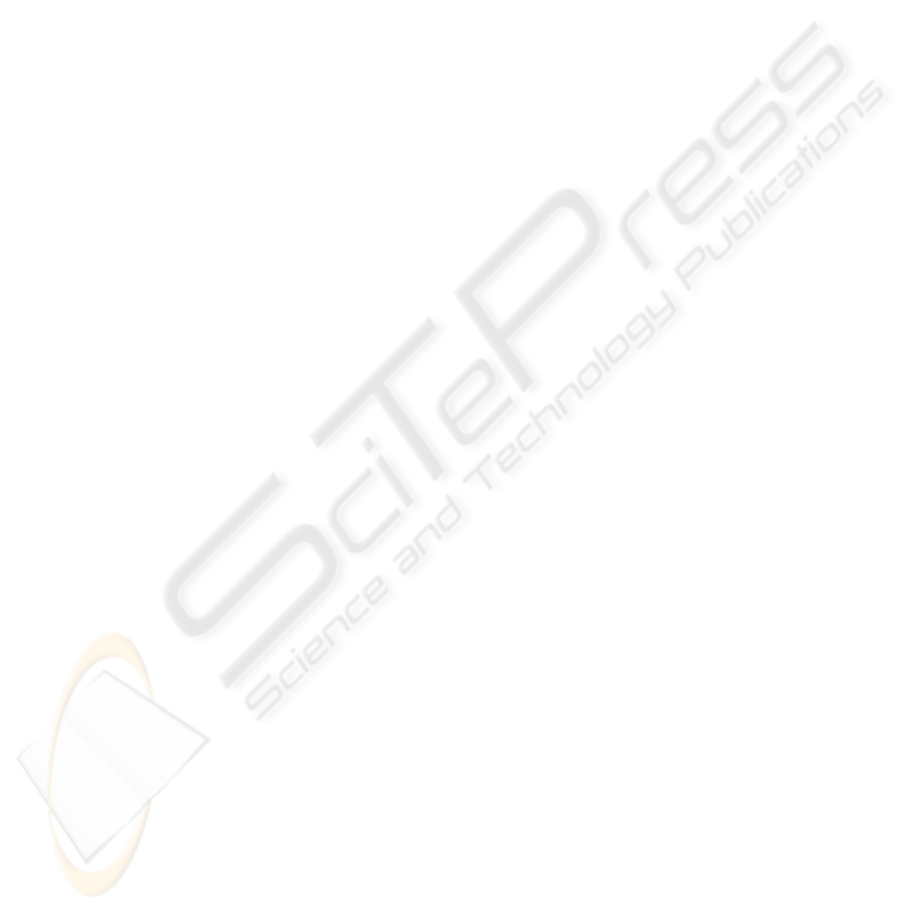
continues and the value for the safety speed limit is re-
duced to slowSpeed at the same time, the unsafe point
of this pattern is reached (see Figure 2 for an exam-
ple). Table 4 contains the qualitative definition of the
pattern.
4.2.2 Pattern 2
In four of the 21 situations we have identified a be-
haviour pattern, in which the subject kept driving to-
ward his/her normal side if possible, in order to avoid
obstacles that may occur at his/her deficit side. If
the user tries to avoid an obstacle located on the nor-
mal side by steering the wheelchair to the other side,
but does not notice an obstacle located on the deficit
side, an intervention of the safety assistant is neces-
sary to avoid a collision with the obstacle located on
the deficit side; see Table 4 for its specification. In
this pattern, the change of the critical point to the un-
safe one is caused predominantly by the change of the
joystick direction from the normal side to the deficit
side.
4.2.3 Pattern 3
The third pattern describes situations in which the
subject commanded the wheelchair to carry out an
obstacle avoidance maneuver by driving backwards,
however the safety module intervened to avoid a col-
lision with an obstacle located in the back of the
wheelchair; see Table 4 for its definition. In this pat-
tern the rotational direction of the wheelchair is in-
significant. This pattern is found in 3 out of 21 situa-
tions.
5 DISCUSSION
The three behaviour patterns cover 19 of 21 situations,
but the remaining two situations cannot be identified
by any reasonable pattern.
The related video material exhibited that the sub-
jects tried to apply several different steering com-
mands to navigate the wheelchair through the narrow
obstacle configuration. These changes of driving di-
rections in a short temporal sequence led to unpre-
dictable orientations of the caster front-wheels. Al-
though the intended steering commands are appro-
priate at that moment, the wheelchair could not fol-
low the subject’s commands in time. The subjects
were confused by the wheelchair’s behaviour, since
they were not aware of the position of the caster
front-wheels. As a consequence, they behaved unpre-
dictably. This phenomenon implies the existence of a
mode confusion, in which the wheelchair is situated in
a state different from the one expected by the subject.
Mode confusions occur typically in shared-control
systems (Thimbleby, 1990), such as the wheelchair.
During the experiment we observed another mode
confusion phenomenon: almost all subjects acted un-
common after the intervention of the safety assistant:
they attempted to regain control over the wheelchair,
but the wheelchair did not drive in the way they ex-
pected; as a result they tried to give the wheelchair
some arbitrary commands via the joystick or pressed
the joystick powerfully, which caused an even less ex-
pected movement of the wheelchair. Mode confusion
situations can be avoided through the improvement of
the system’s behaviour or providing the user with suit-
able clarification (Thimbleby, 1990).
An interesting application of the critical patterns
developed in Section 4 could be to support the re-
habilitation process of neglect and hemianopsia pa-
tients with cognitive and motor deficits. These pat-
terns enable the wheelchair to inform the user about a
possible collision with an approaching obstacle in ad-
vance, which would otherwise be noticed too late or,
even worse, ignored due to their spatial perceptional
problems. Instead of the current feedback from the
wheelchair, i.e., the disregard of the user’s commands
given via the joystick and the braking process in un-
safe situations, some acoustic signal, for example,
could be used to inform the user of an approaching
obstacle on his/her impaired side. As a consequence
the user could be trained to drive the wheelchair more
smoothly or even without the safety module in a nar-
row environment.
On the other hand, the behaviour patterns provide
us with helpful information to improve the existing
safety assistant. In our data we counted up to 5600
interventions in a single test run by some participants,
which is inconvenient in real life in spite of the guar-
anteed safeness. As a possible solution, the safety as-
sistant can be extended with a module that modifies
the joystick commands if a situation matching an un-
safe pattern has been detected, to compensate users’s
visual deficits, and reduce the number of the interven-
tions of the safety assistant.
Finally, the current work makes it clear that user
studies with an intelligent living assistant and real
users present us with hard challenges. They require
close cooperation of engineers, neuropsychologists,
and other medical professionals. In addition to the ex-
periment design, test environment construction, real-
ization, data analysis and user modelling, the accred-
itation of a homogeneous user group turned out to be
the most difficult one, at least if the group consists of
persons with specific cognitive or physical deficits. In
HEALTHINF 2010 - International Conference on Health Informatics
334