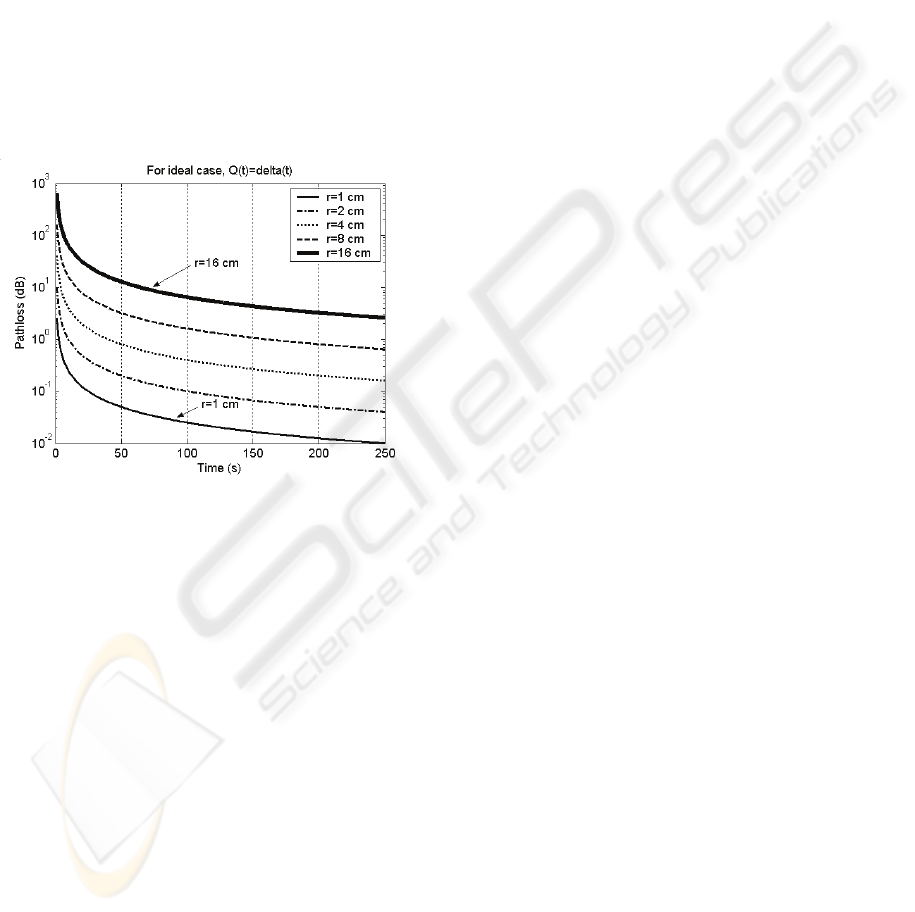
from pathloss. This is how it is shown in the pathloss
equation above that the pathloss is a function of both
distance r and time t and both of these two variables
have to be handled simultaneously. This makes the
pathloss in molecular communication a bit
complicated by not being able to express it as a
function of distance only. The pathloss for different
distances as a function of time are shown in Fig. 9.
Initially there is a high pathloss because when the
TN starts transmitting the molecules, there is no
molecules available at the RN side as being a slow
process it takes some time for the molecules to
propagate from TN to RN. After a long time
transmitted molecules reach the intended RN and so
the pathloss decreases with time. This indicates the t
in the denominator in the power of exp(r
2
/4Dt) in
eqn. (6).
Figure 9: Pathloss as a function of time t for different
distances r from TN.
3 CONCLUSIONS
In this paper we have developed an analytical
approach for getting the channel quantum response
(CQR) or equivalently the throughput response for
molecular communication. This analysis contributes
to the recent research of molecular propagation
channel modeling and subsequent analyses. An
analytical approach is useful in the sense that real
propagation of molecular communication is very
difficult due to extremely small (nano) scale of
dimensions and experimental requirements. In such
cases if a molecular propagation channel could be
characterized analytically then the results would
become very handy to analyze such a propagation
channel without actually waiting for analyses with
real molecular data and in vitro experiments. The
approach presented in this paper is based on the
spatial and temporal distribution of received
concentration of the information molecules in a
given propagation medium. Two things to be noted
regarding the diffusion coefficient parameter D,
firstly, it is assumed that the diffusion coefficient D
remains constant during the period of analysis. This
is validated by several open literature in this area.
Also it is to be noted that propagation in air is
considered (D=0.43) in this paper. However, similar
results in aqueous medium e.g. water, blood plasma
can also be obtained. Please note that different
values of the diffusion coefficient D of the
propagation media characterize differently the
Brownian motion of information molecules in
different media. As a second thought, the effects of
the information molecules themselves on the
propagation are not considered for now but are left
as the on-going part of our current research.
Statistical analyses of the results obtained in this
paper are also one of our recent research works in
this area.
REFERENCES
Akyildiz, I. F., Brunetti, F., Blazquez, C., 2008.
NanoNetworking: A New Communication Paradigm.
Computer Networks Journal (Elsevier), Vol. 52, pp.
2260-2279, August, 2008.
Atakan, B., Akan, O. B., 2007. An Information
Theoretical Approach for Molecular Communication.
In IEEE/ACM Bionetics, Budapest, Hungary,
December.
Berg, H. C., 1993. Random Walks in Biology, Princeton
University Press.
Bossert, W. H., 1963. Analysis of Olfactory
Communication among Animals. Journal of
Theoretical Biology, pp.443-469.
Lacasa, N. R., 2009. Modeling the Molecular
Communication Nanonetworks. M.Sc. thesis, The
Universitat Politècnica de Catalunya (UPC), Spain,
January.
Moritani, Y., Hiyama, S., Suda, T., 2006. Molecular
Communication for Health Care Applications. In
Fourth IEEE International Conference on Pervasive
Computing and Communications Workshops, pp. 549-
553, March.
Moore, M. J., Suda, T., Oiwa, K., 2009. Molecular
Communication: Modeling Noise Effects on
Information Rate. IEEE Trans. on Nanobioscience,
vol. 8, no. 2, pp. 169-180, June.
Parcerisa, L., Akyildiz, I. F., 2009. Molecular
Communication Options for Long Range
Nanonetworks. to appear in Communication Networks
(Elsevier) Journal, UK.
BIOSIGNALS 2010 - International Conference on Bio-inspired Systems and Signal Processing
332