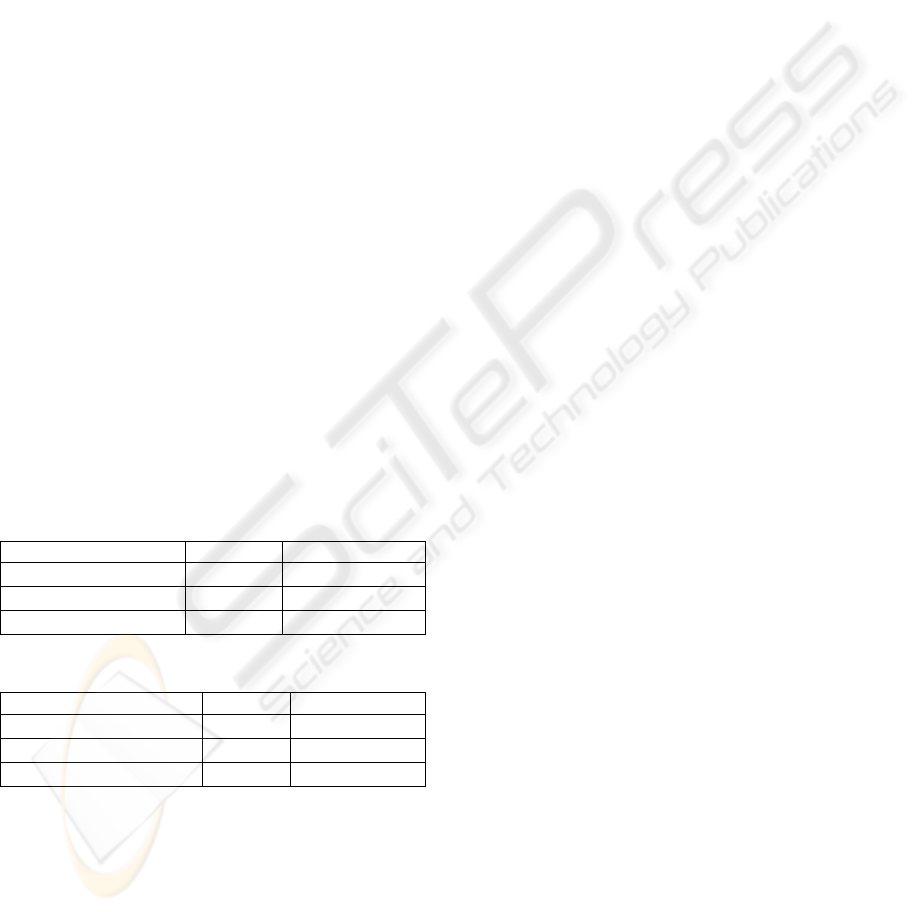
3.1 Entropy in AR and ER Results
The quantification of conflict and non-specificity in
AR and ER results are demonstrated here using the
examples provided in Section 2. Using Equation 6
the conflict involved in the ER result
F1(effectiveness of physical inventory):[0, 0.1, 0.9,
0], is calculated as.
0.1log
0.1
0.9log
0.9 0.469
The non-specificity involved in the ER result is
calculated using Equation 10.
N
|
2
|
1
Using Equation 8 the conflict involved in the AR
result S1(risk): [0, 0, 0.57, 0, 0], is calculated as
follows. Recall that the membership of the
complement is determined from Equation 2.
0.57log
0.57
0.43log
0.43 0.9858
The non-specificity involved in the AR result is
calculated using Equation 10.
N
|
1
|
0
In addition to the ER and AR example provided
previously two additional ER results and AR results
are provided. The ER and AR results and their
quantities of information uncertainty are presented
in Tables 4 and 5, respectively.
Table 4: ER entropy results for Effectiveness of Physical
Inventory example.
ER result Conflict Non-specificity
F1[0, 0.1, 0.9, 0] 0.469 1
F2[0, 0.2, 0.8, 0] 0.722 1
F3[0, 0.15, 0.75, 0.1] 1.054 1.585
Table 5: AR Entropy results for Economic Risk example.
AR result Conflict Non-specificity
S1[0, 0, 0.57, 0, 0] 0.9858 0
S2[0, 0.3, 0.7, 0.2, 0] 2.883 1.585
S3[0, 0.2, 0.6, 0.2, 0.1] 2.484 2.000
The results demonstrate the utility of quantifying
information uncertainty to compare the results. In
Table 4, the effectiveness of physical inventory, F1,
F2 and F3 all result in a linguistic value as “mostly
moderate”. There is an observable difference in each
resulting vector; however, a realistic comparison is
not possible without a useful metric. Entropy
measures, specifically conflict, provide a
recognizable and comparable difference with all
three ER results. In the case of the AR results, Table
5, there is also a recognizable difference in the
conflict and the non-specificity. The non-specificity
reflects a difference that can also be discerned
visually, i.e. the greater number of non-zero
alternatives the greater the non-specificity.
Alternatively, measuring the conflict provides
comparative information that is not as easily
discerned visually.
Tables 4 and 5 illustrate the quantification of
the conflict and non-specificity using simple AR and
ER models. Based on information uncertainty, the
alternative with the least information uncertainty is
also the alternative with the most confidence.
Therefore, F1 and S1 are the alternatives providing
the most confidence.
4 CONCLUSIONS
ER and AR results for SRAMA have quantifiable
amounts of information uncertainty and this study
extends information theory to AR and ER SRAMA
models. Straight-forward extensions of previous
approaches are presented in this paper and used to
quantify the information uncertainty in AR results.
The information uncertainty measurements of
conflict and non-specificity associated with AR and
ER results are illustrated and used to compare the
results to one another. Maximum confidence is
simply based on minimum measured information
uncertainty in each result. Through ongoing
research, the results can be further extended through
the development of a metric comparing measured
confidence to the maximal potential value of
confidence determined from a combined measure of
information uncertainty. Moreover, future work will
involve comparisons of the results obtained using
the proposed metrics to rank the results to those
obtained from a SME ranking of the results.
REFERENCES
Barret, J., and Woodall, W.: A Probabilistic Alternative to
Fuzzy Logic Controllers. IEEE Transactions Vol. 29,
459-467 (1997)
Bott, T. F., Eisenhawer, S. W.: Risk Assessment When
Malevolent Actions Are Involved, Proc. of the RAMS
’06. Annual Reliability and Maintainability Sym-
posium, 2006. Washington, DC USA.
Chavez, G. M.: On Fusing Linguistic and Assignment
Uncertainty in Damage Assessment of Structures,
Diss, University of New Mexico, Dec. 2007
ICAART 2010 - 2nd International Conference on Agents and Artificial Intelligence
404