more
high frequencyestimation error than the KH estimates
(Figures 4 and 5 A) for both experimental and simu-
lated data. This is probably because the LM method
(Westwick and Kearney, 2003) used to estimate h(τ)
in the KH algorithm (Algorithm 1 step 4) approxi-
mates the Hessian
ˆ
H as
ˆ
H =
1
N
J
T
J+ µ
k
I
M
(10)
and the µ
k
I
M
term improves the condition of
ˆ
H.
4 CONCLUSIONS
The accuracy of two Wiener-Hammerstein parame-
ter estimation methods has been compared using ex-
perimental data and computer simulations. Our work
has shown that DE gives fast robust convergence and
good local search performance, providing similar ac-
curacy as the local optimisation method developed by
Korenberg and Hunter (Korenberg and Hunter, 1986).
The DE method offers the advantageof simplicity and
flexibility, for example making it easy to use different
cost functions. Also, as it is a global method, it should
be less sensitive to parameter initialisation, but addi-
tional work is require to verify this feature. Further
investigation into the convergence properties of both
methods and ways to reduce parameter bias and vari-
ance under experimental conditions is required.
ACKNOWLEDGEMENTS
The authors would like to thank the Gerald Kerkut
Trust, the LSI Forum, the ISVR Rayleigh Scholarship
and the BBSRC for their support.
REFERENCES
Al-Duwaish, H. (2000). A genetic approach to the identi-
fication of linear dynamical systems with static non-
linearities. International Journal of Systems Science,
31(3):307–313.
Bai, E., Cai, Z., Dudley-Javorosk, S., and Shields,
R. K. (2009). Identification of a modified wiener-
hammerstein system and its application in electrically
stimulated paralyzed skeletal muscle modeling. Auto-
matica, 45(3):736 – 743.
Dempsey, E. and Westwick, D. (2004). Identification
of hammerstein models with cubic spline nonlinear-
ities. IEEE Transactions on Biomedical Engineering,
51(2):237–245.
Dewhirst, O., Simpson, D., Allen, R., and Newland, P.
(2009). Neuromuscular reflex control of limb move-
ment - validating models of the locust’s hind leg con-
trol system using physiological input signals. In IEEE
EMBS 09, 4th International Conference on Neural En-
gineering. IEEE EMBS.
Juusola, M., Niven, J., and French, A. (2003). Shaker
k+ channels contribute early nonlinear amplification
to the light response in drosophila photoreceptors. J
Neurophysiol, 90(3):2014–2021.
Korenberg, M. and Hunter, I. (1986). The identification of
nonlinear biological systems: lnl cascade models. Bi-
ological Cybernetics, 50(2):125–134.
Marquardt, D. W. (1963). An algorithm for least-squares
estimation of nonlinear parameters. SIAM Journal on
Applied Mathematics, 11(2):431–441.
Newland, P. and Kondoh, Y. (1997). Dynamics of neurons
controlling movements of a locust hind leg iii: Exten-
sor tibiae motor neurons. The Journal of Neurophysi-
ology, 77(5):3297–3310.
Schoukens, J., Pintelon, R., and Enqvist, M. (2008). Study
of the lti relations between the outputs of two coupled
wiener systems and its application to the generation
of initial estimates for wiener-hammerstein systems.
Automatica, 44(7):1654 – 1665.
Schoukens, J., Suykens, J., and Ljung, L. (2009). Wiener
hammerstein benchmark. In Proc. 15 IFAC Sympo-
sium on System Identification, St Malo, France.
Song, D., Marmarelis, V., and Berger, T. (2009). Paramet-
ric and non-parametric modeling of short-term synap-
tic plasticity. part i: Computational study. J Comput
Neurosci, 26(1):1 – 19.
Storn, R. and Price, K. (1997). Differential evolution a sim-
ple and efficient heuristic for global optimization over
continuous spaces. Journal of Global Optimization,
11:341–359.
Westwick, D. and Kearney, R. (2003). Identification of Non-
linear Physiological Systems. Wiley-IEEE Computer
Society Pr, Piscataway, New Jersey, 1st edition.
BIOSIGNALS 2010 - International Conference on Bio-inspired Systems and Signal Processing
276