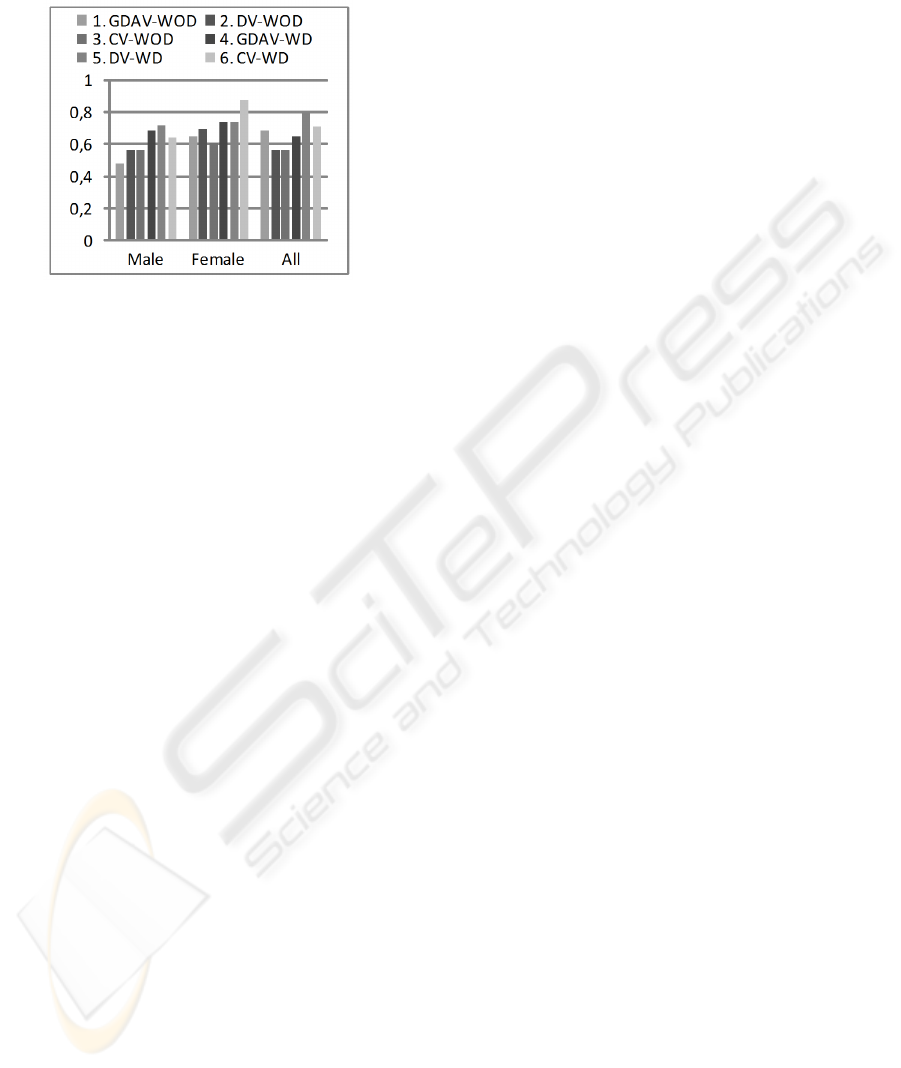
50% accuracy (on male radiographs), the average ac-
curacy is around 55% with peaks close to 70%.
Figure 7: Accuracy obtained with different classifiers with
and without using discrimination matrix.
Regarding discrimination methods, the degree of
accuracy achieved is clearly better, highlighting the
classification of female radiographs again. In most
cases, voting methods perform better than GDAV
method. It is remarkable that with the appropriate
threshold, the CV method classifies women radio-
graphs with success rates very close to 90% of accu-
racy. With all the radiographs the best is DV method,
reaching an accuracy near to 80%. In general, the av-
erage accuracy is around 70% considering all cases.
5 CONCLUSIONS
As a main general conclusion, the utilization of the
LBP texture analysis operator has emerged as an ef-
fective means for the study of radiographic images,
and more particularly chest radiographs, which are
the focus of this paper. LBP is a method that collects
interesting advantages as a small number of features
and a high power of discrimination.
The use of template matching technique at the
stage of segmentation to locate the lungs has been
satisfactory, despite its known limitations. The great
variability among individuals in terms of shape and
size of the lungs makes it difficult to achieve an opti-
mal location of these structures.
Our approach to the problem has been imple-
mented and tested as a first step towards a system
for the detection of normality and pathology in radio-
graphs which can be implanted in a hospital. The ra-
tios of success achieved, near 90% in the best cases,
are quite promising, although the method designed
should not be considered closed. Clearly, higher accu-
racy rates should to be obtained to achieve a practical
CAD system. To work in its development the use of
much larger sets of test will be essential.
ACKNOWLEDGEMENTS
This work has been supported by the Spanish MEC
and European FEDER grants TIN2006-15516-C04-
03 and Consolider CSD2006-00046.
REFERENCES
Campadelli, P. and Casiraghi, E. (2005). Lung Field Seg-
mentation in Digital Postero-Anterior Chest Radio-
graphs. In PRIA, volume 3687 of LNCS, pages 736–
745. Springer Heidelberg.
Fleckenstein, P. and Tranum-Jensen, J. (2001). Anatomy in
Diagnostic Imaging. Blackwell Science, 2 edition.
Hao, B., Leung, C. K.-S., Camorlinga, S., Reed, M. H.,
Bunge, M. K., Wrogemann, J., and Higgins, R. J.
(2008). A Computer-Aided Change Detection Sys-
tem for Paediatric Acute Intracranial Haemorrhage. In
C3S2E ’08, pages 109–111, New York. ACM.
Iakovidis, D. K., Keramidas, E. G., and Maroulis, D.
(2008). Fuzzy Local Binary Patterns for Ultrasound
Texture Characterization. In IAR, volume 5112 of
LNCS, pages 750–759. Springer Heidelberg.
Kallergi, M. (2005). Evaluation Strategies for Medical-
Image Analysis and Processing Metodologies. In
Medical Image Analysis Methods, chapter 12, pages
433–471. CRC Press.
Ojala, T., Pietik¨ainen, M., and Harwood, D. (1996). A Com-
parative Study of Texture Measures with Classifica-
tion Based on Feature Distributions. Pattern Recogni-
tion, 29(1):51–59.
Oliver, A., Llad, X., Freixenet, J., and Mart, J. (2007). False
Positive Reduction in Mammographic Mass Detection
Using Local Binary Patterns. In MICCAI ’07, volume
4791 of LNCS, pages 286–293. Springer Heidelberg.
Papadopoulos, A. N., Plissiti, M. E., and Fotiadis, D. I.
(2005). Medical-Image Processing and Analysis for
CAD Systems. In Medical Image Analysis Methods,
The Electrical Engineering and Applied Signal Pro-
cessing Series, chapter 2, pages 51–86. CRC Press,
Boca Raton, FL, USA.
Park, M., Jin, J. S., and Wilson, L. S. (2004). Detection
of Abnormal Texture in Chest X-rays with Reduction
of Ribs. In VIP ’05, pages 71–74, Darlinghurst, Aus-
tralia. Australian Computer Society, Inc.
Sørensen, L., Shaker, S. B., and de Bruijne, M. (2008).
Texture Classification in Lung CT Using Local Bi-
nary Patterns. In MICCAI ’08, volume 5241 of LNCS,
pages 934–941. Springer Heidelberg.
BIOINFORMATICS 2010 - International Conference on Bioinformatics
172