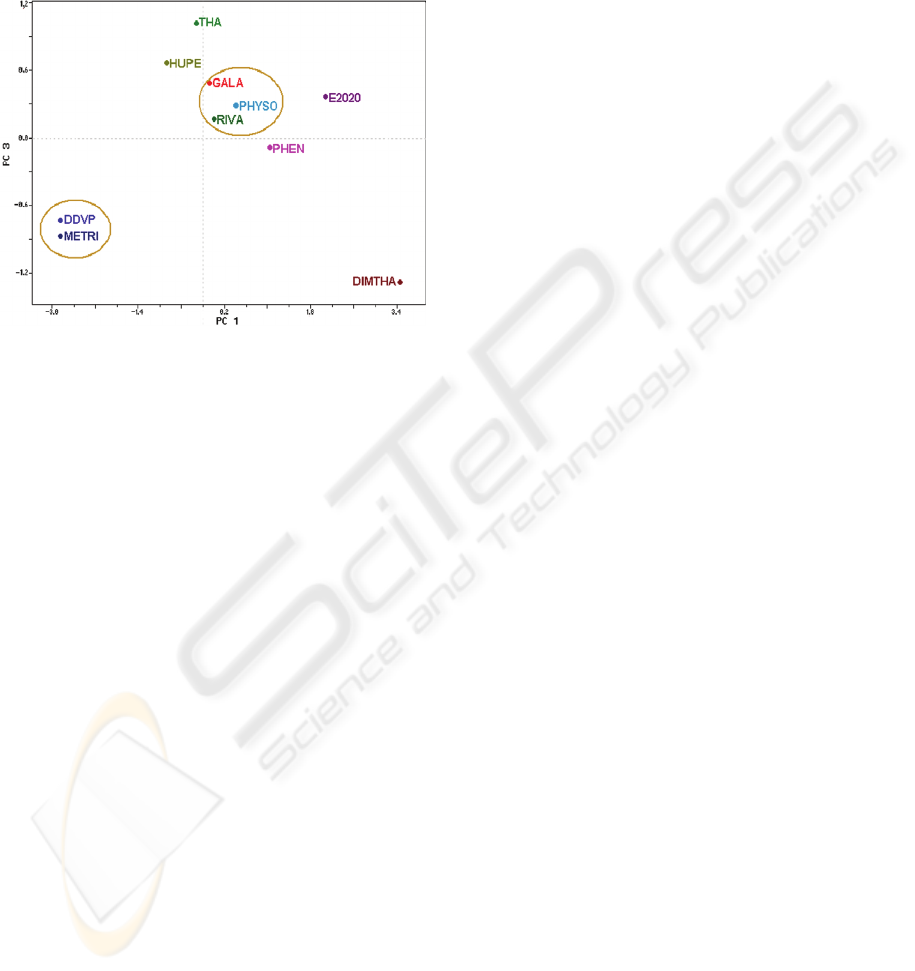
Figure 4 shows PC1 versus PC3 scores plot.
There are two patterns in PC3, i.e., DDVP / METRI
and GALA / PHYSO / RIVA. Which can be
explained, since PC3 is dominated by the orbital
energy of HOMO-1 (+0.79272) (see Equation 3).
These molecules have values close to the HOMO-1
and the molecular volume (Table 1).
Figure 4: PC1 versus PC3 scores at level B3LYP/6-
31+G(d,p).
As can be seen in Figures 3 and 4, the equations
generated in PCs indicate that the electronic
properties are the most significant in the AChEI
molecules study, such as energy of HOMO-1. The
structural parameters that also contribute are:
molecular volume, size of the drug and H-H
distance.
4 CONCLUSIONS
The PCA study showed that electronic property, –
HOMO-1 orbital energy, logP, numbers of aromatic
ring, and structural parameters - volume, drug size
and H-H - are the most significant properties, i.e.,
the principal components of the AChEI drugs
pharmacophforic profile.
Thus, it is estimated that a good candidate to
inhibit the acetylcholinesterase enzyme must
includes: partition coefficient values between 0.8
and 4.9; logS between -5.0 and -1.5; polar surface
area between 30.0 and 60.0 Å
2
. The torsional
degrees number of freedom sufficient to be able to
rearrange itself adequately inside the AChE active
site is also important. Other desirable features for the
AChEI molecules are: preferably aromatic systems
or groups that simulate surface electron density of
aromatic systems; sufficient amount of hydrogen
acceptors and few donors of hydrogen. Furthermore,
according to B3LYP/6-31+G(d,p) level results the
inhibitor should have: HOMO-1 orbital energy
between -8.60 and -6.00 eV; and the distance among
the two more acidic hydrogens molecule between
1.600 – 2.500 Å. Together, all these properties
participate in the pharmacophoric profile of the
studied AChEIs molecules.
ACKNOWLEDGEMENTS
This study is supported by CNPq, and Funpe/UnB.
We also acknowledge the computational resource of
CENAPAD/SP.
REFERENCES
Alcaro, S., Scipione, L., Ortuso, F., Posca, S., Rispoli, V.
& Rotiroti, D. (2002). Molecular modeling and
enzymatic studies of the interaction of a choline
analogue and acetylcholinesterase. Bioorganic &
Medicinal Chemistry Letters, 12, 2899-2905.
Anderson, T. W. 1984. An Introduction to Multivariate
Statistical Analysis. John Wiley & Sons. New York, 2nd
edition.
Camps, P. (2002). Cholinergic Drugs in Pharmacotherapy of
Alzheimer’s Disease. Mini Reviews in Medicinal
Chemistry, 2, 11-25.
de Paula A.A.N., Martins J.B.L., dos Santos, M.L.,
Nascente, L. de C., Romeiro, L.A.S., Áreas, T.F.M.A.,
Vieira, K.S.T., Gambôa, N.F., Castro, N.G., Gargano,
R. (2009). New potential AChE inhibitor candidates.
European Journal of Medicinal Chemistry, 44, 3754–
3759.
Francis, P. T. (1999) The cholinergic hypothesis of
Alzheimer’s disease: a review of progress. J Neurosurg
Psychiatry, 66, 137-147.
Frisch, M. J., G.W. Trucks, H.B.S., G.E. Scuseria, M.A. Robb,
J.R.C., Zakrzewski, V.G., Montgomery, Jr. J.A., Stratmann,
R.E., Dapprich, J.C.B.S., Millam, J.M., Daniels, A.D., Strain,
K.N.K.M.C., Farkas, O., Tomasi, J., Barone, V., Cammi,
M.C.R., Mennucci, B., Pomelli, C., Adamo, C., Clifford, S.,
Petersson, J.O.G.A., Ayala, P.Y., Cui, Q., Morokuma, K.,
Rabuck, D.K.M.A.D., Raghavachari, K., Foresman, J.B.,
Ortiz, J.C.J.V., Stefanov, B.B., Liu, G., Liashenko, A.,
Komaromi, P.P.I., Gomperts, R., Martin, R.L., Fox D.J., Al-
Laham, T.K.M.A., Peng, C.Y., Nanayakkara, A., Gonzalez,
C., Challacombe, M., Gill, P.M.W., Johnson, B., Chen, W.,
Wong, M.W., Andres, J.L., Gonzalez, C., Head-Gordon, M.,
Replogle E.S. And Pople, J.A. (2003). Gaussian03 (Rev.
A11) Pittsburgh, PA, Gaussian Inc.
Gnanadesikan, R. 1997. Methods for statistical data analysis of
multivariate observations. John Wiley & Sons. New York,
2nd edition.
BIOINFORMATICS 2010 - International Conference on Bioinformatics
248