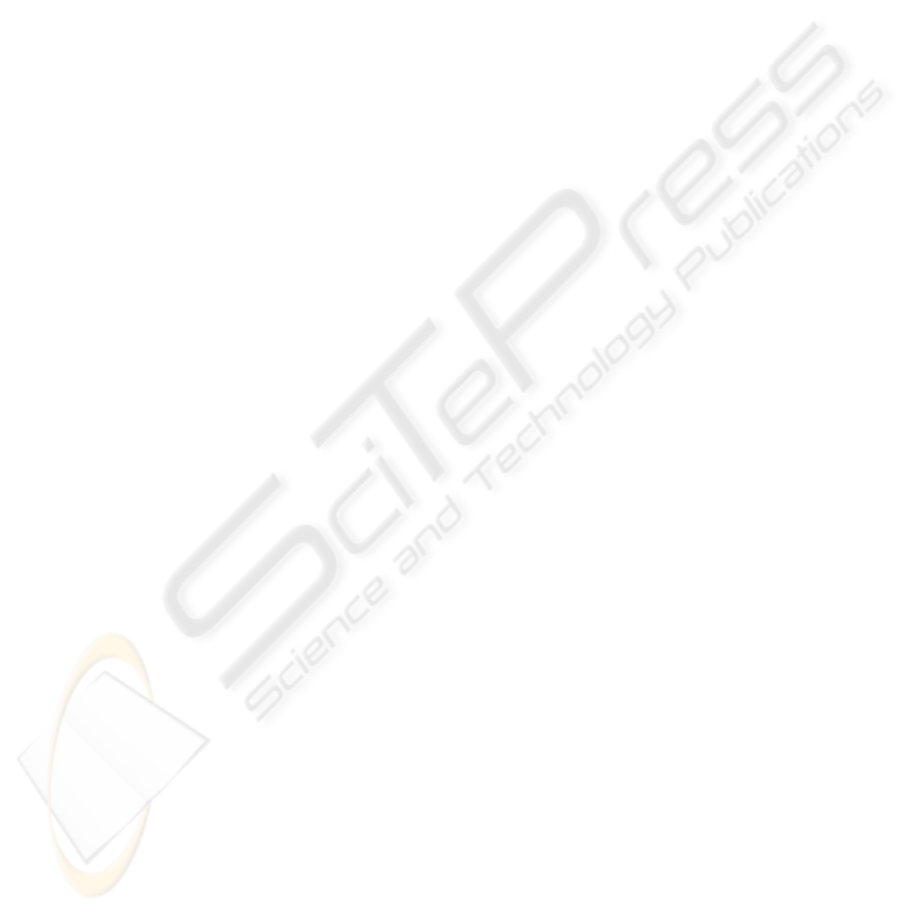
presented APE, a novel adaptive load balancing al-
gorithm, which includes an hierarchical aggregation
of the results found in each worker. APE proved to
be an acceptable and scalable solution for the set of
representative networks studied, successfully reduc-
ing the time needed to calculate the subgraph census
and achieving larger subgraph sizes than were before
possible.
The main drawback of APE seems to be the fi-
nal aggregation of results. We plan to research and
improve this step in the future. One way of doing it
would be to use a more compact and compressed rep-
resentation of the results. We also plan to research
the splitting threshold parameter in order to better un-
derstand on what does it depend, exactly how does it
affect the computation and how could it be automati-
cally determined by the algorithm. We are collaborat-
ing with neuroinformatics scientists in order to apply
the described strategies on real neural networks to ob-
tain new and interesting results on previously unfea-
sible subgraph census.
ACKNOWLEDGEMENTS
We thank Enrico Pontelli for the use of Inter Clus-
ter in the New Mexico State University. Pe-
dro Ribeiro is funded by an FCT Research Grant
(SFRH/BD/19753/2004). This work was also par-
tially supported by project CALLAS of the FCT (con-
tract PTDC/EIA/71462/2006).
REFERENCES
Albert, I. and Albert, R. (2004). Conserved network mo-
tifs allow protein-protein interaction prediction. Bioin-
formatics, 20(18):3346–3352.
Alm, E. and Arkin, A. P. (2003). Biological networks. Cur-
rent Opinion in Structural Biology, 13(2):193–202.
