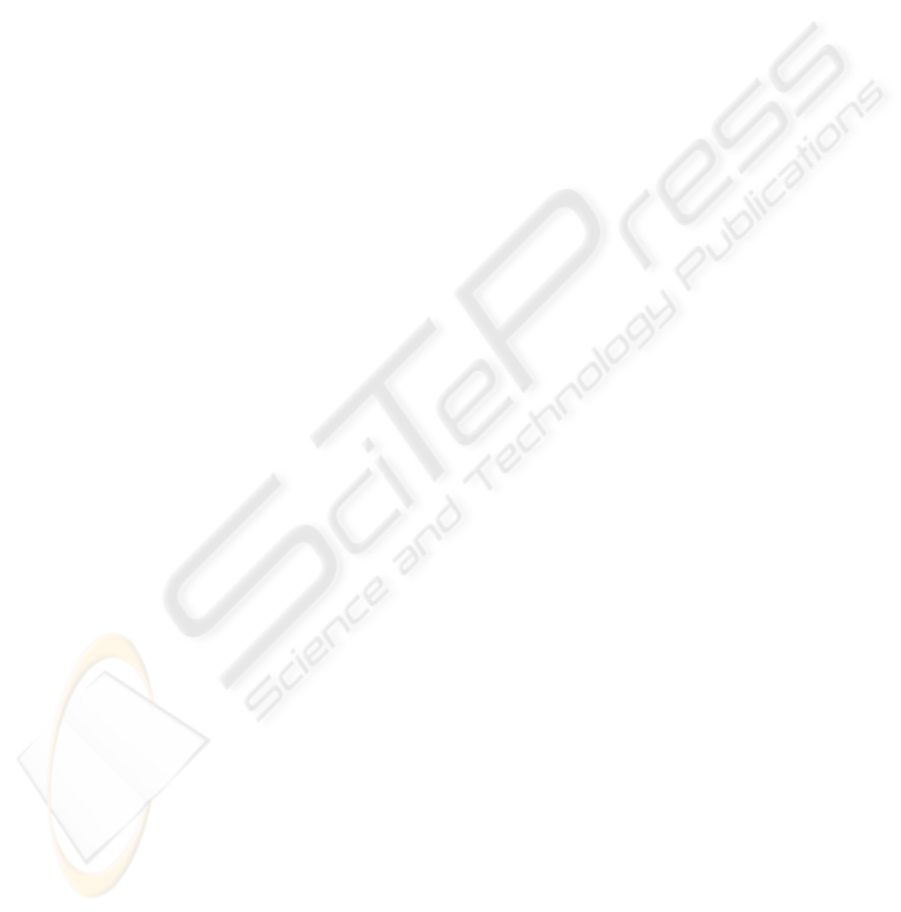
With these factors in mind we hope to work on
new systems of differential equations to model the
physical phenomena and also novel techniques to
solve these numerically. This work will enable a
comprehensive comparison of this DBN approach to
numerical simulations.
Future work will also include additional
validation of the model. We would like to validate
the model on a larger number of patients and
compare our methodology to other approaches.
4.2 Concluding Remarks
The system that has been presented in this paper,
which uses a Dynamic Bayesian Network approach
to modelling glycaemia in critically ill patients,
shows great promise. The system performs
extremely well in the context of great uncertainty,
sparse observations and limited system knowledge.
Our approach demonstrates a principled
technique for using standard real-time measurements
from ICU patients, to recalibrate model parameters
from general values to patient-specific values. This
model has the potential to be used by physicians to
individualise insulin dosage or to be incorporated
into a control system to automate insulin delivery.
The approach demonstrated here is applicable to
other applications where unseen variables must be
assessed and individualized in real-time.
Finally, the methodology introduced in this
paper, for mapping a system of differential equations
directly to a DBN, can be applied to other systems of
differential equations where all model terms vary,
and both continuous and sporadic temporal evidence
must be incorporated for an accurate solution.
