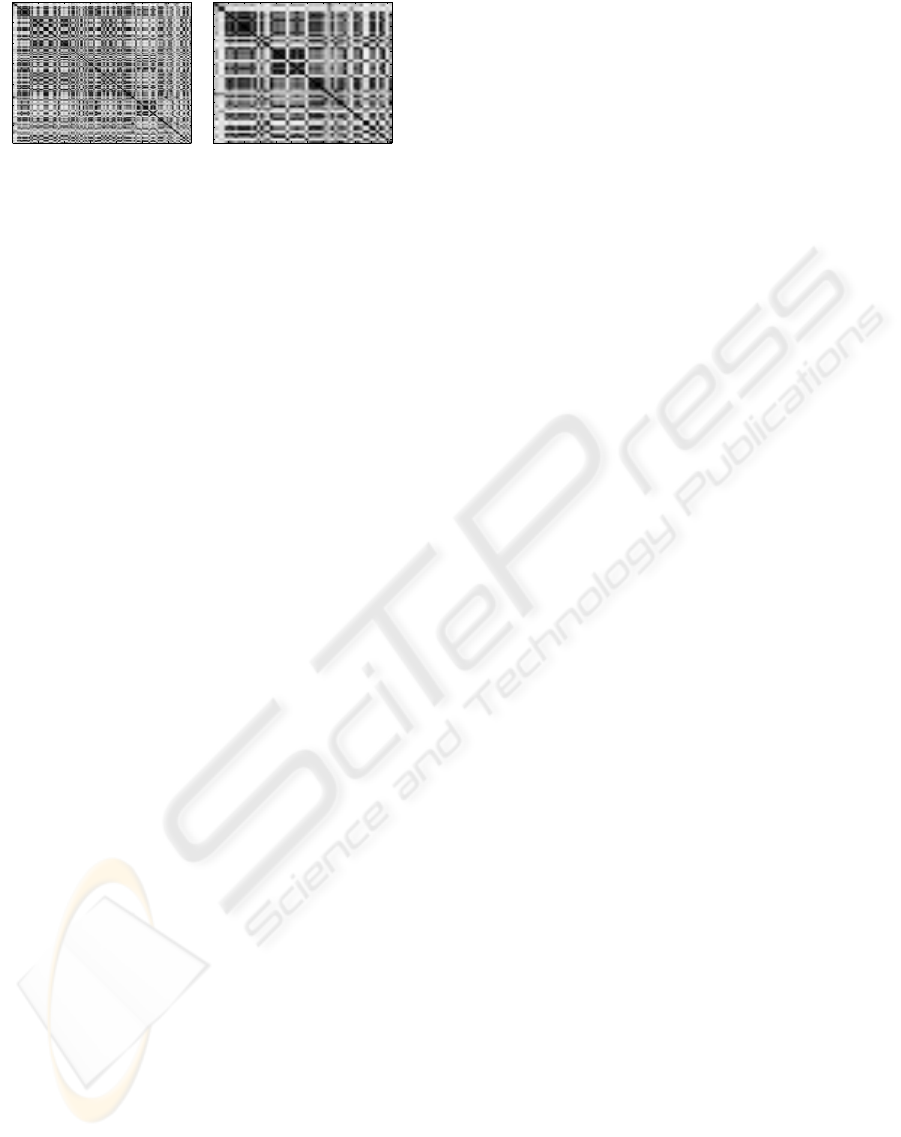
20 40 60 80 100 120
20
40
60
80
100
120
(a) Original data set.
5 10 15 20 25 30 35 40 45 50 55
5
10
15
20
25
30
35
40
45
50
55
(b) Denoised data set.
Figure 10: Subject 5 - Co-association matrices.
ces of one subject that exhibits no differentiated states
during the execution of the concentration task. In this
case, no states were detected for the original data set
or the denoised data set.
