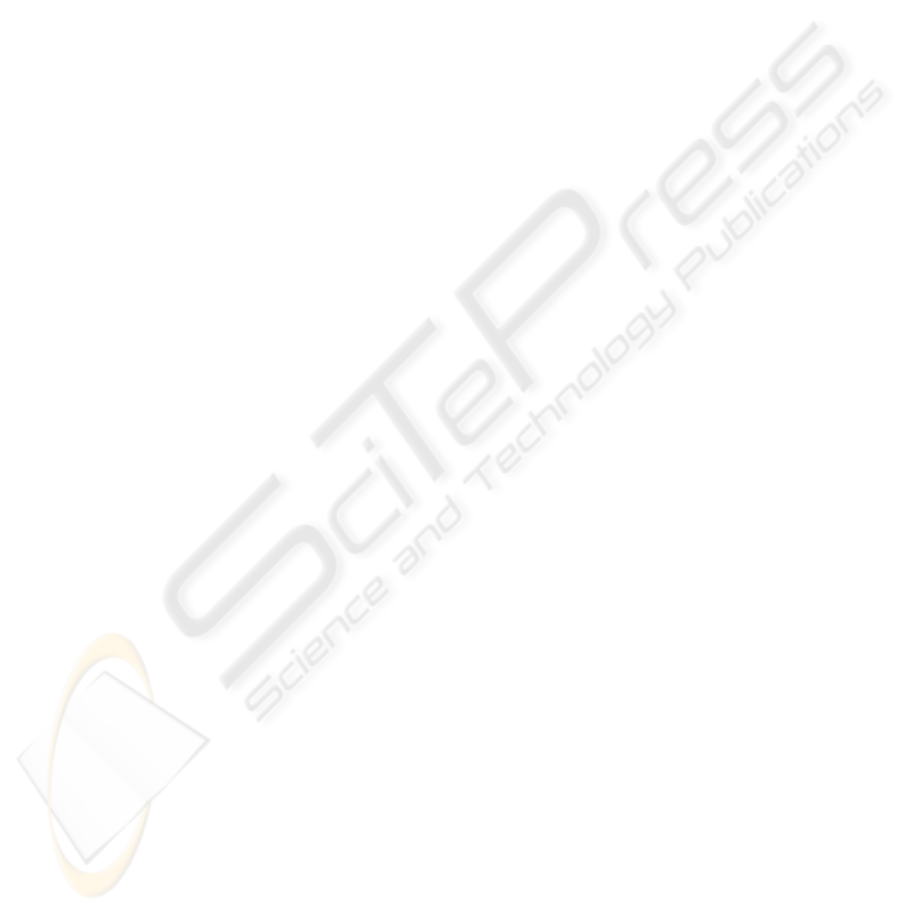
p. 13) write: ‘Mechanisms occur in nested hierar-
chies and the descriptions of mechanisms in neuro-
biology and molecular biology are frequently multi-
level. . . . lower level entities, properties, and activities
are components in mechanisms that produce higher
level phenomena’. That is, mechanisms involve inter-
nal structure, often several levels of internal structure.
The mechanisms of cancer involve just such hi-
erarchical levels. There is the level of the person,
their socioeconomic background, and their lifestyle
choices like diet, exercise and smoking. Then there is
the level of the tumour, particularly its growth rates,
containment, blood supply and so on. Then the cells
in the tumour have particular properties. Within the
cell itself, we are increasingly able to distinguish be-
tween the levels of gene expression, RNA and pro-
teins. All these levels affect the process of the cancer,
and the patient’s prognosis.
It is also recognised that explaining some phe-
nomenon requires finding the mechanism responsible
for that phenomenon using empirical work from mul-
tiple scientific fields. Craver discusses this with re-
gard to neuroscience: ‘The central idea is that neu-
roscience is unified not by the reduction of all phe-
nomena to a fundamental level, but rather by using
results from different fields to constrain a multilevel
mechanistic explanation’—see (Craver, 2007, p. 231).
See also (Russo, 2008) on social mechanisms, and
(McKay-Illari and Williamson, 2009) on explanation
for the spread of HIV. We can call these multi-field
mechanisms as they are modelled on the basis of dif-
ferent kinds of data, for instance molecular, genetic,
chemical, and environmental.
Treating cancer involves integrating information
from multiple fields, each studying one of the hierar-
chical levels we have described. Socioeconomic, clin-
ical, genomic, transcriptomic and proteomic data are
all known to be relevant to therapy choice and prog-
nosis.
Recursive Bayesian nets are legitimate descrip-
tions of physical mechanisms, provided that intra-
and inter-level relations are compatible with avail-
able theoretical knowledge. If so, RBN intra-level
(causal) relations among peer variables stand for
mechanisms and RBN inter-level relations stand for
decompositions of network variables into constituent
sub-mechanisms. Accordingly, simple and network
variables can model entities (or sets of these entities)
in their various states; and RBN causal relations can
model interactions and influences among these enti-
ties, i.e., activities.
Current philosophical work on biological mech-
anisms tends to cover purely qualitative aspects
of mechanisms ((Russo, 2008) discusses, however,
quantitative modelling of social mechanisms). Our
work allows the possibility of adding a quantitative
dimension: the probabilities within an RBN quan-
tify the strengths of the causal connections and lead
to a joint probability distribution over all the vari-
ables in the network. Such a quantitative descrip-
tion of the mechanism is vital for the task of predic-
tion, which requires determining the outcomes that
are most probable given available evidence. Since
causal information is required for control and mech-
anistic information for explanation, the RBN formal-
ism offers the prospect of multiple uses—prediction,
explanation and control—as well as the capacity to
integrated different kinds of evidence and evidence
from different fields.
4 CANCER APPLICATION:
VARIABLES AND DATA
SOURCES
In the last decade the human genome project and tech-
nological breakthroughs such as those in microarray
technology and mass spectrometry-based proteomics
have had a big impact on cancer research. They have
led to a vast increase in available data, from genomics,
transcriptomics, proteomics and metabolomics. Tra-
ditional diagnostics is under strain, and there is in-
creasing need for biomedical decision support tools.
Bayesian nets are an obvious choice for such tools,
but the prospect of being able to include hierarchy in
such Bayesian nets is exciting for cancer research. Hi-
erarchy is important to cancer research since it is still
unclear how the DNA, RNA, protein and metabolic
levels interact to produce cancer and affect prognosis.
Our formalism can be applied to build a model
using TCGA data (clinical patient data) and NCI-60
(cell line data) integrating the following variables:
Top level: Clinical. The top level includes the fol-
lowing recursive variables. The first recursive vari-
able kind of tumour. (Since most studies are focused
on single tumour types, only information on sub-types
will be available in a single study.) Each different sub-
type of tumour is a value of this variable, each value
corresponds to a lower-level Bayesian network of de-
pendencies between gene expression levels. If data is
available, a second important recursive variable will
be metastasis. Metastasis present/absent will corre-
spond to lower-level nets of gene expression levels.
Finally, it is proposed that the model will also include
standard simple variables at the clinical level such as
therapy, age, and survival in months.
BIOINFORMATICS 2010 - International Conference on Bioinformatics
236