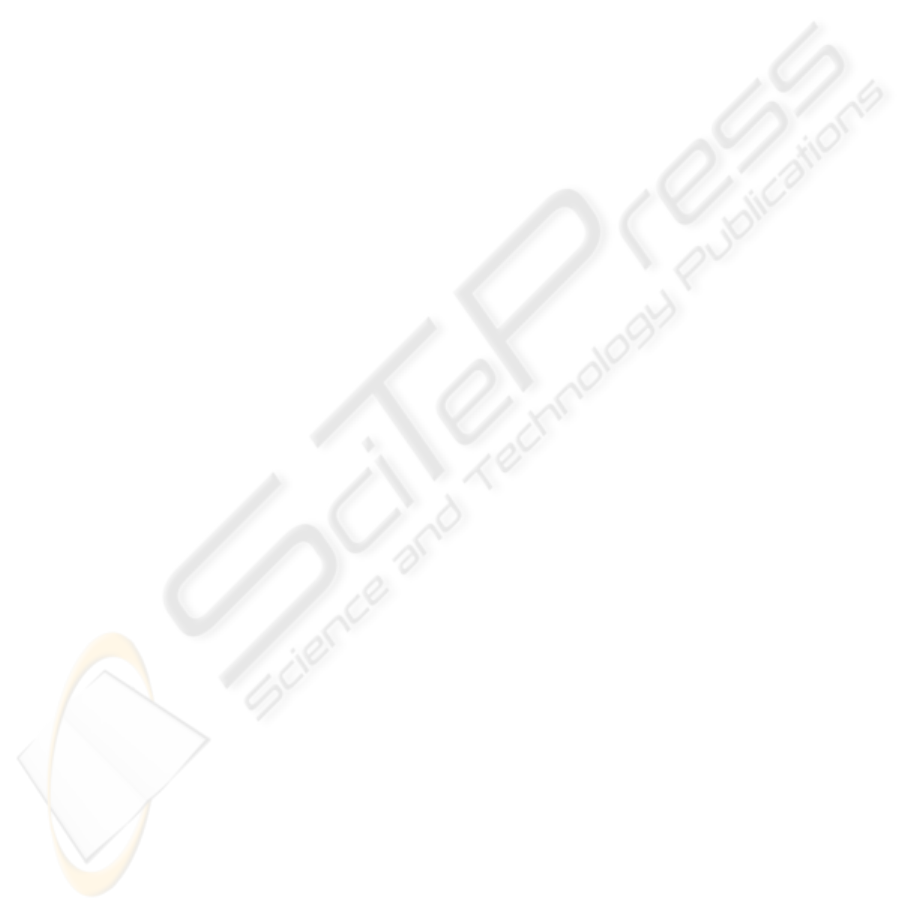
ods.
In the feasibility study. stress was successfully
caused and reported by 26 subjects. By measuring
speech and a subjective report of stress, acoustic fea-
tures of stress in speech were determined. These fea-
tures were able to explain 70% of variance of sub-
jectively reported experienced stress. Hence, demon-
strating the possible success of speech as an objective
measure of experienced stress.
The reported stress, induced by story telling, was
quite dispersed. Although this is partly due to inter-
personal difference, this also indicates that overall
the stories did had an influence. Moreover, a trend
was found for the anxiety inducing story, corroborat-
ing this influence. These results not only suggest the
value of story telling, but also its drawbacks. Two
problems can be identified. First, stories are heav-
ily dependent on their temporal course; i.e., a story
needs a build-up before inducing an affective state.
Second, there were substantial inter-personal differ-
ences in the experience of the stories. However, con-
trary to many other methods, this method is likely to
create true emotions. The triangulation through var-
ious speech characteristics and the SUD did indicate
that indeed true emotions were triggered through the
story-telling.