ACKNOWLEDGEMENTS
We are grateful to the UHG Research Ethics
Committee for granting permission to extract
historical records from the database in the ICU of
University Hospital Galway. We acknowledge the
contributions of Dr Petri Piiroinen to the research
project overall and his feedback on this paper. This
research has been supported by Science Foundation
Ireland under grant 08/RFP/CMS1254, and by a
Marie Curie Transfer of Knowledge Fellowship of
the EU 6th Framework Programme contract CT-
2005-029611.
REFERENCES
Abkai, C. & Hesser, J., 2009. Virtual intensive care unit
(ICU): real-time simulation environment applying
hybrid approach using dynamic Bayesian Networks
and ODEs. Studies in Health Technology and
Informatics, 142, 1-6.
Aleks, N., Russell, S., Madden, M.G., Morabito, D.,
Staudenmayer, K., Cohen, M., Manley, G., 2008.
Probabilistic detection of short events, with
application to critical care monitoring. Proceedings of
NIPS 2008: 22nd Annual Conference on Neural
Information Processing Systems, Vancouver, Canada.
Andersen KE, Højbjerre M., 2003. A Bayesian approach
to Bergman’s minimal model. In C. M. Bishop and B.
J. Frey (eds), Proceedings of the Ninth International
Workshop on Artificial Intelligence and Statistics, Jan
3-6, 2003, Key West, FL.
Bergman, R.N., Phillips, L.S. & Cobelli, C., 1981.
Physiologic evaluation of factors controlling glucose
tolerance in man: measurement of insulin sensitivity
and beta-cell glucose sensitivity from the response to
intravenous glucose. Journal of Clinical Investigation,
68(6), 1456–1467.
Charitos, T., Van der Gaag, L.C., Visscher, S., Schurink,
K.A. & Lucas, P.J., 2009. A dynamic Bayesian
network for diagnosing ventilator-associated
pneumonia in ICU patients. Expert Systems with
Applications, 36(2P1), 1249–1258.
Chase J, Shaw G, Wong X, Lotz T, Lin J, Hann C., 2006.
Model-based glycaemic control in critical care—A
review of the state of the possible. Biomedical Signal
Processing and Control, 1(1), 3-21.
Van Gerven, M.A., Taal, B.G. & Lucas, P.J., 2008.
Dynamic Bayesian networks as prognostic models for
clinical patient management. Journal of Biomedical
Informatics, 41(4), 515-529.
Haverbeke N, Van Herpe T, Diehl M, Van den Berghe G,
De Moor B, 2008. Nonlinear model predictive control
with moving horizon state and disturbance estimation
– application to the normalization of blood glucose in
the critically ill. Proceedings of the 17th IFAC World
Congress 2008.
Hovorka, R., Chassin, L.J., Ellmerer, M., Plank, J. and
Wilinska, M.E.:,2008. A simulation model of glucose
regulation in the critically ill. Physiological
Measurement, 29, 959-978.
Iserles, Arieh, 2009. A first course in the numerical
analysis of differential equations. Second edition.
Cambridge Texts in Applied Mathematics. Cambridge
University Press, Cambridge.
Lin, J., Lee, D., Chase, J., Shaw,G., Le Compte, A., Lotz,
T., Wong, J., Lonergan, T., Hann, C.,2008. Stochastic
modelling of insulin sensitivity and adaptive glycemic
control for critical care. Comput. Methods Prog.
Biomed., 89(2), 141-152.
Russell, S. & Norvig, P., 2002. Artificial Intelligence: A
Modern Approach (2nd Edition), Prentice Hall.
The NICE-SUGAR Study Investigators, Finfer S, Chittock
DR, Su SY, Blair D, Foster D, Dhingra V, Bellomo R,
BIOSIGNALS 2010 - International Conference on Bio-inspired Systems and Signal Processing
458