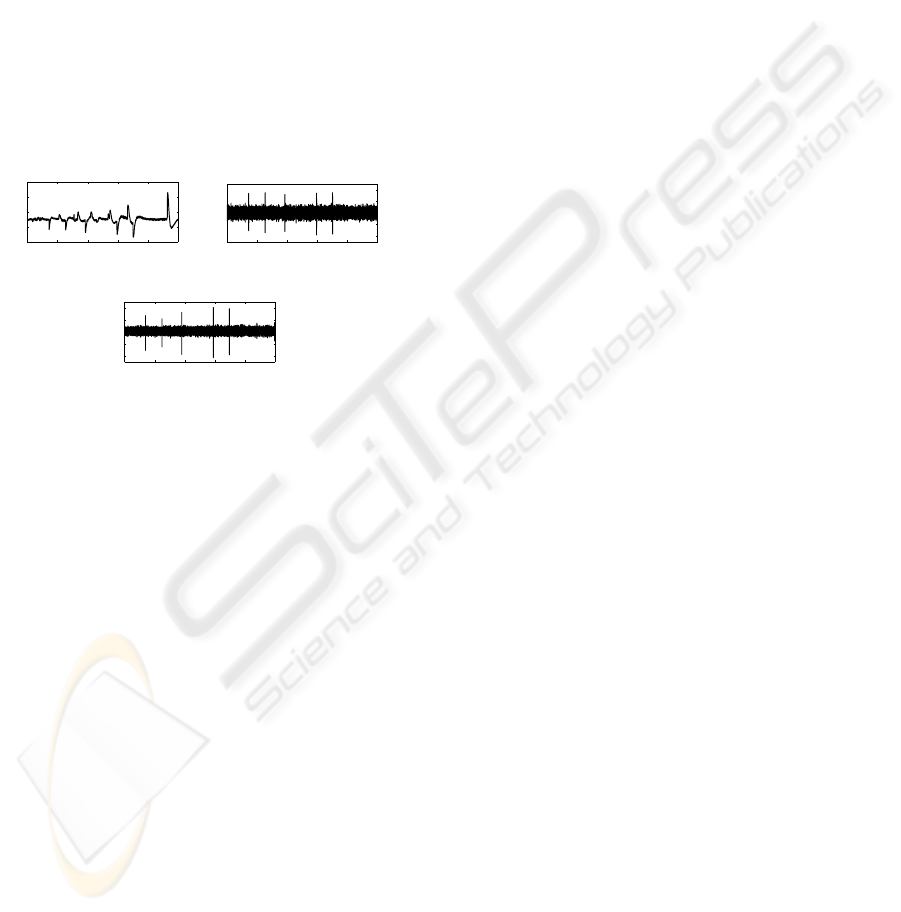
results, while the lower panel shows the frequency re-
sponse obtained by the filter test. As shown in the
first panel, the designed filter has a gain equal to 32dB
in bandwidth (900Hz −2.3kHz) while at 400Hz and
6kHz the attenuation of the signal is over 20dB. The
results of measurements show a lower gain (24dB)
and a band between 1.1kHz and 2kHz. The deviations
from the ideal behavior are due to manufacturing tol-
erances of the resistances and capacities used in the
feedback network of the filter. The selectivity of the
band-pass filter has been tested using as input for the
recording circuitry a sinusoidal signal at different fre-
quencies: 400Hz, 1.3kHz and 5kHz. The effect of the
band-pass filtering is evident in Fig. 6(b), where the
sine at 1.3kHz is allowed to pass. The signals shown
in Fig. 6(a) and in Fig. 6(c), prove how out-band fre-
quencies are completely filtered.