Considering the number of patients used to create
an acoustic profile of stress characteristics in speech,
the achieved explained variance of 70% for the emo-
tional conditions is high. In particular, being a non-
personalized profile, some generic features of stress-
ful speech seem to be uncovered. However, also
some restrictions apply: a) only PTSD patients were
used, other patient groups might show other stress re-
sponses; b) different kinds of stress may exists; and
c) any restrictions applying to story telling as emo-
tion elicitation method may have affected the results.
This triplet can be considered as future research chal-
lenges. Namely, to use other patient groups, different
stressful emotions, and different emotion elicitation
techniques.
This study has demonstrated that giving a second
opinion based on the speech signal is feasible. An
assistive system can help the clinical setting through
several ways: 1) to support the measurement of a
stress response; 2) to assist in deciding whether or
not the patient has excessive stress; and 3) to aid in
the treatment of a stress disorder. Therefore, by mak-
ing the diagnosis objective, the measurement is made
more reliable; i.e., by no longer solely relying on in-
trospection. Hence, objective measurement increases
inter- and intra-expert reliability and helps diagnosis,
decision-making, and treatment become more fine-
grained.
ACKNOWLEDGEMENTS
The patients suffering from a post-traumatic stress
disorder (PTSD), who voluntarily participated in this
research, are gratefully acknowledged. Further, we
thank the anonymous reviewers for their critical and
constructive comments on the original manuscript.
In addition, we would like to acknowledge Paul
Boersma and David Weenink (Institute of Phonetic
Sciences, University of Amsterdam, The Nether-
lands) for their work on Praat and the accompanying
manual, tutorials, and articles.
REFERENCES
American Psychiatric Association (2000). DSM-IV-TR:
Diagnostic and Statistical Manual of Mental Disorders.
Washington, DC, USA: American Psychiatric Publish-
ing, Inc., 4 (Text Revision) edition.
Cowie, R., Douglas-Cowie, E., Tsapatsoulis, N., Votsis, G.,
Kollias, S., Fellenz, W., and Taylor, J. G. (2001). Emo-
tion recognition in human–computer interaction. IEEE
Signal Processing Magazine, 18(1):32–80.
Kedem, B. (1986). Spectral analysis and discrimination by
zero-crossings. Proceedings of the IEEE, 74(11):1477–
1493.
Lazarus, R. S. (1993). From psychological stress to the
emotions: A history of changing outlooks. Annual Re-
view of Psychology, 44(1):1–22.
Lutfi, S. L., Montero, J. M., Barra-Chicote, R., Lucas-
Cuesta, J. M., and Gallardo-Antol
´
ın, A. (2009). Ex-
pressive speech identifications based on hidden markov
model. In Azevedo, L. and Londral, A. R., editors,
HEALTHINF, pages 488–494. INSTICC Press.
Rabkin, J. G. and Struening, E. L. (1976). Life events,
stress, and illness. Science, 194(4296):1013–1020.
Rothkrantz, L. J. M., Wiggers, P., van Wees, J.-W. A., and
van Vark, R. J. (2004). Voice stress analysis. Lecture
Notes in Computer Science (Text, Speech and Dialogue),
3206:449–456.
Scherer, K. R. (2003). Vocal communication of emotion: A
review of research paradigms. Speech Communication,
40(1–2):227–256.
Selye, H. (1936). A syndrome produced by diverse noxious
agents. Nature, 138(3479):32.
Van den Broek, E. L., Janssen, J. H., Westerink, J. H. D. M.,
and Healey, J. A. (2009). Prerequisits for Affective Sig-
nal Processing (ASP). In Encarnac¸
˜
ao, P. and Veloso,
A., editors, Biosignals 2009: Proceedings of the Inter-
national Conference on Bio-Inspired Systems and Signal
Processing, pages 426–433, Porto – Portugal.
Ververidis, D. and Kotropoulos, C. (2006). Emotional
speech recognition: Resources, features, and methods.
Speech Communication, 48(9):1162–1181.
Wolpe, J. (1958). Psychotherapy by reciprocal inhibition.
Stanford, California: Stanford University Press.
TOWARDS SEMI-AUTOMATED ASSISTANCE FOR THE TREATMENT OF STRESS DISORDERS
449