Barabasi, A. L. and Albert, R. (1999). Emergence of scaling
in random networks. Science, 286(5439):509–512.
Bordino, I., Donato, D., Gionis, A., and Leonardi, S.
(2008). Mining large networks with subgraph counting.
In Procs of the 8th IEEE International Conference on
Data Mining (ICDM), pages 6 pp.+.
Bu, D., Zhao, Y., Cai, L., Xue, H., Zhu, X., Lu, H., Zhang,
J., Sun, S., Ling, L., Zhang, N., Li, G., and Chen, R.
(2003). Topological structure analysis of the protein-
protein interaction network in budding yeast. Nucl. Acids
Res., 31(9):2443–2450.
Costa, L., Rodrigues, F. A., Travieso, G., and Boas, P. R. V.
(2007). Characterization of complex networks: A survey
of measurements. Advances In Physics, 56:167.
Eager, D. L., Lazowska, E. D., and Zahorjan, J. (1986).
Adaptive load sharing in homogeneous distributed sys-
tems. IEEE Trans. Softw. Eng., 12(5):662–675.
Faust, K. (2007). Very local structure in social networks.
Sociological Methodology, 37(1):209–256.
Hall, L. A. (1997). Approximation algorithms for schedul-
ing. In Approximation algorithms for NP-hard problems,
pages 1–45, Boston, MA, USA. PWS Publishing Co.
Heymann, E., Senar, M. A., Luque, E., , and Livny, M.
(2000). Evaluation of an adaptive scheduling strategy
for master-worker applications on clusters of worksta-
tions. In Proc. of the 7th International Conference on
High Performance Computing, Bangalore, India.
Itzkovitz, S., Levitt, R., Kashtan, N., Milo, R., Itzkovitz,
M., and Alon, U. (2005). Coarse-graining and self-
dissimilarity of complex networks. Phys Rev E Stat Non-
lin Soft Matter Phys, 71(1 Pt 2).
Jeong, H., Tombor, B., Albert, R., Oltvai, Z. N., and
Barab
´
asi, A. L. (2000). The large-scale organization of
metabolic networks. Nature, 407(6804):651–654.
Kashtan, N., Itzkovitz, S., Milo, R., and Alon, U. (2004).
Efficient sampling algorithm for estimating subgraph
concentrations and detecting network motifs. Bioinfor-
matics, 20(11):1746–1758.
Kondoh, M. (2008). Building trophic modules into a per-
sistent food web. Proceedings of the National Academy
of Sciences, 105(43):16631–16635.
Kuramochi, M. and Karypis, G. (2001). Frequent subgraph
discovery. IEEE International Conference on Data Min-
ing, 0:313.
Matias, C., Schbath, S., Birmel, E., Daudin, J.-J., and
Robin, S. (2006). Network motifs: mean and variance
for the count. REVSTAT, 4:31–35.
Mazurie, A., Bottani, S., and Vergassola, M. (2005). An
evolutionary and functional assessment of regulatory net-
work motifs. Genome Biology, 6:R35.
McKay, B. (1981). Practical graph isomorphism. Congres-
sus Numerantium, 30:45–87.
Middendorf, M., Ziv, E., and Wiggins, C. (2004). Infer-
ring network mechanisms: The drosophila melanogaster
protein interaction network. PNAS, 102:3192.
Milo, R., Shen-Orr, S., Itzkovitz, S., Kashtan, N.,
Chklovskii, D., and Alon, U. (2002). Network motifs:
simple building blocks of complex networks. Science,
298(5594):824–827.
Nijssen, S. and Kok, J. N. (2004). Frequent graph mining
and its application to molecular databases. In SMC (5),
pages 4571–4577. IEEE.
Picard, F., Daudin, J.-J. J., Koskas, M., Schbath, S., and
Robin, S. (2008). Assessing the exceptionality of net-
work motifs. J Comput Biol.
Schatz, M., Cooper-Balis, E., and Bazinet, A. (2008). Par-
allel network motif finding.
Schreiber, F. and Schwobbermeyer, H. (2004). Towards mo-
tif detection in networks: Frequency concepts and flexi-
ble search. In Proceedings of the International Workshop
on Network Tools and Applications in Biology (NET-
TAB04, pages 91–102.
BIOINFORMATICS 2010 - International Conference on Bioinformatics
64