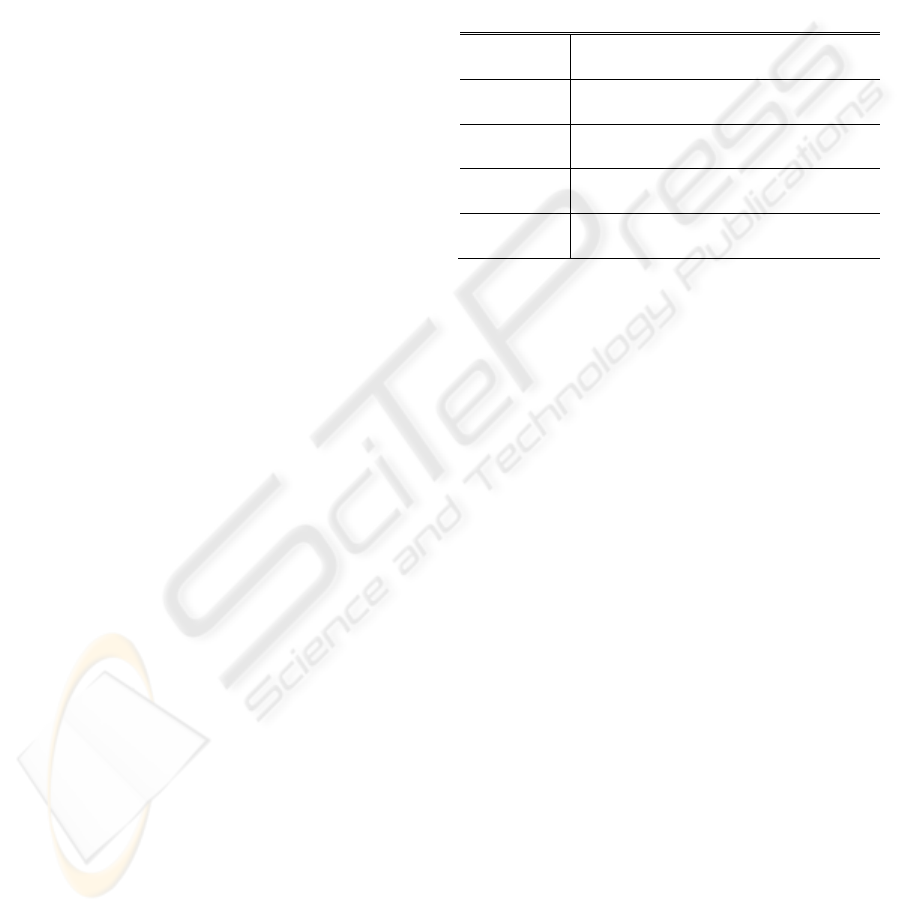
True Positive (TP): a-wave has been classified as a-
wave.
False Negative (FN): a-wave has been missed.
False Positive (FP): Non a-wave classified as a-
wave.
The sensitivity Se is the percentage of true a-
waves that were correctly detected by the algorithm.
The positive predictivity +P is the percentage of
detected a-waves which are real a-waves.
Table I shows the result of a-waves detection in
27 different records of collected SDPTG before
exercise and after each of the three exercise periods,
containing a total of 3332 heart beats.
As shown in Table 1, records which have
relatively irregular fast heart beats signals like A1-
after exercise 2 , irregular fast heart beats with low
amplitudes like B1-after exercise 2, and non-
stationary SDPTG with irregular fast heart beats and
low amplitudes like E3-after exercise 3 contain a
few false positives (FP).
The number of false negatives (FN) was zero. The
overall average sensitivity for a-waves detection was
100% and the positive predictivity was 99.88%.
4 DISCUSSION
The major reason for the interest in measuring heart
rate variability stems from its ability to predict
survival after a heart attack. In ECG signals analysis,
the interval between adjacent QRS complexes is
termed as the normal to normal (NN) or the R to R
(RR) interval. Heart rate variability (HRV) refers to
the beat-to-beat alterations in heart rate. The results
of a HRV analysis portray the physiological
condition of the patient and are an important
indicator of cardiac disease. Many studies have
shown that reduced HRV predicts sudden death in
patients.
The detection of R peak is the main step to
measure HRV. Precise R-R interval calculations are
necessary to accurately depict the physiological
state. (John, 2000) found that more than 26 different
types of arithmetic manipulations of R-R intervals
have been described in the literature to represent
HRV.
The Task Force of the European Society of
Cardiology and the North American Society of
Pacing and Electrophysiology (Task Force of the
European Society of Cardiology and the North
American Society of Pacing and Electrophysiology,
1996) suggest a number of simple time domain
measures to estimation HRV. It has been discussed
in their paper that the HRV is calculated using the
mean the standard deviation of the length of the
cardiac cycle. This can be determined using either
the R-R intervals of a short ECG segment or the. a-a
intervals With these methods either the heart rate or
the a-a intervals a and SDPTG signal. Table 2 shows
some simple time–domain HRV variables: MAX-
MIN, SDNN, RMSSD, and SDSD that can be
calculated based on SDPTG signals.
Table 2: HRV Statistical Variables.
variable Statistical measurement
MAX-MIN
Difference between shortest and longest a-a
interval
SDNN Standard deviation of all a-a intervals
RMSSD
Root mean square of the difference of
successive a-a intervals
SDSD
Standard deviation of of differences between
adjacent a-a intervals
5 CONCLUSIONS
The second derivative of the photoplethysmogram
(SDPTG) can be used to calculate heart rate
variability provided the a-waves can be detected
accurately. Therefore, we propose an algorithm to
detect a-waves in SDPTG signals with a high
frequency noise, low amplitude, non-stationary
effects, irregular heart beat and after exercise. It
achieved an overall average sensitivity for a-waves
detection 100% and a positive predictivity was
99.88%. over 27 records, containing a total of 3370
heart beats.
The accurate detection of a-waves in the SDPTG
offers a non-invasive method of evaluating cardiac
functioning. The usage of SDPTG can be useful for
HRV analysis and identification of individuals at
risk.
ACKNOWLEDGEMENTS
The authors would like to thank Aya Matsuyama for
collecting the data.
REFERENCES
Berntson, G., Jr Bigger, J., Eckberg, D., Grossman, P.,
Kaufmann, P., Malik, M., Nagaraja, H., Porges, S.,
BIOSIGNALS 2010 - International Conference on Bio-inspired Systems and Signal Processing
86