1520 1522 1524 1526 1528 1530
1.4
1.6
1.8
2
Time (s)
Amplitude (V)
(a) Input Signal
1520 1522 1524 1526 1528 1530
−0.8
−0.4
0
0.4
0.8
Time (s)
Amplitude (V)
(b) Matlab Filtered Signal
1520 1522 1524 1526 1528 1530
−0.8
−0.4
0
0.4
0.8
Time (s)
Amplitude (V)
(c) PCB Filtered Signal
Figure 7: Neural signal processing: filtering, amplification
and digital conversion.
Finally, the whole recording system has been tested in
its functionalities using stimuli supplied by the Scuola
Superiore di on the basis of recordings made in clini-
cal trials with rabbits. The input pattern represented in
Fig. 7(a) is the result of ten recording seconds during
which the rabbit was subjected to vibrations at 50Hz
and 100Hz in cutaneous afferents.
In a first phase of simulation, the available input
pattern of Fig. 7(a) has been processed with Matlab
using an ideal band-pass filter. In Fig. 7(b) the ideal
filtered signal obtained is shown. The heavy influ-
ence of low-frequencies noise has been deleted and
is clear the presence of the useful signal components
that in the input pattern were completely masked. The
filtered signal obtained with the implemented neural
recording circuitry, is represented in Fig. 7(c). The
slow components of the input signal coincide with
output peaks characterized by higher frequencies.
5 CONCLUSIONS
We have developed and tested a PCB system for neu-
ral activity recording and stimulation. Given the na-
ture of the signals to be acquired, special design tech-
niques for low noise and low power consumption have
been adopted. The first experimental results prove
that the system works correctly and stably, with the
possibility to acquire and process neural signal in mi-
crovolts order. The recording circuitry allows to filter
the neural signals in the band of 1kHz −2.2kHz, pro-
viding a programmable gain that covers values from
24dB to 44dB. The resulting signal can be digi-
tally converted and sent to PC for further processing
through USB or Wi-Fi transmission. The acquisition
system has been tested using pre-recorded neural pat-
terns extracted by rabbit with tfLIFE electrodes. The
system has been studied in order to generate also elec-
trical stimuli with controlled current amplitude, du-
ration and shape. This effort is part of an on-going
research program which aims to develop smart im-
plantable devices dedicated to neural activity record-
ing and stimulation.
REFERENCES
Gosselin, B. and Sawan, M. (2005). A low power portable
multichannel neural data acquisition system. Proceed-
ings of 10th Annual Conference of the International
FES Society, pages 391–393.
Jochum, T., Denison, T., and Wolf, P. (2009). Integrated cir-
cuit amplifiers for multi-electrode intracortical record-
ing. J. Neural Eng., 6.
Obeid, I., Nicolelis, M., and Wolf, P. (2004). A low power
multichannel analog front end for portable neural sig-
nal recordings. J. Neurosci. Meth., 133:27–32.
Salzman, C. D. and Newsome, W. T. (1994). Neural mech-
anisms for forming a perceptual decision. Science,
264:231–237.
Wang, Y., Wang, Z., L, X., and Wang, H. (2005). Fully
integrated and low power cmos amplifier for neural
signal recording. IEEE Engineering in Medicine and
Biology Society, pages 5250–5230.
Watkins, P., Kier, R., Lovejoy, R., Black, D., and Harrison,
R. (2006). Signal amplification, detection and trans-
mission in a wireless 100-electrode neural recording
system. pages 2193–2196.
Wessberg, Stambaugh, C., Kralik, J., Beck, P., Laubach,
M., Chapin, J., Kim, J., Biggs, S., Srinivasan, M., and
Nicolelis, M. (2000). Real-time prediction of hand
trajectory by ensembles of cortical neurons in primate.
Nature, 408(68102):361–365.
Yoshida, K., Hennings, K., and Kammer, S. (2006).
Acute performance of the thin-film longitudinal intra-
fascicular electrode. IEEE Conference Biomedical
Robotics and Biomechatronics, BioRob, pages 296–
300.
BIODEVICES 2010 - International Conference on Biomedical Electronics and Devices
214