Overall, by visual inspection of co-association
matrices and clusters evolution associated to the orig-
inal data sets, it is possible to infer the existence of
emotional states with temporal evolution for 12 of the
24 subjects. After applying the proposed genetic al-
gorithm, these states are differentiated and in a few
cases completely separated for 8 of those 12 subjects,
and also for two other subjects for whose temporal se-
ries is not possible to obtain a state structure using just
the original data sets. For these 10 subjects, a maxi-
mum of 59% samples and a minimum of 31% are re-
moved in order to evidentiate the temporal separation
of the clusters.
The fact the number of subjects for whom differ-
entiated states are detected is greater for the original
data sets than for the subsets obtained with denois-
ing is related with the fitness function proposed for
evaluation of each possible subset. The criteria cho-
sen to implement it are strict, given that we want to
evidence completely separated states, using for each
evaluation different partitions obtained by each hier-
archical method. It is possible that one or more of
these methods, for instance Single Link, may be in-
adequate for the analysis of the temporal series, thus
degrading the fitness values
6 CONCLUSIONS
The proposed methodology of analysis is based on
unsupervised learning techniques, namely representa-
tive clustering algorithms and a state of the art cluster-
ing ensemble method, and also on a proposed genetic
algorithm for assessment of the existence of differen-
tiated states in temporal series of signals by denoising
of the data sets, as well as for validation of the re-
sults obtained for the original data sets. Results show
that the use of denoising techniques as a means of
pre-processing the data and validation of the state ob-
servations for the original data sets reinforces the as-
sumption that at least two different states are present
in the data set, each corresponding to a single cluster
or combination of clusters. Thus, it is possible to in-
fer the existence of emotional states in the temporal
series analyzed by employing unsupervised learning
methods and an evolutionary computation technique.
Ongoing work consists on a further extensive vali-
dation of the proposedmethodology in the herein pre-
sented application domain, as well as extrapolation to
the automatic analysis of other time series, such as
EEG data.
ACKNOWLEDGEMENTS
We acknowledge financial support from the FET pro-
gramme within the EU FP7, under the SIMBAD
project (contract 213250).
REFERENCES
Gamboa, H. (2008). Multi-Modal Behavioral Biomet-
rics Based on HCI and Electrophysiology. PhD
Thesis, Instituto Superior T´ecnico, Lisboa, Portugal.
http://www.lx.it.pt/ afred/pub/thesisHugoGamboa.pdf
Jain, A. K., Murty, M. N. and Flynn, P. J. (1999). Data
Clustering: A Review. In ACM Computing Surveys,
Vol.31, Issue 3, pp.264-323.
Fred, A. and Jain, A. K. (2005). Combining Multiple Clus-
terings Using Evidence Accumulation. In IEEE Trans.
on Pattern Analysis and Machine Intelligence, Vol.27,
Issue 6.
Xu, R. and Wunsch II, D. (2005). Survey of Clustering
Algorithms. In IEEE Trans. on Neural Networks, Vol.
16, Issue 3, pp.645-678.
Ng, A. Y., Jordan, M. I. and Weiss, Y. (2002). On Spectral
Clustering: Analysis and an Algorithm. In Advances
in Neural Information Processing Systems, volume
14, pp 849-856.
Shi, J. and Malik, J. (2000). Normalized Cuts and Image
Segmentation. In IEEE Trans. on Pattern Analysis and
Machine Intelligence, Vol. 22, Issue 8.
Lourenc¸o, A. and Fred, A. (2008). Unveiling Intrinsic Sim-
ilarity: Application to Temporal Analysis of ECG. In
Proc. of BIOSIGNALS 2008, pp. 104-109.
Sumathi, S., Hamsapriya, T. and Surekha, P. (2008). Evo-
lutionary Intelligence: An Introduction to Theory and
Applications with Matlab,Springer.
ICAART 2010 - 2nd International Conference on Agents and Artificial Intelligence
142